the Creative Commons Attribution 4.0 License.
the Creative Commons Attribution 4.0 License.
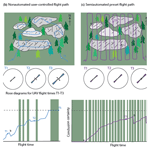
How can geologic decision-making under uncertainty be improved?
Cristina G. Wilson
Clare E. Bond
Thomas F. Shipley
In the geosciences, recent attention has been paid to the influence of uncertainty on expert decision-making. When making decisions under conditions of uncertainty, people tend to employ heuristics (rules of thumb) based on experience, relying on their prior knowledge and beliefs to intuitively guide choice. Over 50 years of decision-making research in cognitive psychology demonstrates that heuristics can lead to less-than-optimal decisions, collectively referred to as biases. For example, the availability bias occurs when people make judgments based on what is most dominant or accessible in memory; geoscientists who have spent the past several months studying strike-slip faults will have this terrain most readily available in their mind when interpreting new seismic data. Given the important social and commercial implications of many geoscience decisions, there is a need to develop effective interventions for removing or mitigating decision bias.
In this paper, we outline the key insights from decision-making research about how to reduce bias and review the literature on debiasing strategies. First, we define an optimal decision, since improving decision-making requires having a standard to work towards. Next, we discuss the cognitive mechanisms underlying decision biases and describe three biases that have been shown to influence geoscientists' decision-making (availability bias, framing bias, anchoring bias). Finally, we review existing debiasing strategies that have applicability in the geosciences, with special attention given to strategies that make use of information technology and artificial intelligence (AI). We present two case studies illustrating different applications of intelligent systems for the debiasing of geoscientific decision-making, wherein debiased decision-making is an emergent property of the coordinated and integrated processing of human–AI collaborative teams.
- Article
(1009 KB) - Full-text XML
- BibTeX
- EndNote
Evidently, if the investigator is to succeed in the discovery of veritable explanations of phenomena, he must be fertile in the invention of hypotheses and ingenious in the application of tests. The practical questions for the teacher are, whether it is possible by training to improve the guessing faculty, and if so, how it is to be done. To answer these, we must give attention to the nature of the scientific guess considered as a mental process. Like other mental processes, the framing of hypotheses is usually unconscious, but by attention it can be brought into consciousness and analyzed.
When Grove Karl Gilbert wrote about the development of a “guessing faculty” in The Inculcation of Scientific Method by Example (1886), he was one of the first to highlight the value of understanding how geoscientists resolve epistemic uncertainty during judgment and decision-making. Epistemic uncertainty refers to knowledge that an individual, in principle, could have but does not, i.e., limited information of the environment or system of study. Although epistemic uncertainty is a feature of all sciences, in the geosciences it is the standard rather than the special case (Bárdossy and Fodor, 2001; Frodeman, 1995). Geoscientists must frequently make decisions when data are incomplete, e.g., when the isolation of processes can be difficult because multiple processes have cumulatively transformed the rocks, and when direct observation (much less experimental control) is impossible due to the large time spans of geologic processes, which leaves evidence lost or buried beneath the Earth’s surface.
To understand the influence of uncertainty on decision-making in the geosciences, and what the human mind adds to the problem of inducing rules from incomplete cases, recent research has followed Gilbert's advice and studied geologic uncertainty as a mental process through the lens of cognitive science. In this work, particular attention has been paid to ways that humans constrain judgment and decision-making through the use of heuristics, i.e., rules of thumb. Heuristics are efficient and offer satisfactory solutions for most decisions, but they can sometimes yield less-than-optimal choices, collectively referred to as human decision biases. Geoscience scholars have begun to characterize the influence of such biases in geologic decision-making (Alcalde et al., 2017a, b; Barclay et al., 2011; Bond et al., 2007; Polson and Curtis, 2010; Rowbotham et al., 2010; Taylor et al., 1997). For example, the interpretation of synthetic seismic images has been shown to be vulnerable to availability bias, which occurs when people make judgments based on what is most dominant or accessible in memory; participants' interpretations were positively related to their primary field of expertise in tectonic settings, i.e., an individual who indicated thrust tectonics as their primary field (and had this setting most accessible in memory) was more likely to interpret the image as thrust faults than an individual with a different expertise (Bond et al., 2007).
Characterizing the impact of decision biases such as the availability bias is important and more work is needed to determine the range of biases influencing geoscientists and their prevalence in geologic decision-making. However, given the potential costs of biased decisions, there is a still greater need to develop effective interventions for removing or mitigating bias: in the words of Gilbert (opening quote), to determine “whether it is possible by training to improve the guessing faculty, and if so, how it is to be done”. The development of debiasing techniques is especially important for geologic decisions that have social and commercial implications (e.g., hazard prediction, resource extraction, waste storage, water supply) but could also benefit the underpinning workflows involved in more commonplace decisions (e.g., navigation, mapping) and result in improved field practice. The cognitive literature on judgment and decision-making offers valuable insights into how susceptibility to decision bias can be reduced and thus how geologic decision-making under uncertainty might be improved. In this paper, we outline the key insights from judgment and decision-making research about how to reduce bias and review the literature on debiasing strategies. In doing so, we seek to highlight the most promising avenues for future research on debiasing geologic decision-making.
The paper is organized as follows. First, we briefly discuss how to define an optimal decision, since “improving” geologic decision-making necessitates having a clear standard to work towards. Next, we describe the origins of decision biases using a dual-process distinction that has been supported by a wealth of research in cognitive science (for review, see Evans and Stanovich, 2013). Dual-process theories of decision-making posit the existence of two unique sets of processes: a set of intuitive and largely automatic processes and a set of deliberative and more effortful processes. We explain how dual-process theories can account for three specific decision biases: the availability bias, framing bias, and anchoring bias. We focus on these three biases because their influence has been well-documented in the geoscience literature. Finally, we analyze existing debiasing strategies that have applicability in the geosciences. We categorize existing strategies based on whether they debias by modifying the decision maker (i.e., provide knowledge or tools that must be self-employed to debias) or debias by modifying the environment (i.e., change settings or the information available in the environment in which decisions occur to debias). Special attention is given to debiasing strategies that make use of information technology and artificial intelligence (AI) when modifying the decision maker or environment. We believe that these technologies offer the opportunity to overcome some of the cognitive constraints that result in biased strategies and thus hold the greatest promise of successful application in the geosciences.
