the Creative Commons Attribution 4.0 License.
the Creative Commons Attribution 4.0 License.
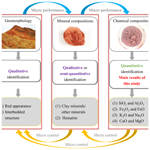
Basic chemical composition combination rules and quantitative criterion of red beds
Guangjun Cui
Jin Liao
Linghua Kong
Cuiying Zhou
Zhen Liu
Lei Yu
Lihai Zhang
Red beds belong to slippery formations, and their rapid identification is of great significance for major scientific and engineering issues, such as geological hazard risk assessment and rapid response to geological disasters. Existing research often identifies red beds from a qualitative or semiquantitative perspective, resulting in slow recognition speed and inaccurate recognition results, making it difficult to quickly handle landslide geological disasters. Combined with the correlation between red beds' geomorphic characteristics, mineral compositions, and chemical compositions, this study established a preliminary identification quantitative criterion based on the basic chemical composition combination rules (SiO2+Al2O3, , FeO+Fe2O3, , K2O+Na2O, , CaO+MgO, and ) in the red beds. Following this, we perform principal component analysis on the basic chemical composition combination rules mentioned above. The results indicate that simultaneously meeting the following principal component features can serve as a rapid quantitative criterion for distinguishing red beds from other rocks: –23.55; –3.11; –4.88; –4.52; –7.30; and –1.89. By comparing the chemical composition combinations of 15 kinds of rocks collected from China in this study, it is proven that the quantitative criterion proposed in this study is effective. The study results can be used for rapid identification of red beds, achieving risk assessment and rapid response to geological disasters such as landslides.
- Article
(6914 KB) - Full-text XML
-
Supplement
(32292 KB) - BibTeX
- EndNote
Red beds are widely distributed throughout the world (Zhou et al., 2023b; Yan et al., 2019; Chen et al., 2021). Geological disasters occur frequently in the red bed distribution area, especially landslides, debris flows, collapses, and underground engineering damage (Chen et al., 2014; Zhou et al., 2023a; Y. Wang et al., 2022). According to the characteristics of disasters such as landslides, the red beds belong to “landslide prone strata”, and the instability of slopes with weak interlayers of the red beds is particularly evident (Zhang et al., 2015). This is mainly due to the strong hydrophilicity and weak permeability of the red beds, which are prone to softening and plastic deformation under the action of water. After absorbing water, the red beds easily expand, and after losing water, they are easily contract. The weathering resistance of the red beds is weak, they easily collapse, and their compressive and shear strengths are low (Zhang et al., 2016, 2024; Wu et al., 2018; Wang et al., 2017; Marat et al., 2022). The red beds have different lithology or poor binding force with other rock strata, which can easily cause differential deformation and lead to mass rock sliding along the bedding plane (Liu et al., 2020; He et al., 2023; Wang et al., 2024). Therefore, the identification of rock types, especially the rapid determination of red beds, is of great significance for major scientific and engineering issues, such as risk assessment and rapid response to geological disasters, in the red bed distribution area.
At present, the studies on red bed identification are mostly carried out from the perspectives of geomorphic characteristics, mineral compositions, and chemical compositions (Cui et al., 2022; Zhou et al., 2021). There is a close relationship between these perspectives (Moonjun et al., 2017; Bankole et al., 2016; Perri et al., 2013). For example, the content of Fe2O3 or hematite in the red beds is higher than that in the gray beds (Hu et al., 2006). Among these perspectives, the research into geomorphic characteristics and mineral compositions mostly adopts qualitative or semiquantitative methods, and there are many such studies. For example, Rainoldi et al. (2015) identified red beds by studying the color of geomorphic characteristics and hematite in mineral compositions and the mechanism of red bed bleaching. Uchida et al. (2000) distinguished red sandstone, yellowish brown sandstone, and green sandstone according to the content of hematite, goethite, biotite, and muscovite in the mineral compositions; analyzed the characteristics of different rocks; and pointedly protected Angkor monuments. Xue et al. (2023) distinguished red mudstone and red sandstone by quantifying the clay mineral content in the mineral compositions in order to analyze the mechanisms and control factors of summer uplift of high-speed railway cutting. At this stage, the research on the geomorphology, mineral color, and clay content of the red beds lays the foundation for the identification of the red beds, but this identification is still vague and needs to be further quantified. Therefore, some scholars have conducted quantitative studies on the chemical compositions of red beds. Hong et al. (2009) analyzed the alteration of clay minerals by studying the changes in the ratio in the chemical compositions of the red beds, thereby obtaining the weathering degree of the red beds. Bankole et al. (2016) studied the relationship between the ratio, ratio, and ratio of red beds to indirectly study the oxygen content of the Paleoproterozoic. Hu et al. (2006) studied the characteristics of high Fe2O3 content and low FeO content in the oceanic red beds and analyzed ancient landslides on the continental margin from the perspective of petrology. However, these studies do not distinguish between red beds and other rocks in terms of chemical compositions. The use of portable spectrometers and drone-borne multi-sensor remote sensing techniques can quickly obtain the chemical compositions of rocks in geological disasters while ensuring safety (Triantafyllou et al., 2021; Kirsch et al., 2018), making it feasible to use chemical compositions as the standards to distinguish red beds from other rocks.
Therefore, the purpose of this study to develop a quantitative criterion for quickly and accurately identifying the red beds. This study collected the data about the geomorphic characteristics, mineral content, and chemical composition of red beds and other rocks; compared these data to obtain the basic characteristics of red beds; and finally summarized and analyzed the red bed identification criterion and verified the reliability of this criterion.
Figure 1 shows the methodology used in this study, involving the investigation of geomorphic characteristics, mineral compositions, and chemical compositions (the perspective of chemical compositions is the focus of this study). In this study, data on geomorphological features, mineral content and chemical composition of the red beds and other rocks were first collected, then these data were compared to derive the basic characteristics of the red beds, the red bed identification criteria were summarized and analyzed, and the reliability of the criteria was verified.
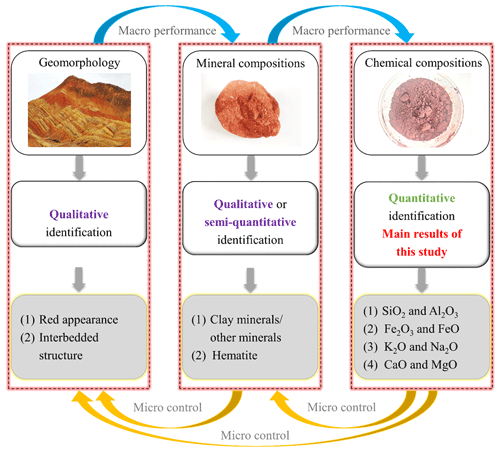
Figure 1Methodology for identifying red beds from geomorphic characteristics, mineral compositions, and chemical compositions.
2.1 Data collection
The geomorphic characteristics data were collected from the previous studies about landslides, debris flows, and collapses of red beds, igneous rocks (andesite, basalt, diorite, granite), metamorphic rocks (gneiss, marble), and other sedimentary rocks (arkose, black shale, breccia, claystone, dolomite, lignite, limestone, marl, mudstone, siliciclastic rock, tuff) (e.g., Zhang et al., 2015, 2017; San et al., 2020; He et al., 2021; Ciftci et al., 2008; Perez-Rey et al., 2019; Anbarasu et al., 2010; Xia et al., 2019; Gokbulak and Ozcan, 2008; Li et al., 2016; F. W. Wang et al., 2022; Underwood et al., 2016; Kavvadas et al., 2020; Harp et al., 2011; De Montety et al., 2007; Contino et al., 2017; Liu et al., 2018; Ni et al., 2015; Hale et al., 2021). The geomorphic characteristics of red beds investigated in this study involve the evolution process and distribution of red beds on Earth's surface, and the results were compared with that of other types of rock samples.
