the Creative Commons Attribution 4.0 License.
the Creative Commons Attribution 4.0 License.
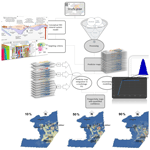
Rare earth elements associated with carbonatite–alkaline complexes in western Rajasthan, India: exploration targeting at regional scale
Alok Porwal
Manikandan Sundaralingam
Ignacio González-Álvarez
Amber Markan
Karunakar Rao
A two-stage fuzzy inference system (FIS) is applied to prospectivity modelling and exploration-target delineation for rare earth element (REE) deposits associated with carbonatite–alkaline complexes in the western part of the state of Rajasthan in India. The design of the FIS and selection of the input predictor map are guided by a generalised conceptual model of carbonatite–alkaline-complex-related REE mineral systems. In the first stage, three FISs are constructed to map the fertility and favourable geodynamic settings, favourable lithospheric architecture for fluid transportation and favourable shallow crustal (near-surface) emplacement architecture, respectively, for REE deposits in the study area. In the second stage, the outputs of the above FISs are integrated to map the prospectivity of REE deposits in the study area. Stochastic and systemic uncertainties in the output prospectivity maps are estimated to facilitate decision-making regarding the selection of exploration targets. The study led to the identification of prospective targets in the Kamthai–Sarnu-Dandeli and Mundwara regions, where detailed project-scale ground exploration is recommended. Low-confidence targets were identified in the Siwana ring complex region, north and northeast of Sarnu-Dandeli, south of Barmer, and south of Mundwara. Detailed geological mapping and geochemical sampling together with high-resolution magnetic and radiometric surveys are recommended in these areas to increase the level of confidence in the prospectivity of these targets before undertaking project-scale ground exploration. The prospectivity-analysis workflow presented in this paper can be applied to the delineation of exploration targets in geodynamically similar regions globally, such as Afar province (East Africa), Paraná–Etendeka (South America and Africa), Siberia (Russia), East European Craton–Kola (eastern Europe), Central Iapetus (North America, Greenland and the Baltic region) and the pan-superior province (North America).
- Article
(4412 KB) - Full-text XML
- BibTeX
- EndNote
According to the International Union of Pure and Applied Chemistry (IUPAC), the term rare earth elements (REEs) includes yttrium (Y), scandium (Sc) and the lanthanides (lanthanum, La; cerium, Ce; praseodymium, Pr; neodymium, Nd; promethium, Pm; samarium, Sm; europium, Eu; gadolinium, Gd; terbium, Tb; dysprosium, Dy; holmium, Ho; erbium, Er; thulium, Tm; ytterbium, Yb; and lutetium, Lu). Because of their increasing use in environment-friendly high-technology industries, REEs are widely considered as the resources of the future (e.g. Goodenough et al., 2018; Wall, 2021). Most countries have classified REEs as “critical minerals and metals” because of their strategic importance and the projected gap between their future demand and supply (Goodenough et al., 2018; González-Álvarez et al., 2021, and references therein).
In spite of significant efforts into developing technology for recovering and recycling REEs from discarded devices (Binnemans et al., 2013), geological resources are likely to remain the primary sources of REEs in the foreseeable future (Goodenough et al., 2018). Several classification schemes for REE deposits have been proposed by different workers based on geological associations and settings, for example, Chakhmouradian and Wall (2012), Jaireth et al. (2014), Wall (2014), Goodenough et al. (2016), Verplanck and Hitzman (2016), and Simandl and Paradis (2018). In general, REE deposits can be broadly classified into those formed by high-temperature (magmatic and hydrothermal) processes and those formed by low-temperature (mechanical and residual concentration) processes (e.g. Wall, 2021). Although the majority of Indian production of REEs comes from low-temperature deposits such as regolith-hosted and heavy-mineral placers (IBM yearbook 2018, 2019), the bulk of geological resources are in high-temperature magmatic deposits, particularly those associated with carbonatites (e.g. Bayan Obo, Inner Mongolia, China; Mount Weld, Western Australia; Maoniuping, South China; Mountain Pass, USA; González-Álvarez et al., 2021 and references therein).
India ranks sixth in terms of production of REEs and fifth in terms of resources (USGS, 2021). All of India's production comes from monazite-bearing beach sands along the eastern and western coasts (IBM yearbook 2018, 2019). Since India has 29 out of the total 527 globally reported carbonatite occurrences (Woolley and Kjarsgaard, 2008a), there is significant latent potential for carbonatite-related REE deposits in the country. Currently, there are no studies available, at least in the public domain, on systematic delineation of prospective REE exploration targets in India.
Mineral prospectivity modelling is a widely used predictive tool for identifying exploration target areas for mineral exploration. Implemented in a GIS environment, it involves the integration of “predictor maps” that represent a set of mappable exploration criteria for the targeted deposit type. Typically, conceptual mineral systems models are used to identify exploration criteria (Porwal and Kreuzer, 2010; Porwal and Carranza, 2015). The integration is done through either linear or non-linear mathematical functions (Bonham-Carter, 1994; Porwal, 2006; Porwal and Carranza, 2015). Depending on how the model parameters are estimated, that is, whether based on training data comprising attributes of known deposits or on expert knowledge, these models are classified as data-driven or knowledge-driven. Data-driven approaches require a sizeable sample of known deposits of the targeted deposit type for estimating the model parameters, while knowledge-driven approaches use expert knowledge for estimating model parameters. Fuzzy-logic-based approaches are the most widely used knowledge-driven approaches to prospectivity modelling. These approaches have evolved from the Prospector (Duda et al., 1978, 1979, 1980), which was the earliest knowledge-based expert system that utilised fuzzy operators in a Bayesian network (Porwal et al., 2015).
The outputs of both data-driven and knowledge-driven prospectivity models are subject to two types of uncertainties (Porwal et al., 2003; Lisitsin et al., 2014), namely, systemic (or epistemic) and stochastic (or aleatory). Systemic uncertainties arise from the incomplete understanding of the geological process involved in the formation of the mineral deposit, leading to imperfect models. Stochastic uncertainties arise from the limitations of the primary and derivative processed datasets, including the algorithms used to derive them. These uncertainties are the results of inaccuracy or imprecision in measurements and observations, data interpolations, and inconsistent data coverage (Porwal et al., 2003; McCuaig et al., 2009; Lisitsin et al., 2014). However, most published prospectivity modelling studies do not specifically deal with uncertainties in model outputs.
There are very few published studies on REE prospectivity modelling. Ekmann (2012) published a study of REEs in coal deposits in the United States. In one of the first GIS-based prospectivity modelling studies for REEs, Aitken et al. (2014) used a fuzzy-logic-based model to delineate prospective targets for pegmatite-, carbonatite- and vein-hosted REEs in the Gascoyne region of Western Australia. This study was part of a larger multi-commodity prospectivity study of the Gascoyne Province. Sadeghi (2017) carried out a regional-scale GIS-based prospectivity analysis for REEs in the Bergslagen district of Sweden, targeting iron-apatite- and skarn-associated deposits using the weights of evidence and weighted-overlay models. Bertrand et al. (2017) used database querying to analyse the prospectivity for REEs as by-products in known mineral deposits in Europe. In a recent study, Morgenstern et al. (2018) analysed the potential of REEs in New Zealand using a multi-stage fuzzy inference system (FIS).
This contribution describes the first systematic and comprehensive prospectivity modelling exercise aimed at identifying exploration targets for REE associated with carbonatite–alkaline complexes in western Rajasthan, India (Fig. 1). Although it is a well-established carbonatite province that is widely considered prospective for REE deposits, only a single REE deposit has been identified in the province so far. We employ fuzzy inference system (FIS), which is a knowledge-driven artificial intelligence technique, to identify and delineate prospective targets for REEs (except Pm and Sc; Pm is an unstable element and Sc is not an element sourced from carbonatite–alkaline complexes) in the study area. The inputs to the FIS were identified based on a generalised mineral systems model for carbonite–alkaline-complex-related REEs, which was further used to guide the design of the FIS. To support decision-making regarding the delineated targets, uncertainties in the output model were also estimated. The prospectivity-analysis workflow presented in this paper can be applied to other geodynamically similar regions (with mantle-plume-related intracontinental extensional settings) globally for exploration targeting, e.g. in eastern Africa, South and North America, Russia, eastern Europe, Greenland and the Baltic region.