What does it mean to choose optimally during scientific decision-making? The scientific decision process is complex and dynamic, and “optimality” may be defined at various levels from the selection of a measurement tool and sampling site, to the calculation of individual parameters, to interpretation (single or multi-scenario). The position we take in this article is that normative decision models offer a reasonable benchmark for assessing optimal choice in geoscience decisions at all levels. Normative models are based in economic theory and describe how people should make decisions: people should strive to maximize the expected utility of a decision, the probability that an act will lead to an outcome that is preferable to all alternatives (the principle of dominance). Also, people should be internally consistent in their decision-making, meaning they should assign the same utility to decision alternatives regardless of minor changes in context, such as the description and order of alternatives or the presence or absence of other alternatives (the principle of invariance).
Unfortunately, what people should do is not always what they actually do. Numerous behavioral decision studies have demonstrated that how people make decisions in the real world can systematically violate normative models (for review, see Gilovich et al., 2002; Kahneman, 2011). These systematic departures from optimal choice are referred to as biases, and they arise (as described in the Introduction) from reliance on heuristics during decision-making. For example, framing equivalent decision outcomes as positive (e.g., 60 % chance to win) or negative (e.g., 40 % chance to lose) has been shown to systematically alter risk preference, a violation of the invariance principle. This framing bias can drive individuals to make decisions that fail to maximize expected value (e.g., preference for a certain gain over a gamble with a probabilistically higher value), a violation of the dominance principle.
While it is clear from past research that people do not always make decisions as they should, there is good reason to believe that people have the capacity to improve decision-making to the normative standard. This is evidenced by observations that (1) when people actively reflect on normative principles they are likely to endorse them, even if they have violated those norms in the past, and (2) some people already adhere to normative principles in their decision-making, and these individuals often have higher analytic skills (are more reflective and engaged) than those who are vulnerable to bias (for review, see Stanovich and West, 2000). Thus, in the current article we will not focus on the question of whether geoscientists can make optimal choices (we assume this is possible); instead, we will address the question of how to effectively move geoscientist decision-making towards a normative standard (Sect. 4, “Debiasing strategies”). However, first we review in more detail the cognitive mechanisms by which biases arise.
In the opening quote, Gilbert is astute in his observation that the mental processes through which decision makers handle uncertainty are frequently outside the focus of their attention. Converging evidence from psychology and neuroscience suggests there are two distinct sets of processes driving judgment and decision-making (for review, see Evans and Stanovich, 2013). One set of processes is intuitive and places minimal demand on cognitive resources because it does not require controlled attention. The other set is deliberative and places greater demand on cognitive resources but also enables uniquely human abilities such as mental simulation and cognitive decoupling during hypothetical thinking, i.e., the ability to prevent real-world representations from becoming confused with representations of imaginary situations. In the judgment and decision-making literature these sets of processes are typically referred to as Type 1 and Type 2 processes, respectively; however, for the purposes of this paper we will simply refer to them as intuitive and deliberative. Both sets of processes serve important functions when making decisions under uncertainty. Generally, intuitive processes are believed to be prompted rapidly and with minimal effort, providing default responses that serve as heuristics. When the heuristic responses are inappropriate and do not align with set goals, deliberative processes intervene and engage available resources for slower, more reflective reasoning (Evans and Stanovich, 2013; Kahneman and Frederick, 2002).
The interaction between intuitive and deliberative processes can be likened to that of a reporter and an editor in a newspaper room.1 Reporters (i.e., intuitive processes) interpret the world and produce the bulk of the written work (i.e., decision-making). It is the job of the editor (i.e., deliberative processes) to endorse the work of reporters, edit the work, or stop it altogether. Unfortunately, editors are often overburdened, so stories that should be edited or stopped (because they are in some way flawed or objectionable) are instead endorsed. Similarly, deliberative processing is often overworked because it is restricted by the limited capacity of available cognitive resources – so heuristic responses that are ill-suited for the current decision environment can be mistakenly endorsed by deliberative processes.
It is important to note that, on most occasions, heuristic responses do lead to good decisions. Intuitive processing draws from our knowledge of the world, our experiences, and the skills we possess – it is what allows us to move quickly and efficiently through our environment, making decisions with relatively little effort. To truly appreciate the value of intuitive processing, consider how your behavior in the last hour would have changed if you had to deliberately think about every choice: you would have had to deliberate about the best form for sitting down and standing up, the amount of time spent in each position, where to put your pen, which way to position your coffee cup, the best web browser to use, and so on. Viewed in this light, we should all be thankful for the intuitive processes that allow us to make decisions quickly and effortlessly.
Yet, while intuitive processing is generally effective, there are some situations in which the heuristic responses generated by intuitive processes are inappropriate and fail to meet desired goals. In such circumstances, we need intervention from deliberative processes to behave optimally. Decision-making is vulnerable to bias when (1) deliberative processes do not interrupt and override faulty heuristic responses or (2) when the content of deliberative processing is itself flawed. Because deliberative processing is constrained by the capacity of available cognitive resources, in situations in which resources are already limited (e.g., high-mental-effort tasks, fatigue, sleep deprivation) decision makers will be more likely to rely on heuristic responses, thus making them particularly susceptible to bias in such situations. Also, in general, humans act as cognitive misers (Böckenholt, 2012; Stanovich, 2009), meaning even when cognitive resources are available for deliberative processing we tend to rely on less effortful intuitive processes. Thus, broadly speaking, decision-making may be debiased by changing the environment or through training change the decision maker, so default heuristic responses lead to good decisions or so the application of deliberative processing is supported under conditions in which it is otherwise unlikely to be applied (Milkman et al., 2009).