The mineral compositions of red beds (1536 datasets) were collected from the previous studies shown in Table 1 in the Supplement (Jian et al., 2009; Liu et al., 2020; Zha et al., 2022; Bai et al., 2020; Zhang et al., 2021, 2020, 2016; Yao et al., 2016; Li et al., 2023, 2015, 2013; Marat et al., 2022; Wang et al., 2017, 2018, 2014; Chen et al., 2014). These studies used semiquantitative or quantitative methods involving X-ray diffraction (XRD) technology to statistically analyze the differences in mineral composition between different red beds (e.g., quartz, feldspar, mica, hematite, clay minerals, and calcite), as detailed in the aforementioned literature. This study mainly focuses on the influence of mineral compositions on geomorphic characteristics, particularly the layered structure and color of red beds.
The chemical compositions of red beds (1536 datasets) with different geological ages and various lithologies, such as conglomerate, sandy conglomerate, sandstone, siltstone, shale and mudstone, were collected from the previous studies as shown in Table 2 in the Supplement (e.g., Uchida et al., 2000; Xue et al., 2023; Jiang et al., 2022; Yang et al., 2016; Liu et al., 2020, 2006, 2007; Kong et al., 2018; Zhao et al., 2005; Gao et al., 2017; Zhang et al., 2008; Zhu et al., 2003; Hong et al., 2009; Wild et al., 2017). We searched for the chemical compositions of igneous rocks, including andesite (Table 3 in the Supplement, 49 203 datasets; data were downloaded from the GEOROC database (https://georoc.mpch-mainz.gwdg.de//georoc/) on 11 May 2023, identified by using “andesite” as the search parameter), basalt (Table 4 in the Supplement, 80 365 datasets; data were downloaded from the GEOROC database on 11 May 2023, identified by using “basalt” as the search parameter), diorite (Table 5 in the Supplement, 4941 datasets; data were downloaded from the GEOROC database on 11 May 2023, identified by using “diorite” as the search parameter), and granite (Table 6 in the Supplement, 17 272 datasets; data were downloaded from the GEOROC database on 11 May 2023, identified by using “granite” as the search parameter). We also searched for the chemical compositions of metamorphic rocks, including gneiss (Table 7 in the Supplement, 24 300 datasets; data were downloaded from the EarthChem Portal Database (http://portal.earthchem.org/) on 20 April 2018, identified by using “metamorphic” under material and “gneiss” under rock name as search parameters) and marble (Table 8 in the Supplement, 3364 datasets; data were downloaded from the EarthChem Portal Database on 12 May 2023, identified by using “metamorphic” under material and “marble” under rock name as search parameters). Finally, we searched for the chemical compositions of other sedimentary rocks, including arkose (Table 9 in the Supplement, 682 datasets; data were downloaded from the EarthChem Portal Database on 10 May 2023, identified by using “sedimentary” under material and “arkose” under rock name as search parameters), black shale (Table 10 in the Supplement, 305 datasets; data were downloaded from the EarthChem Portal Database on 10 May 2023, identified by using “sedimentary” under material and “black shale” under rock name as search parameters), breccia (Table 11 in the Supplement, 1396 datasets; data were downloaded from the EarthChem Portal Database on 10 May 2023, identified by using “sedimentary” under material and “breccia” under rock name as search parameters), claystone (Table 12 in the Supplement, 3790 datasets; data were downloaded from the EarthChem Portal Database on 10 May 2023, identified by using “sedimentary” under material and “claystone” under rock name as search parameters), dolomite (Table 13 in the Supplement, 2169 datasets; data were downloaded from the EarthChem Portal Database on 6 May 2023, identified by using “sedimentary” under material and “dolomite” under rock name as search parameters), lignite (Table 14 in the Supplement, three datasets; data were downloaded from the EarthChem Portal Database on 24 April 2018, identified by using “sedimentary” under material and “lignite” under rock name as search parameters), limestone (Table 15 in the Supplement, 9104 datasets; data were downloaded from the EarthChem Portal Database on 10 May 2023, identified by using “sedimentary” under material and “limestone” under rock name as search parameters), marl (Table 16 in the Supplement, 142 datasets; data were downloaded from the EarthChem Portal Database on 10 May 2023, identified by using “sedimentary” under material and “marlstone, marl” under rock name as search parameters), mudstone (Table 17 in the Supplement, 6140 datasets; data were downloaded from the EarthChem Portal Database on 10 May 2023, identified by using “sedimentary” under material and “mudstone, mud” under rock name), siliciclastic rock (Table 18 in the Supplement, 26 938 datasets; data were downloaded from the EarthChem Portal Database on 10 May 2023, identified by using “sedimentary” under material and “siliciclastic” under rock name as search parameters), and tuff (Table 19 in the Supplement, 10 295 datasets; data were downloaded from the EarthChem Portal Database on 6 May 2023, identified by using “sedimentary” under material and “tuff” under rock name as search parameters).
Studies have found that rock disasters are related to the content of minerals, such as quartz, clay minerals, hematite, calcite, dolomite, and feldspar, and that these mineral contents are also closely related to the combination of major elements or oxides (Table 1), for example, SiO2 and Al2O3 (used to study the relative content relationship between quartz and clay minerals) (Hong et al., 2009), Fe2O3 and FeO (used to study the high hematite content characteristics of hematite) (Hu et al., 2006), CaO and MgO (used to study the content relationship of potassium feldspar, calcite, and dolomite) (Han et al., 2023), and Na2O and K2O (Qiao et al., 2017). Therefore, this study on the basic chemical composition combination rules and the quantitative criterion of the red beds only involves the major elements mentioned above and does not involve the analysis of trace elements or other stable isotopes.
Table 1Chemical composition (%) of minerals in red beds from our chosen database.
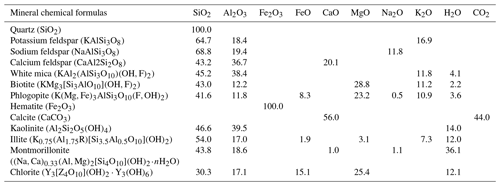
Note that all data were collected from http://webmineral.com/ (last access: 31 October 2023) and https://www.mindat.org/ (last access: 31 October 2023).
Using the SPSS PRO online data analysis program and principal component analysis method to compare the chemical component combination rules of red beds, the quantitative identification criterion was studied at a significance level of P<0.05.
2.2 Criterion verification
In order to verify the proposed basic chemical composition combination rules and quantitative criterion of red beds, 15 kinds of rocks of known rock types were selected in Guangdong, Sichuan, Hubei, Zhejiang, and Anhui provinces (Fig. 2), including 12 kinds of red beds (red claystone, red mudstone, red silty mudstone, red argillaceous siltstone, red fine sandstone, red medium sandstone, red coarse sandstone, red conglomerate), limestone (one kind), arkose (one kind), and mudstone (one kind). After on-site sampling, we used a hammer to smash the rock block out of the fresh surface. Following this, the fresh surface was analyzed using the YL-P-3LRX handheld laser-induced breakdown spectroscopy (LIBS, Fig. 3) instrument to check whether these elements conform to the basic chemical composition combination rules of red beds proposed by this study. This device can detect elements such as K, Na, Si, Al, Ca, Mg, Fe, and oxides.