The study area is located in the state of Rajasthan in northwestern India (Fig. 1). This area was chosen because it is a known major carbonatite province of India, and well-integrated public domain datasets are available. Geologically, the study area contains igneous and sedimentary formations ranging in age from the Neoproterozoic to Holocene. Neoproterozoic Erinpura and Jalore granites, along with a few outcrops of the Mesoproterozoic Delhi Supergroup, occur in the southeastern part of the study area (Fig. 1). The eastern part of the study area comprises extrusive and intrusive igneous rocks belonging to the Neoproterozoic Malani Igneous Suite that is mostly covered by a thick horizon of Holocene wind-blown sand. Sedimentary sequences belonging to the Late Neoproterozoic Marwar Supergroup, Jurassic Jaisalmer, Cretaceous Sarnu–Fatehgarh, Tertiary Barmer (Palaeocene) and Akli (Eocene), and Quaternary Uttarlai formations (Pleistocene to Holocene) (Roy and Jakhar, 2002; Ramakrishnan and Vaidyanadhan, 2008; Singh et al., 2016) occur in the central and western parts around Barmer and Jaisalmer towns (Fig. 1).
Carbonatite–alkaline complexes of the Cretaceous age occur in the Mer-Mundwara area in the eastern part of the study area and the Sarnu-Dandali area in the central part of the study area (Fig. 1; Table A1). The Mer-Mundwara carbonatite–alkaline complex intrudes the Neoproterozoic Erinpura Granite and displays a characteristic ring structure, wherein the alkaline-mafic rock suites form two ring structures and a dome (Pande et al., 2017). Carbonatites mainly occur in the form of linear dykes at Mer-Mundwara. The Sarnu-Dandeli complex covers a relatively large area on the eastern shoulder of the Barmer Basin. The carbonatites occur mainly as scattered plugs and dykes that are covered by Quaternary sand, intruding the Neoproterozoic Malani igneous suite and the Cretaceous Sarnu formation (Vijayan et al., 2016; Sheth et al., 2017). The Sarnu-Dandeli complex also includes more minor occurrences of carbonatites in the Danta-Langera-Mahabar and Kamthai areas. The Kamthai plug is considered to be highly prospective for REEs (Bhushan and Kumar, 2013).
The study area is dissected by the Barmer rift, which continues southwards through the state of Gujarat into the Cambay Basin. The Barmer rift is a failed, roughly north–south-trending, extensional intracratonic rift (Fig. 1) that was active during the Late Cretaceous to Eocene (Dolson et al., 2015). A long-lasting extensional regime predating the Deccan volcanism existed in northwestern India, peaking with the Seychelles rifting at the Cretaceous–Paleogene boundary and the emplacement of the main phase of Deccan volcanics at ca. 65 Ma (Devey and Stephens, 1992; Allegre et al., 1999; Chenet et al., 2007; Collier et al., 2008; Ganerød et al., 2011; Bladon et al., 2015a, b). The well-preserved Cretaceous carbonatite–alkaline complexes of the study area represent a young carbonatite magmatism episode (∼ 68 Ma) that is coeval with the initial magmatism of the Deccan Large Igneous Province (LIP) and is related to the India–Seychelles break-up and northward drifting of India over the Réunion mantle plume (Devey and Stephens, 1992; Basu et al., 1993; Simonetti et al., 1995; Allegre et al., 1999; Ray and Pande, 1999; Ray and Ramesh, 1999; Ray et al., 2000; Chenet et al., 2007; Collier et al., 2008; Sheth et al., 2017; Chandra et al., 2018).
The public domain geoscience datasets used in the study, which include geological, geophysical, topographic and satellite data, were mainly sourced from the Bhukosh portal (https://bhukosh.gsi.gov.in/Bhukosh/MapViewer.aspx, last access: 10 October 2019) of the Geological Survey of India (GSI). Table 1 summarises the sources, scales and other details about the individual datasets. Figure 2 depicts the outline of the methodology used in this study, which is discussed in detail in the next sections.
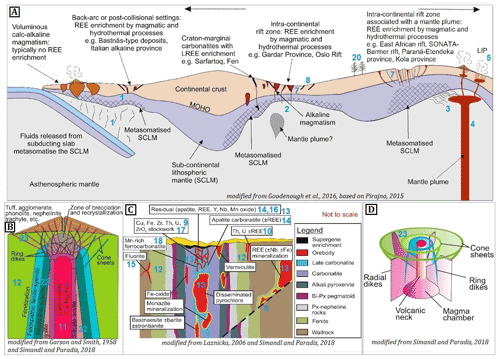
Figure 3Idealised genetic model of a carbonatite–alkaline-complex-related REE mineral system (adapted from Aranha et al., 2022) cross-referenced to processes listed in Table 2 through the numbers in blue. Panel (a) depicts the fertility and geodynamic setting along with the transport architecture on a regional scale. Panels (b–d) focus on the emplacement architecture at the camp-to-prospect-scale. Panel (b) shows the idealised geometry of the intrusion and the relation of carbonatites and associated alkaline rocks and fenitisation. Panel (c) presents the near-surface structural architecture and the spatial distribution of associated rocks. Panel (d) displays the idealised geometry of a carbonatite–alkaline intrusion and the relationship between the magma chamber, ring dykes, cone sheets and radial dykes.
Table 2Conceptual REE mineral systems model (adapted from Aranha et al., under review). The index numbers correlate to the numbers in blue in Fig. 3.
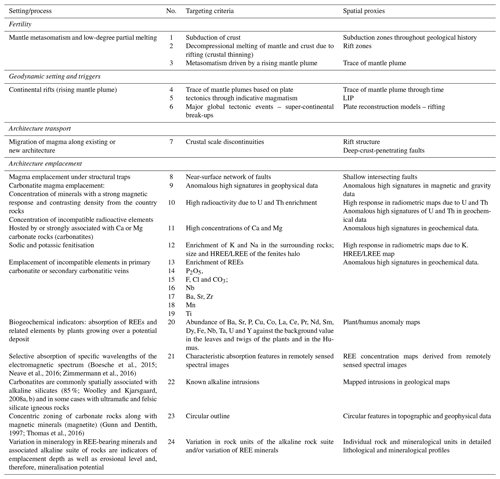
In this study, we used the generalised conceptual model of carbonatite–alkaline-complex-related REE mineral systems developed by Aranha et al. (in review) based on the framework proposed by McCuaig and Hronsky (2014). Figure 3 illustrates the main features of the model. The main components of the mineral systems are compiled in Table 2 and briefly summarised in the following paragraphs.
Fertile source region. Metasomatised pockets of the subcontinental lithospheric mantle (SCLM) are considered to be the fertile source regions for carbonatite–alkaline complexes (Jones et al., 2013) as well as REE deposits associated with these complexes, as the processes of metasomatism also cause enrichment of REE and other incompatible elements (Zheng, 2012, 2019). The metasomatism of the SCLM involves one of the following processes: (1) lithospheric subduction into the SCLM (no. 1 in Fig. 3 and Table 2; Duke, 2009; Duke et al., 2014; Goodenough et al., 2016); (2) anatexis-induced mixing of subducting crustal units with the SCLM (no. 2 in Fig. 3 and Table 2; Jones et al., 2013 and references therein); or (3) metasomatism induced by ascending mantle plumes (no. 3 in Fig. 3 and Table 2; Simonetti et al., 1995, 1998; Bell and Tilton, 2002; Bell and Simonetti, 2010; Ernst and Bell, 2010).
Geodynamic setting. Carbonatite–alkaline complexes and related REE deposits generally occur in extensional intra-continental rifts and large igneous provinces (LIPs) (no. 4, 5, 6 in Fig. 3 and Table 2; Woolley and Kjarsgaard 2008a; Woolley and Bailey, 2012; Pirajno, 2015; Simandl and Paradis, 2018). Extensional tectonic settings and associated LIPs are manifestations of mantle plumes (Simonetti et al., 1995, 1998; Bell and Tilton, 2002; Bell and Simonetti, 2010; Ernst and Bell, 2010), which also induce metasomatism of the SCLM. As a result, fertile source regions of, and favourable geodynamic setting for, REE deposits related to the carbonatite–alkaline complex are interlinked.
Architecture. Carbonatite–alkaline complexes and related REE mineral systems derive fluids from the SCLM through large-scale permeable networks of trans-lithospheric structures. Most carbonatite–alkaline complexes are found spatially associated with crustal-scale faults, rifts and shear zones at regional scales (Ernst and Bell, 2010; Woolley and Bailey, 2012; Pirajno, 2015; Simandl and Paradis, 2018; Spandler et al., 2020). Therefore, lithosphere-scale structures form favourable plumbing structures for carbonatite–alkaline-complex-related REE deposits (no. 7 in Fig. 3 and Table 2). Upper crustal faults, shallow discontinuity structures and joints serve as pathways for focussing fluids to near-surface levels and also form structural traps (no. 8 in Fig. 3 and Table 2; Ernst and Bell, 2010; Skirrow et al., 2013; Jaireth et al., 2014).