There are three decision biases that have been shown to influence geologic decision-making: the availability bias, the framing bias, and the anchoring bias. All three are driven by faulty heuristic responses, which should be overridden by deliberative processes but are not. A form of anchoring bias can also be driven by flawed deliberative processing, which is discussed. These three biases by no means exhaust the full range of biases that could be influencing geologic decision-making under uncertainty, but they are, at present, the best documented in the geosciences literature. For a more complete list of biases and their potential influence on geologic decision-making, see Baddeley et al. (2004), Bond (2015), and Rowbotham et al. (2010).
3.1 Availability bias
This bias is driven by an availability heuristic (intuitive process), which is a tendency to make decisions based on what is most dominant or accessible in memory. To illustrate, consider the following: do air pollutants have a higher concentration outdoors or indoors? When asked to make this judgment you likely recalled (automatically and without effort) news stories related to outdoor air pollutants; maybe you visualized billowing smoke, car exhaust, or a smoggy city skyline. The ease with which examples of outdoor air pollution were drawn to mind probably led you to conclude that air pollution is more highly concentrated outdoors versus indoors. If so, you have just fallen prey to the availability bias. In fact, of air quality studies examining both indoor and outdoor environments, over two-thirds have found higher pollutant concentrations inside (Chen and Zhao, 2011).
The availability heuristic can lead to bias because it substitutes one question (the size or frequency of a category or event) for another (the accessibility of the category or event in memory). When the ease with which something is drawn to mind is not reflective of the true size or frequency, bias occurs. There are many factors besides frequency that can make it easy to come up with instances in memory: for example, the recency with which something has occurred (e.g., flying is perceived as more risky or dangerous immediately following a plane crash), whether it holds personal significance (e.g., people have better attention and memory for the household tasks they complete, causing them to underestimate the contributions of their living partner), and how salient or dramatic it is (e.g., shark attacks get lots of media attention so people tend to exaggerate their frequency). Note, then, that if a event (a) did not occur recently, (b) does not apply to you, or (c) is banal, it will lead to an impression that the event is rare (even if it is not).
In the geoscience literature, evidence of the availability bias during data interpretation has been documented in both experts (Bond et al., 2007) and students (Alcalde et al., 2017b). Bond et al. (2007) found that experts' interpretations of seismic images were related to their primary field of expertise in tectonic settings; specifically, the most dominant tectonic setting in memory was the one selected. Likewise, Alcalde et al. (2017b) found that geology students were more likely to interpret a fault in a seismic image as normal-planar as this fault type and geometry are overrepresented in teaching materials, particularly those the students had encountered. After students were exposed to a greater range of fault models through a 2-week training course, the range of fault interpretation type and geometry increased. The potential value of such education programs for reducing vulnerability to decision bias is discussed in Box 1: “Can better decision-making be taught?”
3.2 Framing bias
Framing bias occurs when people respond differently to objectively equivalent judgments based on how potential outcomes are described or framed. It is generally believed to be the result of an initial affective reaction, or affect heuristic (intuitive process), that makes certain gains particularly attractive and certain losses particularly aversive (Kahneman and Frederick, 2007). In a now classic example of framing bias, Tversky and Kahneman (1981) showed that when disease outbreak intervention programs were framed in terms of lives saved (i.e., 200 out of 600 people will be saved OR 1∕3 probability 600 people will be saved, 2∕3 probability 0 people will be saved) participants preferred the sure option over the risky option, but when the same programs were framed in terms of lives lost participants preferred the risky option over the sure option.2 This research had a huge impact in the fields of psychology and economics (as of 2018 it has been cited over 17 600 times) because it illustrated that human preference can be the product of problem description and not actual substance. Subsequent research has shown that frame-driven changes in risk preference are robust, occurring across a variety of populations and domains, including experts in medicine (McNeil et al., 1982), law (Garcia-Retamero and Dhami, 2013), finance (Fagley and Miller, 1997), and geoscience (Barclay et al., 2011; Taylor et al., 1997).
Early evidence of framing bias in geologic hazard risk assessment was found by Taylor et al. (1997) across two experiments. In experiment 1, participants of varying levels of expertise (high school student, undergraduate student, professional geoscientist) were asked to make decisions regarding hazardous waste storage, flood protection, and volcano monitoring. These problems were presented in a format similar to the disease outbreak problem by Tversky and Kahneman (1981): for the waste storage problem, the positive frame described the probability of safe storage and the negative frame described the probability of an accidental spill; for the flood protection problem, the positive frame described the probability the protection would succeed and the negative frame described the probability it would fail; and for the volcano monitoring problem, the positive frame described the probability the volcano would remain dormant and the negative frame described the probability of an eruption. Across all scenarios, participants demonstrated evidence of frame-driven changes in risk preference. Importantly, professional geoscientists were just as vulnerable to bias as students, suggesting that even experts (who regularly make decisions that impact public safety) can be swayed by superficial choice descriptions.