The working principle of the LIBS is that a miniature X-ray source provides tube voltage and tube current, and the light tube emits continuous X-ray spectral lines. The X-rays irradiated on the sample knock out the inner electrons of the K and L layers of the element atoms, and the holes in the low-energy layer are filled by high-energy outer electrons (N layer). The high-energy electrons emit excess energy as X-ray fluorescence (Kα) with elemental characteristics. Thus, the instrument detects the type and concentration of elements through the emitted spectral lines. On the instrument analysis interface, point the detection window toward the rock sample and press the trigger to start and stop the measurement. After amplification and data collection, the signal is processed to obtain the required test data.
3.1 Geomorphic characteristics of red beds
Geomorphic characteristics of the red beds as shown in Fig. 4. Red beds are sedimentary rocks of different geological ages (mainly Mesozoic and Cenozoic) with bedding structure typically consisting of various lithologies such as conglomerate, sandy conglomerate, sandstone, siltstone, shale, and mudstone that are predominantly red in color due to the presence of ferric oxides (Yan et al., 2019). Owing to differences in depositional environments and influences of late-stage geologic processes, the color of red beds can be brownish to reddish yellow, brownish yellow, purplish red, brownish red, grayish purple, and other reddish tints (Yan et al., 2019; Nance, 2015), making it difficult to accurately describe using the CIELAB color space and/or Munsell color system. Bedding is a common structural feature of sedimentary rocks representing the changes in the sedimentary environment. Sandstone is one of the most common types of red beds, with a distinct reddish appearance. Compared with the obvious layering and red appearance characteristics of red beds, igneous rocks and metamorphic rocks do not show the two characteristics of red appearance and bedding at the same time. Basalts are reddish in appearance but do not have bedding (Cunha et al., 2005). In addition, andesites are mainly light black and have a columnar structure that is similar to that of basalts (Feizizadeh et al., 2021). Most of granites are gray or light brown with a significantly different structure compared to red beds (Migon et al., 2018), while gneisses are generally characterized as a dark and light gneissic structure (Garajeh et al., 2022). Although the red appearance and bedding structure can be used as qualitative criteria for identifying the red beds, the analysis of mineral and chemical compositions is still necessary for identifying the rocks from quantitative perspective.
3.2 Mineral compositions of red beds
Table 2 shows the statistical analysis results of mineral compositions of red beds in Table 1 in the Supplement. The common minerals in the red bed are quartz (median value is 40 %, the same as below), clay minerals (35 %, including kaolinite, illite, montmorillonite, and chlorite), feldspar (10 %, including K-feldspar and plagioclase), calcite (10 %), mica (7 %, including biotite, muscovite, and sericite), and hematite (3 %) according to their content. According to the average value and standard deviation, it can be seen that the content range of various minerals has significant dispersion. The ratio of the content of clay minerals to other minerals (quartz, feldspar, mica, hematite, and calcite) ranges between 0.11 and 1.50. The hematite content ranges between 1.5 % and 10.0 % (percentile=10th–90th), and reddish appearance of red beds is due to the abundant hematite content of the rocks. The change in mineral compositions of red beds could lead to the change in rock color, which is one of the major characteristics of red beds. Furthermore, when the red beds encounter water, softening and expansion could happen because of the large amount of clay minerals in the rocks, especially for mudstone. The differences in mineral compositions of the red beds can also be quantitatively described through their chemical composition combination characteristics (Table 1).
3.3 Chemical composition characteristics of red beds
Figures 5 and 6 are mainly used to qualitatively analyze the differences in chemical compositions between the red beds and other rocks through scatter plots. The area surrounded by dashed black lines is the area where the red bed data points are located. To better distinguish various rock data points, the distribution areas of various rock data are shown on the right side of the figure, and the corresponding colored dashed ellipses are used to indicate the distribution areas in the dataset. Figure 5 shows the comparison of SiO2 and Al2O3, FeO and Fe2O3, K2O and Na2O, and CaO and MgO contents in red beds, igneous rocks, and metamorphic rocks. Figure 6 shows the comparison of SiO2 and Al2O3, FeO and Fe2O3, K2O and Na2O, and CaO and MgO contents in red beds and other sedimentary rocks.
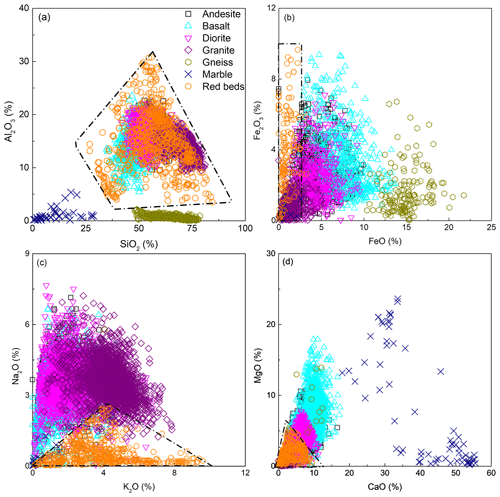
Figure 5Comparison of (a) SiO2 and Al2O3, (b) FeO and Fe2O3, (c) K2O and Na2O, and (d) CaO and MgO contents in red beds, igneous rock, and metamorphic rocks (icons of the same color in the figure have the same meaning).
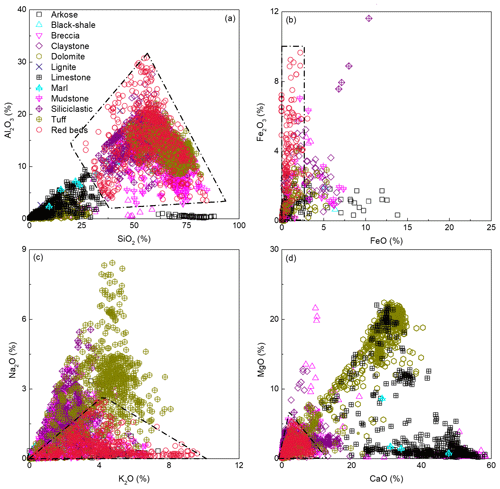
Figure 6Comparison of (a) SiO2 and Al2O3, (b) FeO and Fe2O3, (c) K2O and Na2O, and (d) CaO and MgO contents in red beds and other sedimentary rocks (icons of the same color in the figure have the same meanings).
The content of SiO2 in the red beds is about 30 %–80 %, Al2O3 is about 8 %–30 %, Fe2O3 is about 0 %–10 %, FeO is about 0 %–3 %, K2O is about 0 %–10 %, Na2O is about 0 %–2.5 %, CaO is about 0 %–10 %, and MgO is about 0 %–5 %. Compared with igneous rocks, metamorphic rocks, and other sedimentary rocks, the content of each chemical composition of the red beds has three relationships with the content of corresponding chemical composition of other rocks: inclusion relationship (the data distribution range of one rock completely covers and is larger than the data range of the other rock), intersection relationship (the data distribution range of one rock intersects with the data distribution range of another rock), and mutual difference relationship (the data distribution range of one rock does not intersect at all with the data distribution range of another rock). The distribution range of SiO2 and Al2O3 content in the red beds includes the distribution range of SiO2 and Al2O3 content in nine types of rocks, namely andesite, basalt, diorite, granite, black shale, claystone, mudstone, siliciclastic rock, and tuff. The distribution range of SiO2 and Al2O3 content in the red beds intersects with that in breccia, lignite, and marl. The distribution range of SiO2 and Al2O3 content in gneiss, marble, arkose, dolomite, and limestone is different from that in the red beds. The distribution range of Fe2O3 and FeO content in the red beds includes the distribution range of Fe2O3 and FeO content in granite, marble, and lignite. The distribution range of Fe2O3 and FeO content in the red beds intersects with that in eight kinds of rocks, namely andesite, basalt, diorite, breccia, claystone, dolomite, limestone, and mudstone. The distribution range of Fe2O3 and FeO content in gneiss, arkose, black shale, siliciclastic rock, and tuff is different from that in the red beds. The distribution range of K2O and Na2O content in the red beds includes the distribution range of K2O and Na2O content in lignite. The distribution range of K2O and Na2O content in the red beds intersects with that in 15 kinds of rocks, namely andesite, basalt, diorite, granite, marble, arkose, black shale, breccia, claystone, dolomite, limestone, marl, mudstone, siliciclastic rock, and tuff. The distribution range of K2O and Na2O content in gneiss is different from that in the red beds. The distribution range of CaO and MgO content in the red beds includes the distribution range of CaO and MgO content in granite, black shale, and lignite. The distribution range of CaO and MgO content in the red beds intersects with that in 13 types of rocks, including andesite, basalt, diorite, gneiss, arkose, breccia, claystone, dolomite, limestone, marl, mudstone, siliciclastic, and tuff. The distribution range of CaO and MgO content in marble is different from that in the red beds. Therefore, from a qualitative perspective, it can be seen that the red beds differ in chemical composition from 8 kinds of rocks, namely gneiss, marble, arkose, dolomite, limestone, black shale, siliciclastic rock, and tuff, and also intersects with other rocks to varying degrees. However, this is not enough as a criterion to determine the difference between red beds and other rocks.