The reaction of the carbonatite–alkaline magma with the country-rock results in the formation of Ca and Mg silicates and removal of CO2, dissolved P and F (Skirrow et al., 2013; Jaireth et al., 2014). The above reactions may cause REEs to deposit in silicate minerals along the country-rock interface (Anenburg and Mavrogenes 2018; Anenburg et al., 2020). Enrichment of incompatible elements such as REEs, U, Th, Nb, Ba, Sr, Zr, Mn, Fe and Ti in the fluids occur due to liquid immiscibility, especially in liquids rich in alkalis which promote REE solubility (no. 10, 13, 14, 15, 16, 17, 18, 19 in Fig. 3 and Table 2; Cordeiro et al., 2010; Skirrow et at., 2013; Jaireth et al., 2014; Pirajno, 2015; Mitchell, 2015; Chakhmouradian et al., 2015; Stoppa et al., 2016; Poletti et al., 2016; Giovannini et al., 2017; Simandl and Paradis, 2018; Spandler et al., 2020; Anenburg et al., 2020). Carbonatite–alkaline complexes are often enriched in ferromagnesian minerals that cause well-defined magnetic and gravity anomalies (no. 9 in Fig. 3 and Table 2; Gunn and Dentith, 1997; Thomas et al., 2016). Fenitisation often enriches country rocks in K and Na (no. 12 in Fig. 3 and Table 2; Le Bas, 2008; Elliott et al., 2018). In alkali-rich intrusions, LREEs are retained in the primary carbonatite while HREEs tend to concentrate in fenites, particularly K-fenites, whereas in silica-rich or alkali-poor intrusions, HREEs remain in the carbonatite (Anenburg et al., 2020). Size and HREE/LREE concentration of the fenites halo are major proxies.
REE mineralisation in the carbonatites can be in the form of primary REE-bearing minerals (e.g. Mountain Pass, Mariano, 1989; Castor, 2008; Verplanck and Van Gosen, 2011; Van Gosen et al., 2017) or by secondary hydrothermal activity, including in situ replacement, or from late magmatic fluid phases evolved from the carbonatite magmas (Verplanck and Van Gosen, 2011; Skirrow et at., 2013; Jaireth et al., 2014; Van Gosen et al., 2017). Primary REE-bearing cumulates include perovskite, pyrochlore, apatite and calcite, while late-stage REE-bearing minerals include bastnäsite, parisite and synchysite that form from the redistribution of soluble primary phases such as ancylite, burbankite and carbocernaite (no. 24 in Table 2; Verplanck and Van Gosen, 2011; Skirrow et al., 2013; Van Gosen et al., 2017; Andersen et al., 2017; Anenburg et al., 2020; Kozlov et al., 2020).
The above conceptual model for carbonatite–alkaline-related REE mineral systems was translated into a “targeting model”, which is a compilation of processes whose responses can be mapped directly or indirectly from the publicly available datasets for the study area listed in Table 1. The targeting model was used to identify regional-scale mappable targeting criteria for REE deposits in the study area (Table 3).
The mappable targeting criteria for REE deposits in the study area were represented in the form of GIS layers or predictor maps for inputting into the FIS. The details of the primary data, the algorithms, and GIS tools and techniques used to generate input predictor maps are provided in Table 3. Since there is a 1–2 orders of difference in the spatial resolution of the input datasets (ranging from ∼ 100 m for airborne magnetic data to ∼ 10 km for ground gravity data), we chose a trade-off grid cell size of 3 km for generating the input predictor maps. The same grid cell size was used for prospectivity analysis. This grid cell size corresponded to the size of typical carbonatite–alkaline-complex occurrences in the study area.
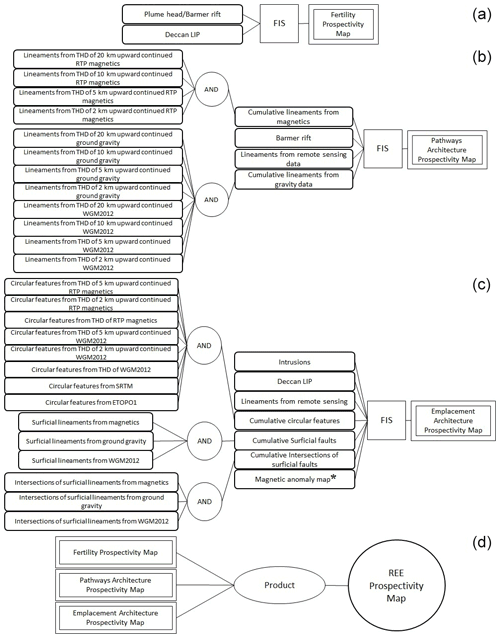
Figure 4The multi-stage FIS for REE prospectivity mapping in the study area. (a) FIS for generating fuzzy prospectivity maps for fertile sources and favourable geodynamics settings. (b) FIS for generating fuzzy prospectivity maps for favourable lithospheric architecture for transportation of REE-enriched carbonatite–alkaline magma. (c) FIS for generating fuzzy prospectivity maps for favourable shallow crustal (near-surface) architecture for emplacement of carbonatite–alkaline complexes. (d) Second-stage FIS combines the above three prospectivity maps obtained from the first stage and generates the final outputs. ∗ All the input maps in (a–c) are Euclidean distance maps, except for the magnetic anomaly map in (c).
The predictor maps were integrated using FIS (Fig. 4) to generate REE prospectivity maps of the study area. The theory of the FIS-based modelling approach and implementation for mineral prospectivity modelling is provided by Porwal et al. (2015) and Chudasama et al. (2016). Several open-source software packages and libraries for implementing FIS are available in the public domain (e.g. FISDeT, Castellano et al., 2017; FisPro, R package “FuzzyR”; Python library “fuzzy expert”). However, in the present study, we used the commercial software Fuzzy Logic Toolbox of MathWorks® to implement the model. The concepts and theory of fuzzy logic, as well as the procedures for designing and implementing FIS using the Fuzzy Logic Toolbox, are explained in detail in the documentation that can be freely accessed at https://mathworks.com/help/fuzzy/fuzzy-inference-process.html.
The modelling was implemented in the following steps.
1. Fuzzification of numeric predictor maps. In the first step, all numeric predictor maps (e.g. the predictor map showing distance to structural lineaments) were converted into fuzzy predictor maps (e.g. proximity to structural lineaments) using membership functions such as linear, piece-wise linear (trapezoidal) or Gaussian (Table 4). However, the output fuzzy membership values of a predictor map are dependent on the shape of the membership function used, which in turn is dependent on the mathematical parameters that define the function, e.g. mean and standard deviation for Gaussian functions and slope and intercept for linear functions.
Because there are insufficient known deposits to use as training data for optimising the fuzzy membership functions, we quantified uncertainty arising from using sub-optimal function parameters (termed “systemic uncertainty”; Porwal et al., 2003; Lisitsin et al., 2014). The algorithm utilising Monte Carlo simulations described by Lisitsin et al. (2014) and Chudasama et al. (2017) was used to estimate model uncertainties. In this approach, instead of using point values for the parameters of the fuzzy membership functions, we used beta-PERT distributions conforming to the possible variations of these point values. The beta is a bounded distribution that is widely used when there are no training data, and the only information available is the expert knowledge about the optimistic, most likely and pessimistic values (Johnson et al., 1995). The parameters of the beta functions (optimistic, most likely and pessimistic values) were assigned based on a geological evaluation of the decay of the influence of a targeting criteria with distance (Table 4). A series of Monte Carlo simulations were then carried out to estimate the values of the parameters at 10 %, 50 % and 90 % probability levels, which were respectively used to generate three fuzzy maps at 10 %, 50 % and 90 % probability levels for each predictor map.
However, the present work has not quantified other systemic uncertainties arising from the choice of the membership function, FIS structure, and choice of distribution.
2. FIS-based prospectivity modelling. In the second step, a multi-stage FIS was designed to replicate the geological reasoning used by an exploration geologist for delineating regional-scale exploration targets.
In the first stage, a series of FISs were developed to generate fuzzy prospectivity maps for individual components of the REE mineral systems by combining their respective fuzzy predictor maps. The FISs for fertility and geodynamic settings, whole lithosphere architecture and near-surface architecture (Fig. 4) comprised 5, 8 and 11 fuzzy if–then rules, respectively, which are shown in Tables A2, A3 and A4, respectively. Since each predictor map was converted into three fuzzy maps at 10 %, 50 % and 90 % probability levels, the outputs of this step were three fuzzy prospectivity maps for each mineral systems component at 10 %, 50 % and 90 % probability levels.
In the second stage, the above three sets of fuzzy prospectivity maps were combined using the fuzzy product operator (Fig. 4D) to generate three REE prospectivity maps of the study area at 10 %, 50 % and 90 % probability levels.