In experiment 2 (Taylor et al., 1997), high school student participants completed a variation of the volcano monitoring problem in which they played the role of a volcanologist who must interpret incoming information from three instruments to make ongoing decisions about how many people to evacuate from the area surrounding a volcano. Readings from the three instruments were correlated with probabilities of volcanic activity that were positively framed (i.e., dormant) or negatively framed (i.e., eruption). Participants completed either a paper–pencil version or a computerized version of the task. Again, participants demonstrated frame-driven changes in risk preference, but only in the paper–pencil version; in the computerized version, participants were resistant to framing bias. In a follow-up study by Barclay et al. (2011), the same computerized volcano monitoring problem was used but instrument readings were either presented in text format (as in experiment 2; Taylor et al., 1997) or in a novel graphical format. Barclay et al. (2011) reported similar findings of resistance to framing in the text version but found that presenting instrument readings graphically produced frame-driven changes in risk preference. That presentation mode (paper–pencil, computer) and presentation format (graphical, text-based) have an influence on vulnerability to framing bias demonstrates the complexity of characterizing cognitive biases and the need for evidence-informed practices, which we discuss further in Sect. 4, “Debiasing strategies”.
3.3 Anchoring bias
Anchoring is the result of focusing on the first available value or estimate for an unknown quantity before making a judgment or decision about that quantity. The initial value “anchors” subsequent judgments, so decisions stay close to the value considered. Bias occurs when the anchor is incorrect or arbitrary. Unfortunately, even when decision makers are fully aware that the anchor is a meaningless value, it still has a strong influence on their choice. For example, a study by Englich et al. (2006) asked expert judges to make a hypothetical prison sentence for a shoplifter (in months) after rolling a loaded die that only landed on three or nine. Those judges who rolled a nine gave an average sentence of 8 months, while those who rolled a three gave an average sentence of 5 months.
In science, anchors come in the form of initial hypotheses or interpretations. Vulnerability to bias can make scientists reluctant to accept alternative explanations, even in the face of disconfirming evidence. Ultimately, this can disrupt the evolution of knowledge. In the geosciences, for example, Rankey and Mitchell (2003) demonstrated that experts only made minor changes to their initial interpretations of 3-D seismic data after being given additional quality information that could aid interpretation. One expert (who did not change his interpretation at all) noted, “I did… not want to change any of my picks based on the additional well data – looks like I had it nailed.”.
A particular form of anchoring bias is called herding (Baddeley, 2015). Herding is group-driven behavior in which members' judgments are anchored to those of influential group members. This can be especially detrimental in science because evidence that conflicts with established consensus or opinion can be sidelined, and if the conflicting findings are successfully published, the authors risk being ostracized or punished. There are well-known historical examples: Galileo Galilei was convicted of heresy by the Catholic church for supporting the Copernican theory that the Earth and planets revolve around the sun (Lindberg, 2003); Alfred Wegener's championing of plate tectonics theory was ignored, mocked, and deemed pseudoscience by his peers for over 40 years (Vine, 1977).
Empirical evidence of herding in the geosciences was first demonstrated by Phillips (1999), who showed that experts' probability distributions of corrosion rates for nuclear waste storage containers differed depending on whether they were elicited independently or in groups. Experts made different prior assumptions, resulting in probability distributions that were initially radically different. Inter-expert discussion resulted in some convergence of probability distributions but was also accompanied by an increase in the variance of each independent distribution; i.e., experts increased the spread of their initial distributions to encompass the spread of the consensus distribution. In a similar study, Polson and Curtis (2010) showed that experts' estimated probability distributions for the presence of a fault were vulnerable to herding; the group of experts moved towards a single member's opinion such that the consensus distribution was primarily a reflection of the views of one individual.
As mentioned in Sect. 3, “Origins of decision biases”, anchoring is typically driven by a faulty heuristic response, which should be overridden by deliberative processing but is not. When given an anchor, intuitive processes immediately construct a world in which the anchor is true by activating compatible memories. This, in turn, primes decision makers to notice information that is consistent with the anchor and ignore or discount information this is inconsistent. In the Rankey and Mitchell (2003) study, the participant who “had it nailed” was demonstrating an anchoring bias driven by faulty intuitive processing; the participant was primed to interpret new data as consistent with his initial hypothesis and ignore disconfirming data.
Alternatively, anchoring can be driven by flawed deliberative processing, which occurs when decision makers fail to adequately adjust from the anchor. We know this is a failure of deliberative processing because people are more prone to insufficient adjustment when their mental resources are depleted, e.g., when their attention is loaded or when consuming alcohol (Epley and Gilovich, 2006). Research shows people tend to only adjust estimates to the first plausible value and are generally unwilling to search for more accurate estimates (Epley and Gilovich, 2006). This may explain why participants in the Phillips (1999) study, when given the opportunity to adjust their initial probability distributions following group discussion, primarily increased their distribution range to encompass the spread of the consensus distribution. The participants made the simplest adjustment that was still a plausible reflection of the true distribution.
Cognitive research on heuristics and biases, beginning in the 1970s and continuing today, has demonstrated the pervasive and robust influence of an ever-increasing list of decision biases. In comparison, our understanding of how to debias decision-making is limited. This is in part due to the relatively unappealing nature of debiasing research; “it is more newsworthy to show that something is broken than to show how to fix it” (Larrick, 2004). It is also likely that researchers have been dissuaded from pursuing debiasing research because early studies found biases were generally robust in the face of commonsense corrective measures, including providing feedback or incentives (Camerer and Hogarth, 1999; Fischhoff, 1982), holding people accountable for their decisions (Lerner and Tetlock, 1999), and offering warnings about the possibility of bias (Fischhoff, 1982). While there is a strong need for additional research on debiasing, a number of successful strategies have been discovered (for review, see Larrick, 2004; Milkman et al., 2009; Soll et al., 2016). These existing strategies can be categorized into one of two approaches: (1) debiasing by modifying the decision maker or (2) debiasing by modifying the environment (Soll et al., 2016). In the remaining section we consider the pros and cons of the two approaches and discuss how each can be best used in geoscience education and industry to minimize bias.