Figures 7 and 8 mainly analyze the differences in chemical compositions between red beds and other rocks through further data statistics and box plots of the scatter plots mentioned above and propose a quantitative identification criterion for the red beds chemical composition combination. The dashed red box in the figures represents rocks that differ from the red bed data, while the dashed black box represents rocks that intersect less than 25 % with the red bed data. The data collected in Sect. 2.1 comes from published papers or databases, and its accuracy and robustness have been explained in relevant literature. In order to ensure the exclusion of outliers in the box plots mentioned above during the analysis of this study. The horizontal gray dashes corresponding to the red bed box chart represent the 10th percentile (the same below), lower quartile (25th percentile), median (50th percentile), upper quartile (75th percentile), and 90th percentile in the red bed data from bottom to top. Figure 7 shows the chemical compositions combination comparison of SiO2+Al2O3 (total content, the same as below) and (content ratio, the same as below), FeO+Fe2O3 and , K2O+Na2O and , and CaO+MgO and in red beds, igneous rock, and metamorphic rocks. Figure 8 shows the chemical composition combination comparisons of SiO2+Al2O3 and , FeO+Fe2O3 and , K2O+Na2O and , and CaO+MgO and in red beds and other sedimentary rocks.
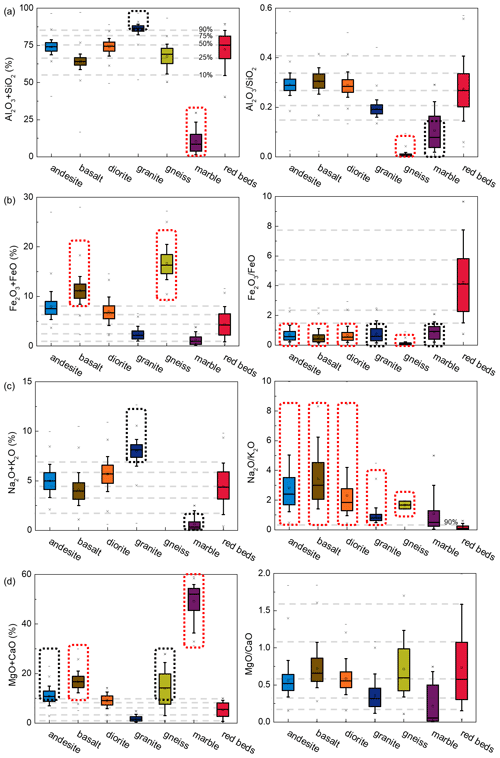
Figure 7Chemical composition comparisons of (a) SiO2+Al2O3 and , (b) FeO+Fe2O3 and , (c) K2O+Na2O and , and (d) CaO+MgO and in red beds, igneous rock, and metamorphic rocks.
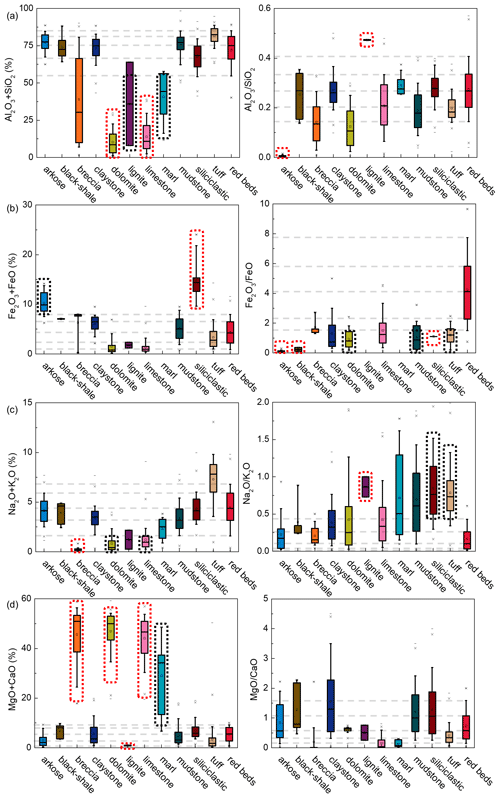
Figure 8Chemical composition comparisons of (a) SiO2+Al2O3 and , (b) FeO+Fe2O3 and , (c) K2O+Na2O and , and (d) CaO+MgO and in red beds and other sedimentary rocks.
The SiO2+Al2O3 content in the red beds is 54.7 %–85.0 % (10th–90th percentile, the same as below), the ratio is 0.14–0.41, the FeO+Fe2O3 content is 0.9 %–7.9 %, the ratio is 1.52–7.70, the K2O+Na2O content is 1.6 %–6.8 %, the ratio is 0.02–0.43, the CaO+MgO content is 0.8 %–9.2 %, and the ratio is 0.16–1.57. By comparing the content of SiO2+Al2O3, the red beds are distinct or have small intersections (less than 25 %, the same below) with granite, marble, dolomite, lignite, limestone, and marl. By comparing the ratio, it is found that the red beds are distinct or have small intersections with gneiss, marble, arkose, and lignite. By comparing the content of FeO+Fe2O3, it is found that the red beds are distinct or have small intersections with basalt, gneiss, arkose, and siliciclastic rock. By comparing the ratio, it is found that the red beds are distinct or have small intersections with andesite, basalt, diorite, granite, gneiss, marble, arkose, black shale, dolomite, mudstone, siliciclastic rock, and tuff. Through the comparison of K2O+Na2O content, the red beds are distinct or have small intersections with granite, marble, breccia, dolomite, and limestone. By comparing the ratio, the red beds are distinct or have small intersections with andesite, basalt, diorite, gneiss, lignite, siliciclastic rock, and tuff. Through the comparison of CaO+MgO content, the red beds are distinct or have small intersections with andesite, basalt, gneiss, marble, breccia, dolomite, limestone, and marl. By comparing the ratio, it is difficult to distinguish the red beds from other rocks.
In summary, there are differences in chemical compositions between red beds and other rocks. Simultaneously meeting the following chemical composition combinations as a preliminary quantitative criterion is required to distinguish red beds with different geological ages and various lithologies from other rocks: %–85.0 %; –0.41; %–7.9 %,; –7.70; %–6.8 %; –0.43; %–9.2 %; and –1.08.
3.4 Principal component analysis and quantitative criterion for red bed identification
Based on the preliminary quantitative criterion for identifying the red beds mentioned above, this section presents principal component analysis (PCA) statistical analysis (dimensionality reduction) of the SiO2+Al2O3, , FeO+Fe2O3, , K2O+Na2O, , CaO+MgO, and of red beds in Figs. 7 and 8. The result is significant with P<0.05 (Table 3), rejecting the null hypothesis. There is correlation between the variables, and principal component analysis is effective. It can be seen that the cumulative variance interpretation rate of the first five principal components reaches 94.788 % (generally greater than 90 % is sufficient), indicating that using the first five principal components is a good approach for red bed recognition.