3. Generation of confidence map. In the third step, stochastic uncertainties, which arise from the limitations of public-domain datasets and procedures used for generating the predictor maps, were quantified in terms of confidence values for each predictor map using the Sherman–Kent scale (Jones and Hillis, 2003; Kreuzer et al., 2008) as described by Porwal et al. (2003), González-Álvarez et al. (2010) and Joly et al. (2012). The confidence value for each predictor map was assigned based on the degree of representativeness of the predictor map, i.e. how well it represents the mineralisation process it seeks to map. A predictor map was assigned a high confidence value if it directly mapped the targeting criteria and a low confidence value if it indirectly mapped the response of the targeting criterion. The confidence factor also captured the fidelity and precision of the primary dataset from which the input was derived. The confidence factor for all predictor maps, along with the justifications, are given in Table 5. The output confidence map was generated by combining the confidence factors of different predictor maps using the same fuzzy inference systems that were used for prospectivity modelling.
Finally, the three REE prospectivity maps of the study area at 10 %, 50 % and 90 % probability levels were colour-coded blue to red and draped over the confidence map for viewing as 3D surface models. In the 3D surface models, the colours represented prospectivity (blue tones signify low prospectivity and red tones signify high prospectivity), and elevation represented confidence (depressions signify low confidence and elevations signify high confidence).
Table 6Matrix summarising the target areas quantified according to probability and confidence levels, and recommended exploration. Target serial numbers refer to the rectangle numbers in Fig. 5a–c.
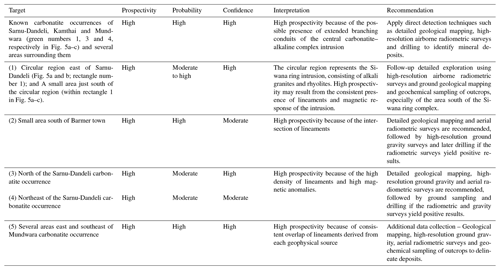
In the first stage, the first FIS maps REE fertility and favourable geodynamic settings (Fig. 4a and Table A2) by delineating areas that are likely to be underlain by plume-metasomatised SCLM. Considering the size of a typical mantle plume, these areas are expected to be very large. The second FIS maps favourable lithospheric architecture for the transportation of REE-enriched carbonatite–alkaline magma (Fig. 4b and Table A3) and narrows down the target areas identified by the first FIS to areas that are proximal to trans-lithospheric structures. The target areas demarcated by the second FIS are also relatively large, as immense trans-lithospheric structures, such as the 600 km long Barmer–Cambay rift, are expected to have a large zone of influence. The third FIS maps favourable shallow crustal (near-surface) architecture for the emplacement of carbonatite–alkaline complexes (Fig. 4c and Table A4) and further narrows down the target area to camp-size areas that are controlled by near-surface higher-order structures. These individual FISs in the first stage rely on simple logic-based rules to integrate the individual predictor maps (Tables A2–A4). The rules were framed based on our understanding of the REE mineral system. The use of the “and” operator in the “if” parts of the rules defining high prospectivity ensured that a pixel would get a high prospectivity value only if it is proximal to predictor features on all predictor maps. Similarly, the use of the “or” operator in the “if” parts of the rules defining low prospectivity ensured that a pixel would get a low prospectivity even if it is distal to predictor features on any one of the predictor maps. As a result, the extents of the areas with background (low) prospectivity are maximised, and high-prospectivity zones are narrowed down efficiently.
In the second stage of the multi-stage FIS, the output prospectivity maps of the individual components were integrated using the fuzzy product operator, which calculates the mathematical product of all input predictor maps (Bonham-Carter, 1994; Porwal et al., 2015). Since the individual FIS output values range between 0 and 1, it decreases the final integrated prospectivity values. The final outputs are shown as continuous-scale (relative) prospectivity maps at 10 %, 50 % and 90 % probability levels draped over the confidence map in Fig. 5a–c.
Conjunctive interpretations of prospectivity maps and confidence maps can help in making decisions regarding follow-up exploration, as summarised in Table 6.
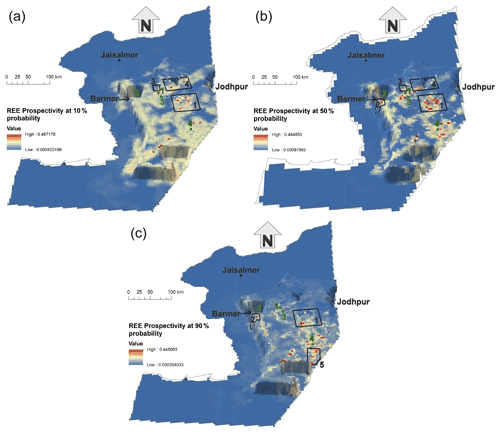
Figure 5Continuous-scale prospectivity maps at 10 %, 50 % and 90 % probability levels draped over the confidence layer, shown in (a), (b) and (c), respectively. The colours mark increasing prospectivity from low (blue) to high (red). The elevations mark high confidence in the data used for prospectivity modelling. Black balls indicate major cities, and green balls indicate known carbonatite occurrences; green numbers correspond to the known carbonatite occurrences: 1 – Sarnu-Dandeli, 2 – Danta-Langera-Mahabar, 3 – Kamthai, 4 – Mundwara. Areas marked with black numbered rectangles are discussed in Sect. 7.
Along with the known Mundwara, Sarnu-Dandeli and Kamthai carbonatite occurrences, high prospectivity (orange-red colours in Fig. 5a–c) occurs in areas immediately surrounding Sarnu-Dandeli and Mundwara at high probability and confidence levels. These areas may represent branching conduits of the central carbonatite–alkaline complex intrusion. Geological mapping and direct detection studies are recommended in these locations.
Areas of moderate to high prospectivity at low probability levels are mapped over a circular region east of Sarnu-Dandeli (Fig. 5a and b; rectangle number 1) and also over an area just south of the circular region (within rectangle 1 in Fig. 5a–c). The circular region corresponds to the Siwana ring intrusion, which consists of alkali granites and rhyolites. The Siwana ring intrusion is part of the Neoproterozoic Malani LIP (Bhushan and Mohanty, 1988). However, the Siwana ring intrusion has low prospectivity at high probability (Fig. 5c; rectangle number 1), while the smaller area to its south consistently has high prospectivity at high probability and confidence levels. The high values may be caused by the consistent presence of lineaments in this region and the magnetic response of the intrusion. It is noteworthy that although it is not a carbonatite–alkaline complex, the peralkaline Siwana ring complex does contain REE potential and has been assessed for REE mineralisation (Bhushan and Somani, 2019). Further assessment of this region is recommended, with detailed radiometric surveys, geological mapping and geochemical sampling, especially of the area south of the Siwana ring complex that has high prospectivity at high probability levels.
A small area south of Barmer has high prospectivity at high probability and moderate confidence levels (Fig. 5b and c; rectangle number 2). This area has high prospectivity due to the intersection of lineaments. Two more areas to the north and northeast of the Sarnu-Dandeli carbonatite occurrence have high prospectivity at moderate probability and confidence levels (Fig. 5a and b, rectangles 3 and 4, respectively). A high density of lineaments and high magnetic anomalies are the likely causes. Detailed geological mapping and aerial radiometric surveys are recommended at all three locations, followed by ground sampling and drilling if the radiometric surveys yield positive results.
Several areas east and southeast of Mundwara have high prospectivity at high probability and confidence levels (Fig. 5c; rectangle 5). This is likely due to the consistent overlap of lineaments derived from each geophysical source at these locations. Acquiring additional data such as detailed geological maps, ground gravity and aerial radiometric surveys would help in delineating the target zone in these areas.
The emplacement of carbonatite–alkaline complexes in the study area was related to the large-scale rifting and splitting of India from Madagascar and later from the Seychelles, which also triggered Deccan volcanism. A similar mode of origin is envisaged for several other carbonatite–alkaline complexes worldwide. Ernst and Bell (2010) have identified several carbonatite provinces that are emplaced in an extensional setting, associated with a mantle plume and a LIP. These include, along with the Deccan province, the Afar province (eastern Africa), Paraná–Etendeka (South America and Africa), Siberian province (Russia), East European Craton–Kola province (eastern Europe), Central Iapetus province (North America, Greenland and the Baltic region), and pan-superior province (North America). The methodologies described in this paper can be used for exploration targeting REEs in these provinces.
Furthermore, at the time of emplacement of these carbonatite–alkaline complexes, the Indian subcontinent was located close to Madagascar and the Seychelles. Therefore, similar complexes could occur in Madagascar and the Seychelles also. The Barmer rift is the northern extension of the Cambay rift, which forms a triple junction in western India along with the Kutch rift. Thus, carbonatite–alkaline complexes are also expected along the Cambay rift and Kutch rifts, also possibly along the offshore E–W-trending Gop and the NNW–SSE-trending West Coast rift zones on the western coast of India. Kala–Dongar (Sen et al., 2016) and Murud–Janjira (Sethna and D'Sa, 1991) are known minor occurrences of carbonatites along the Kutch and West Coast rift zone, respectively. Moreover, the Gop rift is the western extension of the Son–Narmada–Tapti (SONATA) rift zone, along which several significant occurrences of the Chhota–Udepur carbonatite district are found. A similar study may help in identifying exploration targets for REEs in these regions. Paleo-reconstruction of the geography at the time when these complexes were being emplaced and analysing the prospectivity of the entire Deccan province (including western India, Madagascar and the Seychelles) may help identify more prospective targets for carbonatite-related REEs.