4.1 Modifying the decision maker
Debiasing strategies that modify the decision maker provide knowledge and tools that must be self-employed to overcome bias. This includes cognitive strategies to shift the perception of a problem (i.e., consider the opposite), the use of computational models to assist judgment, and education on statistical rules and normative principles (see Box 1). All of the above are likely familiar to geoscience scholars, though perhaps not framed as decision aids. In fact, it can be argued that the cognitive debiasing strategy of “consider the opposite” has been a feature of geoscience for over a century. In his 1890 publication The Method of Multiple Working Hypotheses, Thomas Chrowder Chamberlin advocates that geoscientists should generate multiple plausible alternatives to explain the occurrence of geologic phenomena (essentially a “consider the opposite” strategy). Chamberlin is clear in stating the value of this method in guarding against bias (pp. 756).
The effort is to bring up into view every rational explanation of new phenomena, and to develop every tenable hypothesis respecting their cause and history. The investigator thus becomes the parent of a family of hypotheses: and, by his parental relation to all, he is forbidden to fasten his affections unduly upon any one. In the nature of the case, the danger that springs from affection is counteracted… Having thus neutralized the partialities of his emotional nature, he proceeds with a certain natural and enforced erectness of mental attitude to the investigation.
Cognitive research has supported Chamberlin's assertions that generating multiple alternatives to a decision problem can be an effective debiasing strategy, particularly when bias is driven by a tendency to rely on small and unrepresentative samples of information, as with anchoring bias (e.g., Mussweiler et al., 2000), overconfidence (e.g., Koriat et al., 1980), and hindsight bias (e.g., Sanna and Schwarz, 2006). In modern geoscience practice, as noted by Bond (2015), the method of multiple working hypotheses is not consistent with the culture of science in which advocacy for a single model is rewarded. However, there is recognition of the value of promoting a workflow that increases the consideration of permissible interpretations in the geosciences (Bond et al., 2008; Macrae et al., 2016), as well as other sciences faced with complexity and high uncertainty (Elliot and Brook, 2007). While geoscience educators recognize the centrality of the principle of multiple working hypotheses to geoscience practice, there is, to our knowledge, no accepted pedagogy for supporting practice in the skill.
The debiasing strategy of applying computational models is a more recent fixture of geoscience, and its increased prominence is at least partially owed to the increased availability of user-friendly modeling software. In sustainability and resource management research in particular, many modeling and simulation software tools have been created to aid judgment (for review, see Argent, 2004; Argent and Houghton, 2001; Rizzoli and Young, 1997). Computational models systematize the weights placed on various decision inputs (in lieu of relying on expert experience), enabling better forecasting of outcomes. In situations in which expert experience is critical to predicting outcomes, that experience can become a decision input in the model. Past research has shown that such computational models outperform expert judgment in a variety of domains (Dawes et al., 1989), and even the simplest linear models that equally weight all relevant decision inputs (not taking into account historical data on inputs) can outperform expert judgments (Dawes and Corrigan, 1974). In the geosciences, recent research investigating the impact of model use on human judgment about resource management found that management outcomes were superior when student participants relied on models rather than their own experience (Holden and Ellner, 2016). Also, model use has been shown to improve sustainability policy decisions – in a role-play simulation, model users evidenced better outcomes (e.g., low change in temperature, high access to electricity, and high global economy) than participants who did not use models (Czaika and Selin, 2017).
For decision makers to use computational models or employ cognitive techniques like “consider the opposite” successfully requires (at minimum) that deliberative processing resources be available for suspending and correcting decisions. These debiasing strategies operate by supporting the application of deliberative processing under conditions in which it is likely to not be applied, encouraging decision makers to shift themselves from intuitive to deliberative processing. For example, when decision makers “consider the opposite” it encourages deliberative analysis and suspends reliance on intuitions that distort the representation of information (e.g., intuition to rely on what is most dominant or accessible in memory). Recall from Sect. 3, “Origins of decision biases”, that we have a limited capacity of deliberative resources to draw from; i.e., our “editor” can be overworked. Therefore, these debiasing strategies will have a low probability of success in situations in which deliberative processing resources are reduced (e.g., high-mental-effort tasks, states of fatigue or sleep deprivation). Also, individual differences in thinking style and quantitative ability (i.e., numeracy) impact the effectiveness of deliberative processing debiasing strategies; people who are reflective and have high numeracy are more likely to suspend heuristic responses and invoke deliberative resources to do necessary additional thinking (Frederick, 2005). However, even under ideal conditions, in which deliberative resources are not constrained and the decision maker is prone to reflective thinking, there is no guarantee of debiasing success – and herein lies the problem with self-employed debiasing strategies: they may require too much of the decision maker. Successful implementation requires that the decision maker be able to recognize the need to apply a strategy, have the motivation and the required deliberative resources to do so, select the appropriate strategy, and apply it correctly. A mistake or failure at any step of this process could result in (at best) continued vulnerability to decision bias or (at worse) an increase in bias.
Consider, for example, the application of computational models in sustainability and resource management research. Although there are many modeling and simulation software tools available for forecasting climate outcomes (for review, see Argent, 2004; Argent and Houghton, 2001; Rizzoli and Young, 1997), there is concern amongst geoscience scholars that decision makers are not using computational models as often as expected or correctly (see also Box 1 for a discussion of concerns about the level of quantitative education in the geosciences). Both Edwards et al. (2010) and Oxley et al. (2004) found poor receptivity to and “low uptake” of modeling software tools amongst targeted end users within EU-funded research projects. The authors of these studies argue that low uptake resulted from bad communication between tool developers and end users. Thus, despite computational models being available for use in forecasting climate outcomes, some experts are not sufficiently motivated to apply them or are unconfident in their ability to select the appropriate model and apply it correctly, instead relying on their experience and intuition as a substitute for formal analysis.