According to the component matrix (Table 4) obtained during the PCA analysis process, the calculation equations for five principal components F1–F5 (Eqs. 1–5) and the calculation formula for the overall principal components F (Eq. 6) can be obtained.
Substituting the relevant data of the red beds in Figs. 7 and 8 into Eqs. (1)–(6) can calculate the quantitative criterion for the red beds: –23.55; –3.11; –4.88; –4.52; –7.30; and –1.89.
Table 5Chemical composition combinations of 15 kinds of rocks. Bold font indicates that the chemical composition of the red beds exceeds the quantification criterion. Italic font indicates that the chemical composition of the three rocks that do not belong to red beds exceeds the quantification criterion.
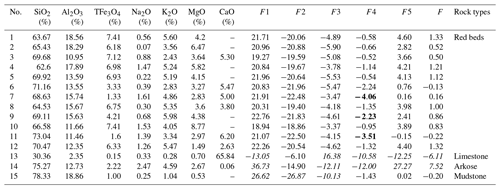
Note that TFe3O4 represents the content of Fe2O3 and FeO. “–” indicates that no content was detected. When Eqs. (1)–(5) cannot take values for Fe2O and , they can be set to 0.
3.5 Red bed identification quantification criterion verification
The chemical composition combinations of the 15 selected rocks in this study are shown in Table 5. Previous studies have found that the rapid detection of Fe2+ and Fe3+ is very difficult (Chen et al., 2019) and exceeds the detection range of the handheld laser-induced breakdown spectroscopy detailed in this paper and similar devices. However, this factor does not affect the reliability of the quantification criterion for red bed recognition. F1–F5 and F are considered as six evaluation indicators, and there are a total of 72 (6×12) evaluation indicators for the 12 types of red beds. Among them, three evaluation indicators exceed the scope of the quantification criterion for red bed identification (bold font in Table 5 indicates that the chemical composition of the red beds exceeds the quantification criterion), indicating that the reliability of detecting these 12 types of rocks belonging to the red beds is as high as 95.8 %. For three rocks that do not belong to red beds (limestone, arkose, and mudstone), there are a total of 18 evaluation indicators, of which 13 exceed the scope of the quantification criterion for red bed identification (indicated by italics in Table 5), indicating a high reliability of 72.2 % in detecting these three types of rocks that do not belong to the red beds. Therefore, this study proposes a quantitative criterion for red bed recognition with high reliability. In the future, if there are new devices that can quickly detect Fe2+ and Fe3+, the recognition efficiency of the red bed recognition quantification criterion in this study will be higher.
3.6 Application methods of our research results
Figure 9 shows the application methods of the research results. According to the methods for emergency management of landslide geological disasters (Fu et al., 2021), landslide risk assessment (including risk identification, risk analysis, and risk assessment) and risk management (developing and selecting treatment plans, as well as planning, implementing, and evaluating treatment methods) need to be carried out before the landslide occurs. In the field of engineering geology, risk identification is the most important prerequisite for landslide emergency response. Red bed are in the slippery layer that needs to be identified in risk identification.
At present, the commonly used risk identification method is to use drones to carry image capture devices for three-dimensional reconstruction of slope images, determine the volume of landslide accumulation, and determine the shape changes of the slope (Chen et al., 2020; Fu et al., 2021), which can be also used for mountain rescue (Wankmuller et al., 2021). Based on the drone technology, combined with the Optech Polaris LR 3D laser scanner and the HY-9070 hyperspectral analyzer of Sun Yat-sen University, the landslide shape change and remote monitoring of mineral and chemical compositions can be realized to identify whether it is a red bed landslide. It can also use a drone equipped with a block rock and soil sampling device to collect representative blocks of rock and soil within cracks to a safe area and then use the YL-P-3LRX Handheld laser-induced breakdown spectroscopy for rapid analysis. Therefore, the research results can be used for rapid identification of red beds, achieving risk assessment and rapid response to geological disasters such as landslides.
In response to the rapid identification of red beds in geological disaster emergency response, a rapid quantitative identification criterion based on the basic chemical compositions combination rules of red beds has been established, taking into account the correlation between red bed geomorphic characteristics, mineral composition, and chemical composition. It solves the current problem of fuzzy identification of the red beds.
The results indicate that the red beds in the geomorphic characteristics have obvious interlayer characteristics and that their appearance is red. In mineral composition, the ratio of clay minerals to other minerals of red beds ranges from 0.11 to 1.50, and the content of hematite of red beds ranges from 1.5 % to 10.0 %. The following chemical composition combinations can be used as a red bed preliminary quantification criterion: –85.0 %; –0.41; –7.9 %; –7.70; –6.8 %; –0.43; and –9.2 %. The principal component features can serve as a rapid quantitative criterion for distinguishing red beds: –23.55; –3.11; –4.88; –4.52; –7.30; and –1.89. The reliability of the quantitative criterion was verified by collecting 15 kinds of rocks and analyzing their chemical composition combinations.
The combination of research results with existing landslide geological hazard risk identification techniques can effectively carry out a rapid response to geological disasters, which is very important for emergency responses to geological disasters. Moreover, the research results can also be applied to the quantitative identification of red beds in other fields such as resources, ecology, environment, energy, and materials.
The data that support the findings of this study are available in the Supplement.
The supplement related to this article is available online at: https://doi.org/10.5194/se-15-1185-2024-supplement.
Conceptualization: CZ and ZL; methodology: GC and ZL; software: GC and LK; validation: GC, LK, and ZL; formal analysis: CZ and ZL; investigation: GC, JL, and LY; resources: GC and LK; data curation: GC, JL, LY and LK; writing – original draft preparation: GC and LK; writing – review and editing: GC, ZL, and LZ; visualization: LY; supervision: ZL and LZ; project administration: CZ; funding acquisition: CZ. All authors have read and agreed to the published version of the manuscript.
The contact author has declared that none of the authors has any competing interests.
Publisher's note: Copernicus Publications remains neutral with regard to jurisdictional claims made in the text, published maps, institutional affiliations, or any other geographical representation in this paper. While Copernicus Publications makes every effort to include appropriate place names, the final responsibility lies with the authors.
The authors would like to thank the anonymous reviewers for their very constructive and helpful comments.
This research has been supported by the National Natural Science Foundation of China (grant nos. 42293354, 42277131, 42293351, 42293355, and 42293350).
This paper was edited by Andrea Di Muro and reviewed by two anonymous referees.
Anbarasu, K., Sengupta, A., Gupta, S., and Sharma, S. P.: Mechanism of activation of the Lanta Khola landslide in Sikkim Himalayas, Landslides, 7, 135–147, https://doi.org/10.1007/s10346-009-0193-0, 2010.
Bai, Y., Shan, R., Ju, Y., Wu, Y., Tong, X., Han, T., and Dou, H.: Experimental study on the strength, deformation and crack evolution behaviour of red sandstone samples containing two ice-filled fissures under triaxial compression, Cold Reg. Sci. Technol., 174, 103061, https://doi.org/10.1016/j.coldregions.2020.103061, 2020.
Bankole, O. M., Albani, A. E., Meunier, A., Rouxel, O. J., Oisgauthier-Lafaye, F., and Bekker, A.: Origin of Red Beds in the Paleoproterozoic Franceville Basin, Gabon, and Implications for Sandstone-Hosted Uranium Mineralization, Am. J. Sci., 316, 839–872, https://doi.org/10.2475/09.2016.02, 2016.