Rare earth elements comprise 17 metallic elements that are considered as “critical metals” for future development of environmentally friendlier and technologically based societies. India's production entirely comes from secondary beach placer deposits on the western and eastern coasts. Even though just one primary economic-grade deposit of REE is identified in India, there is significant latent potential for carbonatite-related REE deposits. This study has developed a knowledge-driven, GIS-based prospectivity model for exploration targeting of REEs associated with carbonatite–alkaline complexes in the western Rajasthan, northwestern India.
The generalised mineral systems model for carbonatite–alkaline-complex-related REEs described by Aranha et al. (under review) was used to identify regional-scale targeting criteria for REEs in the study area. Several predictor maps were derived from public-domain geological, geophysical and satellite data based on the mineral systems model. A multi-stage FIS was constructed to represent the different components of the mineral system. The first stage of the multi-stage FIS comprises three individual FISs to represent (1) plume-metasomatised SCLM in an extensional regime that make up fertile source regions for REE-bearing fluids and favourable geodynamic settings, (2) trans-lithospheric structures that provide favourable lithospheric architecture for the transportation of REE-enriched carbonatite–alkaline magma, and (3) near-surface higher-order structures that make up a shallow crustal architecture facilitating emplacement of carbonatite–alkaline complexes.
Systemic uncertainties associated with the fuzzification of the predictor maps was quantified based on the procedure described by Lisitsin et al. (2014) and Chudasama et al. (2017) that produced prospectivity maps at 10 %, 50 % and 90 % confidence levels. Stochastic uncertainties associated with the primary data used and the processing methods adopted to derive predictor maps were quantified based on the procedure described by Porwal et al. (2003), producing a confidence layer over which the prospectivity maps were draped.
Based on the results, a structural control over the emplacement of carbonatite–alkaline complexes is clearly recognised. The following are the recommendations based on the results of this study. Detailed project-scale ground exploration is recommended for the Kamthai–Sarnu-Dandeli and Mundwara regions and their immediate surroundings, where areas of high prospectivity are mapped at high probability levels. Exploration of the Siwana ring complex is recommended, particularly for the high prospectivity region to its south. Detailed geological mapping, high-resolution ground gravity and aerial radiometric surveys should be carried out in the regions to the north and northeast of Sarnu-Dandeli, south of Barmer, and the south of Mundwara to better resolve and delineate targets for ground exploration.
The prospectivity-analysis workflow presented in this paper can be applied to other geodynamically similar regions globally for targeting geological provinces for follow-up exploration such as the Deccan province, the Afar province (eastern Africa), Paraná–Etendeka (South America and Africa), Siberian province (Russia), East European Craton–Kola province (eastern Europe), Central Iapetus province (North America, Greenland and the Baltic region), and pan-superior province (North America).
Table A1Location, physiography and geological setting of carbonatite–alkaline complexes in NW India.
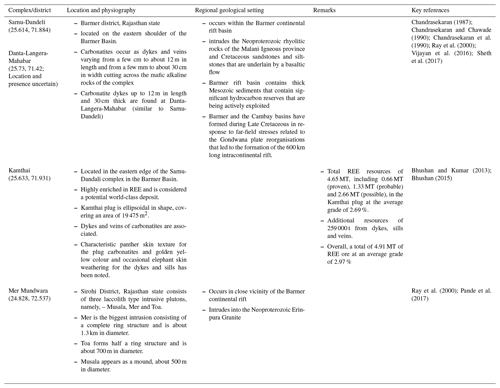
Table A2Fuzzy if–then rules used for generating fuzzy prospectivity maps for fertile sources and favourable geodynamics settings.

Table A3Fuzzy if–then rules used for generating fuzzy prospectivity maps for favourable lithospheric architecture for transportation of REE-enriched carbonatite–alkaline magma.
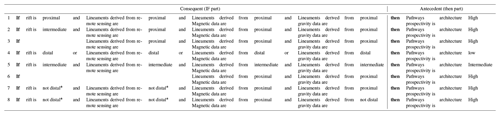
∗ “Not distal” includes all fuzzy sets other than the “distal” set. In this case, it includes both the “proximal” and “intermediate” fuzzy sets.
The study mainly used public domain datasets that have been summarised in Table 1 and in Sect. 3.
MA and AP conceptualised the study. MA, AP and MS designed the methodology. MA and MS carried out the preprocessing and feature extraction. Formal analysis was carried out by MA, AP and IGA. The investigation was carried out by MA, AP and IGA. MA, AP, AM, and KR organised the resources. Data curation was carried out by MA, MS and AM. MA, AP, and IG wrote the paper.
The contact author has declared that neither they nor their co-authors have any competing interests.
Publisher's note: Copernicus Publications remains neutral with regard to jurisdictional claims in published maps and institutional affiliations.
This article is part of the special issue “State of the art in mineral exploration”. It is a result of the EGU General Assembly 2020, 3–8 May 2020.
This paper benefited greatly from valuable discussions with Majid Keykhay-Hosseinpoor and Bijal Chudasama, for which they are thanked. Juan Alcade is thanked for putting together this special issue. We express our gratitude to Michael Anenburg, Gregor Partington and two anonymous reviewers for their excellent insights and comments that significantly improved the quality of this paper and the handling editor, Solveig Pospiech, for processing this paper. Bureau Gravimétrique International (BGI)/International Association of Geodesy is acknowledged for distributing the satellite gravity data.
This paper was edited by Solveig Pospiech and reviewed by Gregor Partington and two anonymous referees.
Aitken, A. R. A., Joly, A., Dentith, M. C., Johnson, S. P., Thorne, A. M., and Tyler, I. M.: 3D architecture, structural evolution, and mineral prospectivity of the Gascoyne Province, Western Australia, Rep. Geol. Surv. West. Aust., 123, 2014.
Allegre, C. J., Birck, J. L., Capmas, F., and Courtillot, V.: Age of the Deccan traps using 187Re–187Os systematics, Earth Planet. Sc. Lett., 170, 197–204, 1999.
Amante, C. and Eakins, B. W.: ETOPO1: 1 arc−minute global relief model: procedures, data sources and analysis, NOAA Tech. Mem. NESDIS NGDC24, NOAA, National Geophysical Data Center, Boulder (Co), 2009.
Andersen, A. K., Clark, J. G., Larson, P. B., and Donovan, J. J.: REE fractionation, mineral speciation, and supergene enrichment of the Bear Lodge carbonatites, Wyoming, USA, Ore Geol. Rev., 89, 780–807, 2017.
Anenburg, M. and Mavrogenes, J. A.: Carbonatitic versus hydrothermal origin for fluorapatite REE-Th deposits: Experimental study of REE transport and crustal “antiskarn” metasomatism, Am. J. Sci., 318, 335–366, 2018.
Anenburg, M., Mavrogenes, J. A., Frigo, C., and Wall, F.: Rare earth element mobility in and around carbonatites controlled by sodium, potassium, and silica, Sci. Adv., 6, eabb6570, https://doi.org/10.1126/sciadv.abb6570, 2020.
Aranha, M., Porwal, A., and González-Álvarez, I.: Targeting REE deposits associated with carbonatite and alkaline complexes in northeast India, Ore Geol. Rev., in review, 2022.
Basu, A. R., Renne, P. R., DasGupta, D. K., Teichmann, F., and Poreda, R. J.: Early and late alkali igneous pulses and a high–3He plume origin for the Deccan flood basalts, Science, 261, 902–906, 1993.
Bell, K. and Simonetti, A.: Source of parental melts to carbonatites–critical isotopic constraints, Miner. Petrol., 98, 77–89, 2010.
Bell, K. and Tilton, G. R.: Probing the mantle: the story from carbonatites, Eos T. Am. Geophys. Un., 83, 273–277, 2002.
Bertrand, G., Billa, M., Cassard, D., Tourlière, B., Angel, J. M., and Tertre, F.: A new method to assess favorability of critical by-product commodities: application to rare earth elements in Europe, in: Mineral Prospectivity, current approaches and future innovations – Orléans, France, The Mineral Prospectivity, current approaches and future innovations conference, 24–26 October 2017, p. 17, 2017.
Bhushan, S. K.: Geology of the Kamthai rare earth deposit, J. Geol. Soc. India, 85, 537–546, 2015.
Bhushan, S. K. and Kumar, A.: First carbonatite hosted REE deposit from India, J. Geol. Soc. India, 81, 41–60, 2013.
Bhushan, S. K. and Mohanty, M.: Mechanics of intrusion and geochemistry of alkaline granites from Siwana, Barmer district, Rajasthan, Indian Journal of Earth Sciences, 15, 103–115, 1988.