Determining methods for facilitating the adoption of self-employed debiasing strategies is a critical issue for debiasing research both generally and in the geosciences. Some of the reluctance to use computational models in the geosciences can be solved by improving the design and user interface of modeling and simulation software. To this end, McIntosh and colleagues have outlined design principles (McIntosh et al., 2005) and best practices (McIntosh et al., 2008) for the development of computer-based models, with the goal of improving the usefulness and usability of modeling tools in the geosciences. However, even with improved tool design, decision makers may continue to resist using computational models and other self-employed debiasing strategies. In the words of debiasing researcher Richard Larrick (2004, pp. 331),
[decision makers] do not want to be told that they have been “doing it wrong” for all these years. They do not want to relinquish control over a decision process. And, perhaps most importantly, they fail to understand the benefits of many debiasing techniques relative to their own abilities, not just because they are overconfident, but because the techniques themselves are alien and complex, and the benefits are noisy, delayed, or small.
In sum, self-employed debiasing strategies carry a high risk of being used inappropriately by decision makers (i.e., used incorrectly or not at all), and for this reason we believe that such strategies alone do not offer the most promise for successful application in the geosciences. Instead, we advocate that debiasing strategies (including “consider the opposite” and using computational models) be supported by modifying the decision environment such that (1) people are “nudged” towards the optimal choice strategy or (2) the environment becomes a good fit for the strategy people naturally apply, thereby relieving decision makers of the impetus for debiasing.
4.2 Modifying the environment
Debiasing techniques that modify the environment alter the settings in which decisions occur. The environment can be modified to make it a better fit for the strategy people naturally apply (e.g., status-quo bias pushes people to stick with a default response option over selecting a new option, so making the default a desirable outcome will maximize decision-making). The environment can also be modified to nudge people towards the optimal choice strategy (e.g., prompts to induce reflection and deliberation). The environment modification approach to debiasing is sometimes referred to as choice architecture, making the individual or entity responsible for organizing the environment in which people make decisions the choice architect (Thaler and Sunstein, 2008). It is the role of the choice architect, as put forward by Thaler and Sunstein (2008) in their popular press book Nudge, to influence people's decision-making such that their well-being (and the well-being of others) is maximized, without restricting the freedom to choose. Importantly, there is no such thing as neutral choice architecture; the way the environment is set up will guide decision-making, regardless of whether the setup was intentional on the part of the architect; e.g., descriptions of risk will be framed in terms of gains or losses, and a wise architect chooses the framing that will maximize well-being.
The advantage of debiasing techniques that modify the environment, over those that modify the decision maker, is that it is the choice architect and not the decision maker who is accountable for debiasing (unless, of course, the architect and the decision maker are the same person). Conscious choice architecture is a naturally deliberative process – potential mechanisms of bias must be considered and used to design nudges, and user responses to these nudges must also be considered, including factors unrelated to the nudge that may influence responses. Therefore, techniques that modify the environment tend to be more successful in reducing vulnerability to bias and improving decision-making, and this has been evidenced in varied domains, e.g., improving rates of organ donation (Johnson and Goldstein, 2003), increasing employee retirement savings (Madrian and Shea, 2001), and encouraging healthier eating habits (Downs et al., 2009).
Choice architecture debiasing techniques have been adopted in the sciences in the form of imposed workflow practices and structured expert elicitation exercises. The latter may be more familiar to geoscientists given that there is a long history of using cumulative expert judgments in geologic research when data are insufficient (e.g., Cooke and McDonald, 1986; Hemming et al., 2018; Wood and Curtis, 2004). Expert elicitation research demonstrates that structured methods can be employed to enforce the consideration of group ideas and opinions such that the vulnerability to overconfidence and other biases is reduced (Wood and Curtis, 2004; Polson and Curtis, 2010). The use of imposed workflows, in comparison, is a newer feature of scientific practice. In the social and life sciences, a research reproducibility crisis has led many academic gatekeepers to advocate for the use of workflows such as study preregistration (i.e., a description of study methods, materials, and analyses published prior to data collection) and open sharing of data and study materials. In geoscience research, the use of workflows is also increasingly encouraged. For example, Gil et al. (2016) propose that the “geoscience paper of the future” should make data and software reusable and explicitly describe higher-level computational workflows.
One way the value of choice architecture debiasing in the sciences manifests is through improved efficiency and effectiveness of an ongoing decision process – how many data points are enough to reach a conclusion about my interpretation(s) and how confident am I in that conclusion? This decision process can be visualized by plotting the relationship between conclusion certainty and data, wherein the term data is generally defined as the quality and quantity of information known. Notably, in most sciences, the amount of data (as defined above) is directly proportional to time, resources, and funds. As data accumulate, conclusion certainty increases until some threshold of confidence is reached, at which point the scientific decision maker makes a conclusion about their interpretation(s). We define this threshold of confidence as a geologist's individual perception of being “certain enough” in their interpretation, and hence the threshold can differ dramatically between individuals (what seems certain to one will not seem certain to all) and be shaped by research context (what counts as “certain enough” in one research field will not count in all research fields). In the ideal case, in which data are homogeneous, collected in an unbiased manner, and consistent with a theory, there is a positive linear relationship between data and conclusion certainty, with greater slopes indicating greater decision efficiency, i.e., faster ascension to the decision maker's threshold of confidence (see Fig. 1). However, as every researcher knows, this ideal case is rare. More often, as data accumulate, researchers experience upward and downward shifts in conclusion certainty. Decision biases can impact how confidence shifts with incoming data and where the threshold of confidence is set.