Chen, J., Dai, F., Xu, L., Chen, S., Wang, P., Long, W., and Shen, N.: Properties and microstructure of a natural slip zone in loose deposits of red beds, southwestern China, Eng. Geol., 183, 53–64, https://doi.org/10.1016/j.enggeo.2014.10.004, 2014.
Chen, L. F., Tian, X. K., Xia, D. S., Nie, Y. L., Lu, L. Q., Yang, C., and Zhou, Z. X.: Novel Colorimetric Method for Simultaneous Detection and Identification of Multimetal Ions in Water: Sensitivity, Selectivity, and Recognition Mechanism, ACS Omega, 4, 5915–5922, https://doi.org/10.1021/acsomega.9b00312, 2019.
Chen, S. J., Xiang, C. C., Kang, Q., Zhong, W., Zhou, Y. L., and Liu, K.: Accurate landslide detection leveraging UAV-based aerial remote sensing, IET Commun., 14, 2434–2441, https://doi.org/10.1049/iet-com.2019.1115, 2020.
Chen, Z. Y., Männik, P., Fan, J. X., Wang, C. Y., Chen, Q., Sun, Z. Y., Chen, D. Y., and Li, C.: Age of the Silurian Lower Red Beds in South China: Stratigraphical Evidence from the Sanbaiti Section, J. Earth Sci.-China, 32, 524–533, https://doi.org/10.1007/s12583-020-1350-6, 2021.
Ciftci, E., Hogan, J. P., Kolayli, H., and Cadirli, E.: Natrolitite, an unusual rock – Occurrence and petrographic and geochemical characteristics (eastern Turkey), Clay. Clay Miner., 56, 207–221, https://doi.org/10.1346/Ccmn.2008.0560206, 2008.
Contino, A., Bova, P., Esposito, G., Giuffré, I., and Monteleone, S.: Historical analysis of rainfall-triggered rockfalls: the case study of the disaster of the ancient hydrothermal Sclafani Spa (Madonie Mts, northern-central Sicily, Italy) in 1851, Nat. Hazards Earth Syst. Sci., 17, 2229–2243, https://doi.org/10.5194/nhess-17-2229-2017, 2017.
Cui, G., Zhou, C., Liu, Z., Xia, C., and Zhang, L.: The synthesis of soft rocks based on physical and mechanical properties of red mudstone, Int. J. Rock Mech. Min., 151, 105037, https://doi.org/10.1016/j.ijrmms.2022.105037, 2022.
Cunha, P., Marques, J., Curi, N., Pereira, G. T., and Lepsch, I. F.: Geomorphic surfaces and latosol (oxisol) characteristics on a sandstone/basalt sequence from the Jaboticabal region, Sao Paulo State, Brazil, Rev. Bras. Cienc. Solo, 29, 81–90, https://doi.org/10.1590/S0100-06832005000100009, 2005.
de Montety, V., Marc, V., Emblanch, C., Malet, J. P., Bertrand, C., Maquaire, O., and Bogaard, T. A.: Identifying the origin of groundwater and flow processes in complex landslides affecting black marls: insights from a hydrochemical survey, Earth Surf. Proc. Land., 32, 32–48, https://doi.org/10.1002/esp.1370, 2007.
Feizizadeh, B., Garajeh, M. K., Blaschke, T., and Lakes, T.: An object based image analysis applied for volcanic and glacial landforms mapping in Sahand Mountain, Iran, Catena, 198, 105073, https://doi.org/10.1016/j.catena.2020.105073, 2021.
Fu, L., Zhu, J., Li, W.-L., You, J.-G., and Hua, Z.-Y.: Fast estimation method of volumes of landslide deposit by the 3D reconstruction of smartphone images, Landslides, 18, 3269–3278, https://doi.org/10.1007/s10346-021-01702-9, 2021.
Gao, F., Wu, X., and Deng, R.: The distribution of red beds and analysis on engineering characteristics of mudstone in Guangxi, Journal of Geological Hazards and Environment Preservation, 28, 48–52, 2017.
Garajeh, M. K., Feizizadeh, B., Blaschke, T., and Lakes, T.: Detecting and mapping karst landforms using object-based image analysis: Case study: Takht-Soleiman and Parava Mountains, Iran, The Egyptian Journal of Remote Sensing and Space Science, 25, 473–489, https://doi.org/10.1016/j.ejrs.2022.03.009, 2022.
Gokbulak, F. and Ozcan, M.: Hydro-physical properties of soils developed from different parent materials, Geoderma, 145, 376–380, https://doi.org/10.1016/j.geoderma.2008.04.006, 2008.
Hale, S., Ries, X., Jaeggi, D., and Blum, P.: Mechanical and hydraulic properties of the excavation damaged zone (EDZ) in the Opalinus Clay of the Mont Terri rock laboratory, Switzerland, Solid Earth, 12, 1581–1600, https://doi.org/10.5194/se-12-1581-2021, 2021.
Han, P. H., Zhang, C., Wang, X. J., and Wang, L.: Study of mechanical characteristics and damage mechanism of sandstone under long-term immersion, Eng. Geol., 315, 107020, https://doi.org/10.1016/j.enggeo.2023.107020, 2023.
Harp, E. L., Dart, R. L., and Reichenbach, P.: Rock fall simulation at Timpanogos Cave National Monument, American Fork Canyon, Utah, USA, Landslides, 8, 373–379, https://doi.org/10.1007/s10346-010-0251-7, 2011.
He, J., Niu, F., Luo, F., Jiang, H., He, P., and Ju, X.: Mechanical properties and modified binary-medium constitutive model for red-bed soft rock subjected to freeze-thaw cycles, Cold Reg. Sci. Technol., 209, 103803, https://doi.org/10.1016/j.coldregions.2023.103803, 2023.
He, K., Ma, G. T., and Hu, X. W.: Formation mechanisms and evolution model of the tectonic-related ancient giant basalt landslide in Yanyuan County, China, Nat. Hazards, 106, 2575–2597, https://doi.org/10.1007/s11069-021-04555-6, 2021.
Hong, H., Li, Z., and Xiao, P.: Clay Mineralogy Along the Laterite Profile in Hubei, South China: Mineral Evolution and Evidence for Eolian Origin, Clay. Clay Miner., 57, 602–615, https://doi.org/10.1346/Ccmn.2009.0570508, 2009.
Hu, X., Wang, C., Li, X., and Luba, J.: Upper Cretaceous oceanic red beds in southern Tibet: Lithofacies, environments and colour origin, Sci. China Ser. D, 49, 785–795, https://doi.org/10.1007/s11430-006-0785-7, 2006.
Jian, W. X., Wang, Z. J., and Yin, K. L.: Mechanism of the Anlesi landslide in the Three Gorges Reservoir, China, Eng. Geol., 108, 86–95, https://doi.org/10.1016/j.enggeo.2009.06.017, 2009.
Jiang, H., Xia, Y., Li, J., Liu, S., Zhang, M., and Wang, Y.: Controlling the Iron Migration Mechanism for the Cretaceous Sediment Color Variations in Sichuan Basin, China, ACS Omega, 7, 480–495, https://doi.org/10.1021/acsomega.1c04893, 2022.
Kavvadas, M., Roumpos, C., and Schilizzi, P.: Stability of Deep Excavation Slopes in Continuous Surface Lignite Mining Systems, Geotechnical and Geological Engineering, 38, 791–812, https://doi.org/10.1007/s10706-019-01066-x, 2020.