Bhushan, S. K. and Somani, O. P.: Rare earth elements and yttrium potentials of Neoproterozoic peralkaline Siwana granite of Malani igneous suite, Barmer district, Rajasthan, J. Geol. Soc. India, 94, 35–41, 2019.
Binnemans, K., Jones, P. T., Blanpain, B., Van Gerven, T., Yang, Y., Walton, A., and Buchert, M.: Recycling of rare earths: a critical review, J Clean. Prod., 51, 1–22, 2013.
Bladon, A. J., Burley, S. D., Clarke, S. M., and Beaumont, H.: Geology and regional significance of the Sarnoo Hills, eastern rift margin of the Barmer Basin, NW India, Basin Res., 27, 636–655, 2015a.
Bladon, A. J., Clarke, S. M., and Burley, S. D.: Complex rift geometries resulting from inheritance of pre–existing structures: Insights and regional implications from the Barmer Basin rift, J. Struct. Geol., 71, 136–154, 2015b.
Boesche, N. K., Rogass, C., Lubitz, C., Brell, M., Herrmann, S., Mielke, C., Tonn, S., Appelt, O., Altenberger, U., and Kaufmann, H.: Hyperspectral REE (rare earth element) mapping of outcrops–applications for neodymium detection, Remote Sens.-Basel, 7, 5160–5186, 2015.
Bonham-Carter, G. F.: Geographic information systems for geoscientists-modelling with GIS, Computer Methods in the Geoscientists, 13, p.398, 1994.
Bonvalot, S., Balmino, G., Briais, A., Kuhn, M., Peyrefitte, A., Vales, N., Biancale, R., Gabalda, G., Reinquin, F., and Sarrailh, M.: World Gravity Map, Commission for the Geological Map of the World, edited by: BGI-CGMW-CNES-IRD, Paris, 2012.
Castellano, G., Castiello, C., Pasquadibisceglie, V., and Zaza, G.: FISDeT: Fuzzy inference system development tool, Int. J. Comput. Int. Sys., 10, 13–22, 2017.
Castor, S. B.: The Mountain Pass rare-earth carbonatite and associated ultrapotassic rocks, California, Can. Mineral., 46, 779–806, 2008.
Chakhmouradian, A. R. and Wall, F.: Rare earth elements: minerals, mines, magnets (and more), Elements, 8, 333–340, 2012.
Chakhmouradian, A. R., Reguir, E. P., Kressall, R. D., Crozier, J., Pisiak, L. K., Sidhu, R., and Yang, P.: Carbonatite-hosted niobium deposit at Aley, northern British Columbia (Canada): Mineralogy, geochemistry and petrogenesis, Ore Geol. Rev., 64, 642–666, 2015.
Chandra, J., Paul, D., Viladkar, S. G., and Sensarma, S.: Origin of the Amba Dongar carbonatite complex, India and its possible linkage with the Deccan Large Igneous Province, Geological Society, London, Special Publications, 463, 137–169, 2018.
Chandrasekaran, V.: Geochemistry of the basic, acid and alkaline intrusives and extrusives of Sarnu-Dandali area, District Barmer, Rajasthan, Unpublished Ph.D. Thesis, University of Rajasthan, Jaipur, India, 108, 1987.
Chandrasekaran, V. and Chawade, M. P.: Carbonatites of Barmer district, Rajasthan, Indian Minerals, 44, 315–324, 1990.
Chandrasekaran, V., Srivastava, R. K., and Chawade, M. P.: Geochemistry of the alkaline rocks of Sarnu-Dandali area, district Barmer, Rajasthan, India, Journal of Geological Society of India (Online archive from Vol 1 to Vol 78), 36, 365–382, 1990.
Chenet, A. L., Quidelleur, X., Fluteau, F., Courtillot, V., and Bajpai, S.: 40K–40Ar dating of the Main Deccan large igneous province: Further evidence of KTB age and short duration, Earth Planet. Sc. Lett., 263, 1–15, 2007.
Chudasama, B., Porwal, A., Kreuzer, O. P., and Butera, K.: Geology, geodynamics and orogenic gold prospectivity modelling of the Paleoproterozoic Kumasi Basin, Ghana, West Africa, Ore Geol. Rev., 78, 692–711, 2016.
Chudasama, B., Lisitsin, V. A., and Porwal, A. K.: Uncertainties in prospectivity analysis of surficial uranium mineral systems in Western Australia, in: Mineral Prospectivity, current approaches and future innovations – Orléans, France, The Mineral Prospectivity, current approaches and future innovations conference, 24–26 October 2017, p. 24, 2017.
Collier, J. S., Sansom, V., Ishizuka, O., Taylor, R. N., Minshull, T. A., and Whitmarsh, R. B.: Age of Seychelles–India break–up, Earth Planet. Sc. Lett., 272, 264–277, 2008.
Cordeiro, P. F., Brod, J. A., Dantas, E. L., and Barbosa, E. S.: Mineral chemistry, isotope geochemistry and petrogenesis of niobium-rich rocks from the Catalão I carbonatite-phoscorite complex, Central Brazil, Lithos, 118, 223–237, 2010.
Devey, C. W. and Stephens, W. E.: Deccan–related magmatism west of the Seychelles–India rift, in: Magmatism and the Causes of Continental Break–up, edited by: Storey B. C., Alabaster T., Pankhurst R. J., Geological Society, London, Special Publications, 68, 271–291, 1992.
Dolson, J., Burley, S. D., Sunder, V. R., Kothari, V., Naidu, B., Whiteley, N. P., Farrimond, P., Taylor, A., Direen, N., and Ananthakrishnan, B.: The discovery of the Barmer Basin, Rajasthan, India, and its petroleum geology, The Association of American Petroleum Geologists Bulletin, 99, 433–465, 2015.
Duda, R., Gaschning, J., and Hart, P.: Model design in the prospector consultant system for mineral exploration, in: Expert Systems, In the Microelectronic Age, edited by: Michie, D., Edinburgh University Press, Edinburgh, 153–167, 1979.
Duda, R. O., Hart, P. E., Barrett, P., Gasching, J. G., Konolige, K., Reboh, R., and Slocum, J.: Development of the prospector consultation system for mineral exploration, Final Report, SRI Projects 5821 and 6415, Artificial Intelligence Center, SRI International, Menlo Park, p. 193, 1978.
Duda, R. O., Nilsson, N. J., and Raphael, B.: State of technology in artificial intelligence, in: Research Directions in Software Technology, edited by: Wegner, P., MIT, Cambridge, Mass, 729–749, 1980.
Duke, G. I.: Black Hills–Alberta carbonatite–kimberlite linear trend: Slab edge at depth?, Tectonophysics, 464, 186–194, 2009.
Duke, G. I., Carlson, R. W., Frost, C. D., Hearn Jr, B. C., and Eby, G. N.: Continent-scale linearity of kimberlite–carbonatite magmatism, mid-continent North America, Earth Planet. Sc. Lett., 403, 1–14, 2014.
Ekmann, J. M.: Rare earth elements in coal deposits–a prospectivity analysis, in: Adapted from poster presentations AAPG Easter Section meeting, Cleveland, Ohio, The AAPG Eastern Section meeting, 22–26 September 2012, 22–26, 2012.
Elliott, H. A. L., Wall, F., Chakhmouradian, A. R., Siegfried, P. R., Dahlgren, S., Weatherley, S., Finch, A. A., Marks, M. A. W., Dowman, E., and Deady, E.: Fenites associated with carbonatite complexes: A review, Ore Geol. Rev., 93, 38–59, 2018.
Ernst, R. E. and Bell, K.: Large igneous provinces (LIPs) and carbonatites, Miner. Petrol., 98, 55–76, 2010.
Ganerød, M., Torsvik, T. H., Van Hinsbergen, D. J. J., Gaina, C., Corfu, F., Werner, S., Owen–Smith, T. M., Ashwal, L. D., Webb, S. J., and Hendriks, B. W. H.: Palaeoposition of the Seychelles microcontinent in relation to the Deccan Traps and the Plume Generation Zone in Late Cretaceous–Early Palaeogene time, in: The formation and evolution of Africa: a synopsis of 3.8 Ga of Earth history, edited by: Van Hinsbergen, D. J. J., Buiter, S. J. H., Torsvik, T. H., Gaina, C., and Webb, S. J., Geological Society, London, Special Publications, 357, 229–252, 2011.
Garson, M. S. and Smith, W. C.: Chilwa Island, Nyasaland [Malawi]: Nyasaland Geological Survey Department, memoir 1, 1958.
Giovannini, A. L., Neto, A. C. B., Porto, C. G., Pereira, V. P., Takehara, L., Barbanson, L., and Bastos, P. H.: Mineralogy and geochemistry of laterites from the Morro dos Seis Lagos Nb (Ti, REE) deposit (Amazonas, Brazil), Ore Geol. Rev., 88, 461–480, 2017.