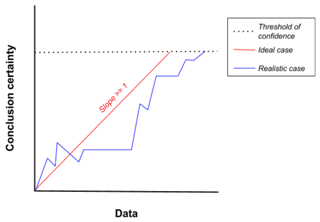
Figure 1The relationship between data and conclusion certainty in the scientific decision-making process. In the ideal case, an increasing number of data are accompanied by increasing certainty, with slopes greater than or equal to one indicating an efficient decision process. In reality, an increasing number of data are more often accompanied by upward and downward shifts in certainty. A scientific conclusion is reached once a decision maker reaches their personal subjective threshold of confidence and feels “certain enough” in their interpretation.
Consider the following study by Macrae et al. (2016) on geologic workflows as an example. Macrae et al. (2016) gave geoscientists a 2-D seismic reflection image and asked them to provide an interpretation within a limited time frame. Half the geoscientist participants were asked to undertake a specific workflow in which they received instruction to explicitly consider geological evolution through writing or drawing (a choice architecture debiasing technique), and the other half received no workflow. The results from Macrae et al. (2016) reveal that geoscientists nudged to consider geological evolution had higher-quality interpretations than those who received no nudge. Because real seismic data were used, the correct interpretation was unknown, but interpretations were deemed high quality if they were consistent with the interpretations of at least one of five leading experts. Some participants may have been overconfident in their interpretation (as suggested by Macrae et al., 2016), which implies that their threshold of confidence was lower, thus increasing the likelihood of accepting an erroneous interpretation. The workflow nudge could have mitigated overconfidence by testing the interpretation (during or after creation) to determine whether the final interpreted geometry could evolve in a geologically reasonable manner. Figure 2 shows the decision process of two hypothetical individuals, Participant 1 and Participant 2. For interpretations in which the evolution was not feasible (Participant 1, Interpretation A), the workflow nudge would force the participant to consider modifications (1B) or alternative interpretations (1C), thereby reducing overconfidence. For interpretations in which the evolution was reasonable, certainty in interpretation would likely increase to the threshold of confidence (Participant 2, Interpretation A).
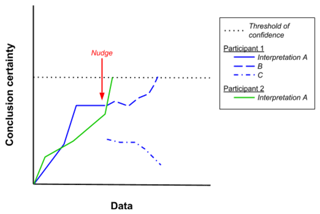
Figure 2The influence of a geologic workflow nudge on conclusion certainty in a study by Macrae et al. (2016). Participants 1 and 2 both experience shifting uncertainty in their initial interpretations as data are accumulated prior to the nudge onset. After being nudged to consider the geological evolution of their interpretations, Participant 2 finds a geologically reasonable evolution for his or her interpretation, certainty in the interpretation increases to their threshold of confidence, and a conclusion is made. Participant 1 cannot construct a geologically reasonable evolution of their initial interpretation, prompting a consideration of modifications (1B) or alternative interpretations (1C).
We can also use the data certainty plot to visualize how the choice architecture practice of expert elicitation influences decision-making and vulnerability to herding (anchoring) in the aforementioned study by Polson and Curtis (2010). Polson and Curtis (2010) asked four expert geoscientists to assess the probability that a fault existed. Figure 3 shows the decision process of the four participants. Note that the x axis of Fig. 3 is represented as time rather than data; as previously stated, we view the quality or quantity of known information (data) as related to time and in this example time is the more coherent descriptor. After making their initial assessment, participants were alerted to common biases in expert judgment and allowed to modify their assessment. If as a result of the warning the participant felt they had made a mistake or their initial probability was somewhat biased, they could have modified their interpretation (Participants 1 and 3) or experienced a reduction in certainty (Participant 4). Alternatively, if the participant perceived their initial analysis to be free of bias – either because it truly was or because the warning was not sufficient for resolving bias – then they would likely stick with their initial probability distribution and potentially experience an increase in conclusion certainty (Participant 2). Following the bias warning and any changes to the initial interpretation, experts shared their probability distributions, explained their reasoning to the group, and were then asked to reach a group consensus.
The results from Polson and Curtis (2010) showed that the consensus interpretation did not reflect the opinions of all participants in the group; instead, the group moved towards one expert's opinion (Participant 1), herding around and anchoring to it (see gray zone, Fig. 3). Thus, although the bias warning may have been an effective debiasing strategy for the individual probabilities at the start of the elicitation, the experimental evidence suggests that it had a minimal impact later in the elicitation process when coming to the group consensus. As discussed in Sect. 4.1, “Modifying the decision maker”, there are many reasons why a simple warning may be ineffective for debiasing – it requires that the interpreter be able to recognize his or her own bias and have the motivation and available cognitive resources to apply a strategy to combat bias – and in group situations in which socially constructed hierarchies exist, bias warnings may be particularly ineffective. A potentially better debiasing strategy in this instance would have been to weight expert opinions and present this information in the form of a digital nudge; e.g., this is what the consensus probability distribution would be if a mean of the experts was calculated and it does not accommodate the range of individual expert opinions. Expert elicitations focusing on geological problems (e.g., Polson and Curtis, 2010; Randle et al., 2019) show that much of their value is in better understanding decision-making workflows and where uncertainties and bias arise, which can be used to inform nudge design.
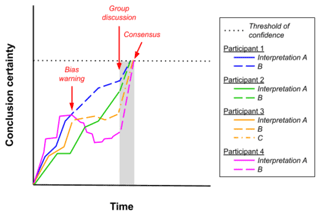
Figure 3The influence of expert elicitation practices on conclusion certainty in a study by Polson and Curtis (2010). All participants experience shifting uncertainty in initial probabilities for the existence of a fault as elicitation progresses. Interpretations A, B, and C refer to each individual’s successive interpretation. When exposed to information on how cognitive bias impacts expert judgment, Participants 1 and 3 modify their interpretations (to 1B and 3B) and Participant 4 experiences a decline in conclusion certainty (4A). Participant 2, in contrast, becomes more certain in his or her initial interpretation following the bias warning, either because the interpretation was truly unbiased or because the warning was insufficient to recognize and resolve bias. When interpretations are shared and discussed amongst the group, Participants 2, 3, and 4 modify their interpretations to be in accordance with Participant 1 – certainty in this new interpretation (2B, 3C, 4B) increases such that a consensus assessment is reached. This herding bias is noted by the gray zone in the figure.