Kirsch, M., Lorenz, S., Zimmermann, R., Tusa, L., Mockel, R., Hodl, P., Booysen, R., Khodadadzadeh, M., and Gloaguen, R.: Integration of Terrestrial and Drone-Borne Hyperspectral and Photogrammetric Sensing Methods for Exploration Mapping and Mining Monitoring, Remote Sens.-Basel, 10, 1366, https://doi.org/10.3390/rs10091366, 2018.
Kong, L. W., Zeng, Z. X., Bai, W., and Wang, M.: Engineering geological properties of weathered swelling mudstones and their effects on the landslides occurrence in the Yanji section of the Jilin-Hunchun high-speed railway, B. Eng. Geol. Environ., 77, 1491–1503, https://doi.org/10.1007/s10064-017-1096-2, 2018.
Li, A., Deng, H., Zhang, H., Liu, H., and Jiang, M.: The shear-creep behavior of the weak interlayer mudstone in a red-bed soft rock in acidic environments and its modeling with an improved Burgers model, Mech. Time-Depend. Mat., 27, 1–18, https://doi.org/10.1007/s11043-021-09523-y, 2023.
Li, J., Xu, Q., Hu, Z., Liu, H., Zhang, Q., Lu, Y., and Wang, S.: Experimental research on softening of undisturbed saturated slip soil in eastern of Sichuan province red bed, Chinese Journal of Rock Mechanics and Engineering, 34, 4333–4342, 2015.
Li, S., Chen, J., and Yi, G.: Experimental study on the relationship between micro-characteristics and compressive strength of the red bed rock, Geotechnical Investigation and Surveying, 41, 1–5, 2013.
Li, X. N., Zhu, B. L., and Wu, X. Y.: Swelling characteristics of soils derived from black shales heightened by cations in Northern Chongqing, China, J. Mt. Sci.-Engl., 13, 1107–1119, https://doi.org/10.1007/s11629-015-3576-9, 2016.
Liu, C., He, C., and He, M.: Engineering geology study on failure of red beds slopes along railway in the west of Hunan Province, The Chinese Journal of Geological Hazard and Control, 18, 58–62, 2007.
Liu, J., Wei, J. H., Hu, H., Wu, J. M., Sun, S. R., and Kanungo, D. P.: Research on the engineering geological conditions and stability evaluation of the B2 talus slide at the Jin'an Bridge hydropower station, China, B. Eng. Geol. Environ., 77, 105–125, https://doi.org/10.1007/s10064-017-1005-8, 2018.
Liu, J., Xu, Q., Wang, S., Siva Subramanian, S., Wang, L., and Qi, X.: Formation and chemo-mechanical characteristics of weak clay interlayers between alternative mudstone and sandstone sequence of gently inclined landslides in Nanjiang, SW China, B. Eng. Geol. Environ., 79, 4701–4715, https://doi.org/10.1007/s10064-020-01859-y, 2020.
Liu, X., Zhao, M., Su, Y., and Long, Y.: Grey Correlation Analysis of Slake Durability of Red Bed Weak Rock, Journal of Hunan University (Natural Sciences), 33, 16–20, 2006.
Marat, A. R., Tamas, T., Samsudean, C., and Gheorghiu, R.: Physico-Mechanical and Mineralogical Investigations of Red Bed Slopes (Cluj-Napoca, Romania), B. Eng. Geol. Environ., 81, 78, https://doi.org/10.1007/s10064-021-02542-6, 2022.
Migon, P., Woo, K. S., and Kasprzak, M.: Landform Recognition in Granite Mountains in East Asia (Seoraksan, Republic of Korea, and Huangshan and Sanqingshan, China) – a Contribution of Geomorphology to the Unesco World Heritage, Quaest. Geogr., 37, 103–114, https://doi.org/10.2478/quageo-2018-0008, 2018.
Moonjun, R., Shrestha, D. P., Jetten, V. G., and van Ruitenbeek, F. J. A.: Application of airborne gamma-ray imagery to assist soil survey: A case study from Thailand, Geoderma, 289, 196–212, https://doi.org/10.1016/j.geoderma.2016.10.035, 2017.
Nance, H. S.: Interfingering of evaporites and red beds: an example from the queen/grayburg formation, Texas, Sediment Geol., 56, 357–381, 2015.
Ni, L. T., Zhong, J. H., Shao, Z. F., Li, Y., Mao, C., and Liu, S. X.: Characteristics, Genesis, and Sedimentary Environment of Duplex-Like Structures in the Jurassic Sediments of Western Qaidam Basin, China, J. Earth Sci.-China, 26, 677–689, https://doi.org/10.1007/s12583-015-0578-2, 2015.
Perez-Rey, I., Riquelme, A., Gonzalez-deSantos, L. M., Estevez-Ventosa, X., Tomas, R., and Alejano, L. R.: A multi-approach rockfall hazard assessment on a weathered granite natural rock slope, Landslides, 16, 2005–2015, https://doi.org/10.1007/s10346-019-01208-5, 2019.
Perri, F., Critelli, S., Martín-Algarra, A., Martín-Martín, M., Perrone, V., Mongelli, G., and Zattin, M.: Triassic redbeds in the Malaguide Complex (Betic Cordillera – Spain): Petrography, geochemistry and geodynamic implications, Earth-Sci. Rev., 117, 1–28, https://doi.org/10.1016/j.earscirev.2012.11.002, 2013.
Qiao, L. P., Wang, Z. C., and Huang, A. D.: Alteration of Mesoscopic Properties and Mechanical Behavior of Sandstone Due to Hydro-Physical and Hydro-Chemical Effects, Rock Mech. Rock Eng., 50, 255–267, https://doi.org/10.1007/s00603-016-1111-0, 2017.
Rainoldi, A. L., Franchini, M., Beaufort, D., Mozley, P., Giusiano, A., Nora, C., Patrier, P., Impiccini, A., and Pons, J.: Mineral reactions associated with hydrocarbon paleomigration in the Huincul High, Neuquen Basin, Argentina, Geol. Soc. Am. Bull., 127, 1711–1729, https://doi.org/10.1130/B31201.1, 2015.
San, N. E., Topal, T., and Akin, M. K.: Rockfall Hazard Assessment Around Ankara Citadel (Turkey) Using Rockfall Analyses and Hazard Rating System, Geotechnical and Geological Engineering, 38, 3831–3851, https://doi.org/10.1007/s10706-020-01261-1, 2020.
Triantafyllou, A., Mattielli, N., Clerbois, S., Da Silva, A. C., Kaskes, P., Claeys, P., Devleeschouwer, X., and Brkojewitsch, G.: Optimizing multiple non-invasive techniques (PXRF, pMS, IA) to characterize coarse-grained igneous rocks used as building stones, J. Archaeol. Sci., 129, 105376, https://doi.org/10.1016/j.jas.2021.105376, 2021.
Uchida, E., Ogawa, Y., Maeda, N., and Nakagawa, T.: Deterioration of stone materials in the Angkor monuments, Cambodia, Eng. Geol., 55, 101–112, https://doi.org/10.1016/S0013-7952(99)00110-6, 2000.
Underwood, S. J., Schultz, M. D., Berti, M., Gregoretti, C., Simoni, A., Mote, T. L., and Saylor, A. M.: Atmospheric circulation patterns, cloud-to-ground lightning, and locally intense convective rainfall associated with debris flow initiation in the Dolomite Alps of northeastern Italy, Nat. Hazards Earth Syst. Sci., 16, 509–528, https://doi.org/10.5194/nhess-16-509-2016, 2016.
Wang, D., Li, X.-B., Peng, K., Ma, C., Zhang, Z., and Liu, X.: Geotechnical characterization of red shale and its indication for ground control in deep underground mining, J. Cent. South Univ., 25, 2979–2991, https://doi.org/10.1007/s11771-018-3968-4, 2018.