Gönenç, T.: Investigation of distribution of embedded shallow structures using the first order vertical derivative of gravity data, J. Appl. Geophys., 104, 44–57, 2014.
González-Álvarez, I., Porwal, A., Beresford, S. W., McCuaig, T. C., and Maier, W. D.: Hydrothermal Ni prospectivity analysis of Tasmania, Australia, Ore Geol. Rev., 38, 168–183, 2010.
González-Álvarez, I., Stoppa, F., Yang, X. Y., and Porwal, A.: Introduction to the Special Issue, Insights on Carbonatites and their Mineral Exploration approach: A Challenge towards Resourcing Critical Metals, Ore Geol. Rev. Special Issue, 133, 104073, https://doi.org/10.1016/j.oregeorev.2021.104073, 2021.
Goodenough, K. M., Schilling, J., Jonsson, E., Kalvig, P., Charles, N., Tuduri, J., Deady, E. A., Sadeghi, M., Schiellerup, H., Müller, A., and Bertrand, G.: Europe's rare earth element resource potential: An overview of REE metallogenetic provinces and their geodynamic setting, Ore Geol. Rev., 72, 838–856, 2016.
Goodenough, K. M., Wall, F., and Merriman, D.: The rare earth elements: demand, global resources, and challenges for resourcing future generations, Natural Resources Research, 27, 201–216, 2018.
GSI and AMD: Strategic Plan for Enhancing REE Exploration in India, Geological Survey of India and Atomic Mineral Directorate for Exploration and Research, https://employee.gsi.gov.in/cs/groups/public/documents/document/b3zp/oda5/~edisp/dcport1gsigovi809087.pdf or https://www.amd.gov.in/WriteReadData/userfiles/file/GSI_AMD_Vision_Document_REE.pdf, 2020.
Gunn, P. J. and Dentith, M. C.: Magnetic responses associated with mineral deposits, AGSO Journal of Australian Geology and Geophysics, 17, 145–158, 1997.
Holden, E. J., Fu, S. C., Kovesi, P., Dentith, M., Bourne, B., and Hope, M.: Automatic identification of responses from porphyry intrusive systems within magnetic data using image analysis, J. Appl. Geophys., 74, 255–262, 2011.
Indian Bureau of Mines: Indian Minerals Yearbook 2018 (Part- III: Mineral Reviews), Rare Earths, 57, 24.1–24.7, https://ibm.gov.in/writereaddata/files/02182020103349Rare%20Earths_2018.pdf (last access: 5 March 2022), 2018.
Indian Bureau of Mines: Indian Minerals Yearbook 2019 (Part- III: Mineral Reviews), Rare Earths, 58, 24.1–24.10, https://ibm.gov.in/writereaddata/files/10012020172151RareEarth_2019_AR.pdf (last access: 5 March 2022), 2019.
International Union of Pure and Applied Chemistry (IUPAC): Nomenclature of Inorganic Chemicals – IUPAC Recommendations 2005, RSC Publishing, Cambridge, UK , pp. 377, ISBN 0 85404 438 8, 2005.
Jacobsen, B. H.: A case for upward continuation as a standard separation filter for potential-field maps, Geophysics, 52, 1138–1148, 1987.
Jaireth, S., Hoatson, D. M., and Miezitis, Y.: Geological setting and resources of the major rare-earth-element deposits in Australia, Ore Geol. Rev., 62, 72–128, 2014.
Johnson, N. L., Kotz, S., and Balakrishnan, N.: Chapter 25: Beta Distributions, in: Continuous univariate distributions, volume 2 (Vol. 289), John Wiley & sons, ISBN 978-0-471-58494-0, 1995.
Joly, A., Porwal, A., and McCuaig, T. C.: Exploration targeting for orogenic gold deposits in the Granites–Tanami Orogen: Mineral system analysis, targeting model and prospectivity analysis, Ore Geol. Rev., 48, 349–383, 2012.
Jones, A. P., Genge, M., and Carmody, L.: Carbonate melts and carbonatites, Rev. Mineral. Geochem., 75, 289–322, 2013.
Jones, R. M. and Hillis, R. R.: An integrated, quantitative approach to assessing fault-seal risk, AAPG Bull., 87, 507–524, 2003.
Keating, P. and Sailhac, P.: Use of the analytic signal to identify magnetic anomalies due to kimberlite pipes, Geophysics, 69, 180–190, 2004.
Kozlov, E., Fomina, E., Sidorov, M., Shilovskikh, V., Bocharov, V., Chernyavsky, A., and Huber, M.: The Petyayan-Vara carbonatite-hosted rare earth deposit (Vuoriyarvi, NW Russia), Mineral. Geochem. Mineral., 10, p. 73, 2020.
Kreuzer, O. P., Etheridge, M. A., Guj, P., McMahon, M. E., and Holden, D. J.: Linking mineral deposit models to quantitative risk analysis and decision-making in exploration, Econ. Geol., 103, 829–850, 2008.
Latham, A. G., Harding, K. L., Lapointe, P., Morris, W. A., and Balch, S. J.: On the lognormal distribution of oxides in igneous rocks, using magnetic susceptibility as a proxy for oxide mineral concentration, Geophys. J. Int., 96, 179–184, 1989.
Laznicka, P.: Giant metallic deposits: Future sources of industrial metals, Springer Science & Business Media, Springer-Verlag Berlin Heidelberg, ISBN 978-3-642-12405-1, 2006.
Le Bas, M. J.: Fenites associated with carbonatites, Can. Mineral., 46, 915–932, 2008.
Lisitsin, V. A., Porwal, A., and McCuaig, T. C.: Probabilistic fuzzy logic modelling: quantifying uncertainty of mineral prospectivity models using Monte Carlo simulations, Math. Geosci., 46, 747–769, 2014.
Mariano, A. N.: Economic Geology of Rare Earth Elements, Rev. Mineral., 21, 309–337, 1989.
McCuaig, T. C. and Hronsky, J. M.: The mineral system concept: the key to exploration targeting, Soc. Eco. Geo. Spc. Pub., 18, 153–175, 2014.
McCuaig, T. C., Porwal, A., and Gessner, K.: Fooling ourselves: recognizing uncertainty and bias in exploration targeting, Centre for Exploration Targeting Quarterly News, The University of Western Australia, Crawley Western Australia, 2, p. 1, 2009.
Mitchell, R. H.: Primary and secondary niobium mineral deposits associated with carbonatites, Ore Geol. Rev., 64, 626–641, 2015.
Morgenstern, R., Turnbull, R. E., Hill, M. P., Durance, P. M. J., and Rattenbury, M. S.: Rare Earth Element Mineral Potential in New Zealand, GNS Science Consultancy Report, 23, GNS Science, Lower Hutt (NZ), 2018.
Neave, D. A., Black, M., Riley, T. R., Gibson, S. A., Ferrier, G., Wall, F., and Broom-Fendley, S.: On the feasibility of imaging carbonatite-hosted rare earth element deposits using remote sensing, Econ. Geol., 111, 641–665, 2016.
Pande, K., Cucciniello, C., Sheth, H., Vijayan, A., Sharma, K. K., Purohit, R., Jagadeesan, K. C., and Shinde, S.: Polychronous (Early Cretaceous to Palaeogene) emplacement of the Mundwara alkaline complex, Rajasthan, India: 40Ar–39Ar geochronology, petrochemistry and geodynamics, Int. J. Earth Sci., 106, 1487–1504, 2017.
Pawlowski, R. S.: Preferential continuation for potential-field anomaly enhancement, Geophysics, 60, 390–398, 1995.
Pirajno, F.: Intracontinental anorogenic alkaline magmatism and carbonatites, associated mineral systems and the mantle plume connection, Gondwana Res., 27, 1181–1216, 2015.
Poletti, J. E., Cottle, J. M., Hagen-Peter, G. A., and Lackey, J. S.: Petrochronological constraints on the origin of the Mountain Pass ultrapotassic and carbonatite intrusive suite, California, J. Petrol., 57, 1555–1598, 2016.
Porwal, A. and Carranza, E. J. M.: Introduction to the Special Issue: GIS-based mineral potential modelling and geological data analyses for mineral exploration, Ore Geol. Rev., 71, 477–483, 2015.
Porwal, A., Carranza, E. J. M., and Hale, M.: Knowledge-driven and data-driven fuzzy models for predictive mineral potential mapping, Natural Resources Research, 12, 1–25, 2003.
Porwal, A., González-Álvarez, I., Markwitz, V., McCuaig, T. C., and Mamuse, A.: Weights-of-evidence and logistic regression modelling of magmatic nickel sulfide prospectivity in the Yilgarn Craton, Western Australia, Ore Geol. Rev., 38, 184–196, 2010.
Porwal, A., Das, R. D., Chaudhary, B., González-Álvarez, I., and Kreuzer, O.: Fuzzy inference systems for prospectivity modelling of mineral systems and a case-study for prospectivity mapping of surficial Uranium in Yeelirrie Area, Western Australia, Ore Geol. Rev., 71, 839–852, 2015.
Porwal, A. K.: Mineral potential mapping with mathematical geological models (Vol. 130), ITC PhD dissertation, Utrecht University, Enschede, the Netherlands, ISBN 90-6164-240-X, 2006.
Porwal, A. K. and Kreuzer, O. P.: Introduction to the special issue: mineral prospectivity analysis and quantitative resource estimation, Ore Geol. Rev., 38, 121–127, 2010.
Rajagopalan, S.: Analytic signal vs. reduction to pole: solutions for low magnetic latitudes, Explor. Geophys., 34, 257–262, 2003.
Ramakrishnan, M. and Vaidyanadhan, R.: Geology of India (Vol. 1), Geological society of India, Bangalore, 556, 2008.
Ray, J. S. and Pande, K.: Carbonatite alkaline magmatism associated with continental flood basalts at stratigraphic boundaries: cause for mass extinctions, Geophys. Res. Lett., 26, 1917–1920, 1999.
Ray, J. S. and Ramesh, R.: Evolution of carbonatite complexes of the Deccan flood basalt province: stable carbon and oxygen isotopic constraints, J. Geophys. Res.-Sol. Ea., 104, 29471–29483, 1999.
Ray, J. S., Ramesh, R., Pande, K., Trivedi, J. R., Shukla, P. N., and Patel, P. P.: Isotope and rare earth element chemistry of carbonatite–alkaline complexes of Deccan volcanic province: implications to magmatic and alteration processes, J. Asian Earth Sci., 18, 177–194, 2000.
Reddi, A. G. B. and Ramakrishna, T. S.: Bouguer gravity atlas of western Indian (Rajasthan–Gujarat) shield, Geological Survey of India, New Delhi, India, 1988.
Roy, A. B. and Jakhar, S.: Geology of Rajasthan (North West India) Precambrian to Recent, Scientific Publishers, Jodhpur, India, pp. 421, 2002.
Sadeghi, M.: Regional-scale prospectivity mapping on REE mineralization in Bergslagen district, Sweden, in: Mineral Prospectivity, current approaches and future innovations – Orléans, France, The Mineral Prospectivity, current approaches and future innovations conference, 24–26 October 2017, p. 61, 2017.
Sen, G., Hames, W. E., Paul, D. K., Biswas, S. K., Ray, A., and Sen, I. S.: Pre–Deccan and Deccan magmatism in Kutch, India: implications of new 40Ar/39Ar ages of intrusions, Special Publication, J. Geol. Soc. India, 6, 211–222, 2016.
Sethna, S. F. and D'Sa, C. P.: Occurrence of ijolite with veinlets of carbonatite in the Deccan Trap at Murud–Janjira, Maharashtra, India, J. Geol. Soc. India, 37, 257–263, 1991.
Sheth, H., Pande, K., Vijayan, A., Sharma, K. K., and Cucciniello, C.: Recurrent Early Cretaceous, Indo–Madagascar (89–86 Ma) and Deccan (66 Ma) alkaline magmatism in the Sarnu-Dandali complex, Rajasthan: 40Ar–39Ar age evidence and geodynamic significance, Lithos, 284, 512–524, 2017.
Simandl, G. J. and Paradis, S.: Carbonatites: related ore deposits, resources, footprint, and exploration methods, Applied Earth Science, 127, 123–152, 2018.
Simonetti, A., Bell, K., and Viladkar, S. G.: Isotopic data from the Amba Dongar carbonatite complex, west–central India: evidence for an enriched mantle source, Chemical Geology, 122, 185–198, 1995.
Simonetti, A., Goldstein, S. L., Schmidberger, S. S., and Viladkar, S. G.: Geochemical and Nd, Pb, and Sr isotope data from Deccan alkaline complexes–inferences for mantle sources and plume–lithosphere interaction, J. Petrol., 39, 1847–1864, 1998.
Singh, P. K., Rajak, P. K., Singh, M. P., Singh, V. K., Naik, A. S., and Singh, A. K.: Peat swamps at Giral lignite field of Barmer basin, Rajasthan, Western India: understanding the evolution through petrological modelling, International Journal of Coal Science & Technology, 3, 148–164, 2016.
Skirrow, R. G., Huston, D. L., Mernagh, T. P., Thorne, J. P., Duffer, H., and Senior, A.: Critical commodities for a high–tech world: Australia's potential to supply global demand, Geoscience Australia, Canberra, 2013.
Spandler, C., Slezak, P., and Nazari-Dehkordi, T.: Tectonic significance of Australian rare earth element deposits, Earth-Sci. Rev., 207, p. 103219, 2020.
Stoppa, F., Pirajno, F., Schiazza, M., and Vladykin, N. V.: State of the art: Italian carbonatites and their potential for critical-metal deposits, Gondwana Res., 37, 152–171, 2016.
Thomas, M. D., Ford, K. L., and Keating, P.: Review paper: Exploration geophysics for intrusion-hosted rare metals, Geophys. Prospect., 64, 1275–1304, 2016.
U.S. Geological Survey: Mineral commodity summaries 2021, U.S. Geological Survey, 200 p., https://doi.org/10.3133/mcs2021, 2021.
Van Gosen, B. S., Verplanck, P. L., Seal, R. R., II, Long, K. R., and Gambogi, J.: Rare-earth elements, chap. O (No. 1802-O), in: Critical mineral resources of the United States–Economic and environmental geology and prospects for future supply, edited by: Schulz, K. J., DeYoung Jr., J. H., Seal II, R. R., and Bradley, D. C., U.S. Geological Survey Professional Paper 1802p, Reston, VA, O1–O31, https://doi.org/10.3133/pp1802O, 2017.
Verplanck, P. L. and Hitzman, M.: Rare earth and critical elements in ore deposits (Vol. Reviews in Economic Geology 18), Littleton, Society of Economic Geologists, Colorado, 2016.
Verplanck, P. L. and Van Gosen, B. S.: Carbonatite and alkaline intrusion–related rare earth element deposits–a deposit model (No. 2011–1256), US Geological Survey, Reston, Virginia, 2011.
Vijayan, A., Sheth, H., and Sharma, K. K.: Tectonic significance of dykes in the Sarnu-Dandali alkaline complex, Rajasthan, northwestern Deccan Traps, Geosci. Front. 7, 783–791, 2016.
Wall, F.: Rare earth elements, in: Critical metals handbook, edited by: Gunn, A. G., Wiley, London, 312–339, 2014.
Wall, F.: Rare earth elements, Encyclopedia of Geology, second edn., 680–693, https://doi.org/10.1016/B978-0-08-102908-4.00101-6, Academic Press, 2021.
Woolley, A. R. and Bailey, D. K.: The crucial role of lithospheric structure in the generation and release of carbonatites: geological evidence, Mineral. Mag., 76, 259–270, 2012.
Woolley, A. R. and Kjarsgaard, B. A.: Carbonatite occurrences of the world: map and database, Open File 5796, Geological Survey of Canada, Ottawa, Ontario, 2008a.
Woolley, A. R. and Kjarsgaard, B. A.: Paragenetic types of carbonatite as indicated by the diversity and relative abundances of associated silicate rocks: evidence from a global database, Can. Mineral., 46, 741–752, 2008b.
Zheng, Y. F.: Metamorphic chemical geodynamics in continental subduction zones, Chem. Geol., 328, 5–48, 2012.
Zheng, Y. F.: Subduction zone geochemistry, Geosci. Front., 10, 1223–1254, 2019.
Zimmermann, R., Brandmeier, M., Andreani, L., Mhopjeni, K., and Gloaguen, R.: Remote sensing exploration of Nb-Ta-LREE-enriched carbonatite (Epembe/Namibia), Remote Sens.-Basel, 8, p. 620, 2016.
- Abstract
- Introduction
- Geological setting of the study area
- Datasets and methodology pipeline
- Mineral systems model for carbonatite–alkaline complex related REE deposits
- Targeting criteria and predictor maps
- FIS-based prospectivity modelling
- Results and discussion
- Summary, conclusions and recommendations
- Appendix A
- Data availability
- Author contributions
- Competing interests
- Disclaimer
- Special issue statement
- Acknowledgements
- Review statement
- References
- Abstract
- Introduction
- Geological setting of the study area
- Datasets and methodology pipeline
- Mineral systems model for carbonatite–alkaline complex related REE deposits
- Targeting criteria and predictor maps
- FIS-based prospectivity modelling
- Results and discussion
- Summary, conclusions and recommendations
- Appendix A
- Data availability
- Author contributions
- Competing interests
- Disclaimer
- Special issue statement
- Acknowledgements
- Review statement
- References