Wang, F. W., Chen, Y., Peng, X. L., Zhu, G. L., Yan, K. M., and Ye, Z. H.: The fault-controlled Chengtian landslide triggered by rainfall on 20 May 2021 in Songyang County, Zhejiang Province, China, Landslides, 19, 1751–1765, https://doi.org/10.1007/s10346-022-01891-x, 2022.
Wang, L., Wang, L., Zhang, W., Meng, X., Liu, S., and Zhu, C.: Time series prediction of reservoir bank landslide failure probability considering the spatial variability of soil properties, J. Rock Mech. Geotech., https://doi.org/10.1016/j.jrmge.2023.11.040, 2024.
Wang, M., Qi, Y. A., Li, D., Dai, M. Y., and Chang, Y. G.: Ichnofabrics and Their Environmental Interpretation from the Fluvial Deposits of the Middle Triassic Youfangzhuang Formation in Western Henan, Central China, J. Earth Sci.-China, 25, 648–661, https://doi.org/10.1007/s12583-014-0454-2, 2014.
Wang, Y., Liu, J., Yan, S., Yu, L., and Yin, K.: Estimation of probability distribution of shear strength of slip zone soils in Middle Jurassic red beds in Wanzhou of China, Landslides, 14, 2165–2174, https://doi.org/10.1007/s10346-017-0890-z, 2017.
Wang, Y., Tang, H., Huang, J., Wen, T., Ma, J., and Zhang, J.: A comparative study of different machine learning methods for reservoir landslide displacement prediction, Eng. Geol., 298, 106544, https://doi.org/10.1016/j.enggeo.2022.106544, 2022.
Wankmuller, C., Kunovjanek, M., and Mayrgundter, S.: Drones in emergency response-evidence from cross-border, multi-disciplinary usability tests, Int. J. Disast. Risk Re., 65, 102567, https://doi.org/10.1016/j.ijdrr.2021.102567, 2021.
Wild, K. M., Walter, P., and Amann, F.: The response of Opalinus Clay when exposed to cyclic relative humidity variations, Solid Earth, 8, 351–360, https://doi.org/10.5194/se-8-351-2017, 2017.
Wu, L. Z., Zhang, L. M., Zhou, Y., Xu, Q., Yu, B., Liu, G. G., and Bai, L. Y.: Theoretical analysis and model test for rainfall-induced shallow landslides in the red-bed area of Sichuan, B. Eng. Geol. Environ., 77, 1343–1353, https://doi.org/10.1007/s10064-017-1126-0, 2018.
Xia, K. Z., Chen, C. X., Zheng, Y., Zhang, H. N., Liu, X. M., Deng, Y. Y., and Yang, K. Y.: Engineering geology and ground collapse mechanism in the Chengchao Iron-ore Mine in China, Eng. Geol., 249, 129–147, https://doi.org/10.1016/j.enggeo.2018.12.028, 2019.
Xue, Y., Wang, Q., Ma, L., Yu, Y., and Zhang, R.: Mechanisms and controlling factors of heave in summer for high-speed railway cutting: A case study of Northwest China, Constr. Build. Mater., 365, 130061, https://doi.org/10.1016/j.conbuildmat.2022.130061, 2023.
Yan, L. B., Peng, H., Zhang, S. Y., Zhang, R. X., Kasanin-Grubin, M., Lin, K. R., and Tu, X. J.: The Spatial Patterns of Red Beds and Danxia Landforms: Implication for the formation factors-China, Sci. Rep.-UK, 9, 1961, https://doi.org/10.1038/s41598-018-37238-7, 2019.
Yang, Y., Zhou, J., Xu, F., and Xing, H.: An Experimental Study on the Water-Induced Strength Reduction in Zigong Argillaceous Siltstone with Different Degree of Weathering, Adv. Mater. Sci. Eng., 2016, 4956986, https://doi.org/10.1155/2016/4956986, 2016.
Yao, H., Jia, S., Gan, W., Zhang, Z., and Lu, K.: Properties of Crushed Red-Bed Soft Rock Mixtures Used in Subgrade, Adv. Mater. Sci. Eng., 2016, 624974, https://doi.org/10.1155/2016/9624974, 2016.
Zha, F., Huang, K., Kang, B., Sun, X., Su, J., Li, Y., and Lu, Z.: Deterioration Characteristic and Constitutive Model of Red-Bed Argillaceous Siltstone Subjected to Drying-Wetting Cycles, Lithosphere-US, 2022, 8786210, https://doi.org/10.2113/2022/8786210, 2022.
Zhang, M., Yin, Y., and Huang, B.: Mechanisms of rainfall-induced landslides in gently inclined red beds in the eastern Sichuan Basin, SW China, Landslides, 12, 973–983, https://doi.org/10.1007/s10346-015-0611-4, 2015.
Zhang, S., Xu, Q., and Hu, Z. M.: Effects of rainwater softening on red mudstone of deep-seated landslide, Southwest China, Eng. Geol., 204, 1–13, https://doi.org/10.1016/j.enggeo.2016.01.013, 2016.
Zhang, W., Lin, S., Wang, L., Wang, L., Jiang, X., and Wang, S.: A novel creep contact model for rock and its implement in discrete element simulation, Comput. Geotech., 167, 106054, https://doi.org/10.1016/j.compgeo.2023.106054, 2024.
Zhang, Y., Li, F., and Chen, J.: Analysis of the interaction between mudstone and water, Journal of Engineering Geology, 16, 22–26, 2008.
Zhang, Z., Gao, W., Zeng, C., Tang, X., and Wu, J.: Evolution of the disintegration breakage of red-bed soft rock using a logistic regression model, Transp. Geotech., 24, 100382, https://doi.org/10.1016/j.trgeo.2020.100382, 2020.
Zhang, Z. H., Chen, X. C., Yao, H. Y., Huang, X., and Chen, L. W.: Experimental Investigation on Tensile Strength of Jurassic Red-Bed Sandstone under the Conditions of Water Pressures and Wet-Dry Cycles, KSCE J. Civ. Eng., 25, 2713–2724, https://doi.org/10.1007/s12205-021-1404-z, 2021.
Zhang, Z. L., Wang, T., Wu, S. R., Tang, H. M., and Liang, C. Y.: The role of seismic triggering in a deep-seated mudstone landslide, China: Historical reconstruction and mechanism analysis, Eng. Geol., 226, 122–135, https://doi.org/10.1016/j.enggeo.2017.06.001, 2017.
Zhao, M., Liu, X., and Su, Y.: Experimental studies on engineering properties of red bed material containing slaking rock, Chinese Journal of Geotechnical Engineering, 27, 667–671, 2005.
Zhou, C., Yu, L., Huang, Z., Liu, Z., and Zhang, L.: Analysis of microstructure and spatially dependent permeability of soft soil during consolidation deformation, Soils Found., 61, 708–733, https://doi.org/10.1016/j.sandf.2021.02.004, 2021.
Zhou, C., Hu, Y., Xiao, T., Ou, Q., and Wang, L.: Analytical model for reinforcement effect and load transfer of pre-stressed anchor cable with bore deviation, Constr. Build. Mater., 379, 131219, https://doi.org/10.1016/j.conbuildmat.2023.131219, 2023a.
Zhou, C., Liu, Z., Xue, Y., Li, Y., Fan, X., Chen, W., and Sun, P.: Some thoughts on basic research of red beds disaste, Journal of Engineering Geology, 31, 689–705, https://doi.org/10.13544/j.cnki.jeg.2022-0842, 2023b.
Zhu, B., Hu, H., and Chen, Q.: Preliminary study on the characteristics and hazards of M-shaped roadcut slope in red beds, Journal of Engineering Geology, 11, 411–415, 2003.