As the above examples illustrate, the potential for choice architecture to aid decision-making in geoscience and other scientific fields is significant. Yet, choice architecture debiasing is not infallible to human error. For example, research on expert elicitation practices in the geosciences has shown that erroneous predictions about geologic events are made when using subjective methods for selecting experts (Shanteau et al., 2002) and when judgments are not aggregated appropriately (Lorenz et al., 2011; Randle et al., 2019). Also, it is worth noting that Macrae et al. (2016) found that over 80 % of participants in the no-workflow group reported that they had considered the geological evolution of their interpretation – these individuals thought they were using a normative workflow, but did not apply it effectively, either because their deliberative processing resources were overburdened from the demands of the interpretation task (“busy editor”) or because of a general tendency towards cognitive miserliness (“lazy editor”). We believe recent innovations in AI and information technology offer the opportunity to overcome the cognitive constraints that lead to both biased choice and trouble in effectively self-employing debiasing strategies. Specifically, we advocate for coordinated and integrated decision-making in humans and intelligent systems, wherein debiased decisions are an emergent property of the human–AI collaborative team. This approach has been referred to as “captology”, standing for “computers as persuasive technology” (Fogg, 2003), and, more recently, “digital nudging” (Mirsch et al., 2017; Weinmann et al., 2016).
This paper is not the first to call attention to the utility of intelligent systems for geoscientific research. The use of intelligent technologies in geoscience research is increasingly common, e.g., mobile robotic platforms (Qian et al., 2017) and machine-learning algorithms (Karpatne et al., 2018), and recent articles in GSA Today (Spino, 2019) and Communications of the ACM (Gil et al., 2018) both outline promising opportunities for intelligent systems to address research challenges in the geosciences. Most of this existing work implicitly takes the view that humans and intelligent systems have separate but complementary functions in geologic decision-making (e.g., Shipley and Tikoff, 2018). Here we present a different view, namely that geologic decision-making can be enhanced when humans and intelligent systems work in collaboration with a shared understanding of the task goal, relevant contextual features, and existing scientific knowledge. To illustrate the value of this digital nudging approach in geoscience research, we discuss two case studies that represent different applications of intelligent systems for the geosciences that are presently in practice: the first case study addresses the use of unmanned aerial vehicles (UAVs or “drones”) to collect new field data, and the second addresses the use of software for geologic interpretation of seismic image data. For each case study, we describe how the intelligent system is currently being used to aid geologic research and how this application of technology has improved, or could improve, upon pretechnological research methods. Then, we describe how digital nudging can be incorporated into intelligent systems and illustrate the scientific value of nudging using the data conclusion certainty plot (similar to Figs. 2 and 3 above).
4.2.1 Case Study 1 – optimizing field data collection with UAVs to minimize anchoring bias
In this case study, we describe how automated unmanned aerial vehicle (UAV) navigation could be used to nudge geoscientists to be more efficient when making decisions regarding reconnaissance and mapping, as well s mitigate against anchoring bias. The advent of better mobile robot platforms has allowed for the deployment of robots by ground, sea, and air to collect field data at a high spatial and temporal resolution. Here, we focus on the use of aerial robots (semiautonomous or autonomous UAVs) for data collection, but the conclusions we draw are likely applicable to other mobile robot platforms (i.e., underwater autonomous vehicles, ground robots).
Currently, the majority of geoscience research with UAVs is nonautonomous, i.e., user controlled. Efforts have been made to automate the interpretation of geological data from UAV imagery or 3-D reconstructions with some success (Thiele et al., 2017; Vasuki et al., 2014, 2017), and the application of image analysis and machine-learning techniques continues to be developed (Zhang et al., 2018). In reconnaissance and geologic mapping, the decision of where to go and how to fly there is made by the expert – either the expert flies the UAV and makes navigation decisions in situ or they preset a flight path for the UAV to follow semiautonomously (e.g., Koparan et al., 2018; Ore et al., 2015). However, a UAV that is capable of attending to measurements in real time and reacting to local features of measurement data could navigate autonomously to collect observations where they are most needed. Such autonomous workflows should increase the efficiency of data collection and could be designed to mitigate against potential biases. Here, we consider how an automated UAV navigation nudge could reduce the tendency to anchor field exploration based on existing models and hypotheses.
In our hypothetical example, a UAV surveys a large bedding surface with the aim of identifying fractures to define the orientations of fracture sets. The bedding surface exposure is large but split into difficult to access exposure, e.g., due to cliff sections or vegetation (see column A, Fig. 4). A bird's-eye view afforded by the UAV improves the ability to observe fractures, which would otherwise require time-costly on-foot reconnaissance to different outcrops of the bedding surface. Note that in our hypothetical example we assume that fracture information is obtained only when the flight path crosses fractures (e.g., column B, blue flight path), thereby representing a high-level reconnaissance rather than a flight path in which overlapping imagery is collected. When the UAV flight path is user controlled, the decision of where and how to fly is unlikely to be optimal: users could be distracted by irrelevant information in UAV view and are likely biased towards exploring certain features and ignoring others (e.g., Andrews et al., 2019). For example, fractures may only be sampled where fracture data are dense or in an orientation that maximizes sample size but not the range in orientation (e.g., Watkins et al., 2015) or when it fits with a hypothesis (e.g., tensional fractures parallel to the axial trace of a fold). These strategies are all informed by expectations, leaving the geoscientist vulnerable to anchoring their sampling behavior to align with initial interpretations and hypotheses.
This anchoring bias is visualized in column B (blue flight path), where the user detects two unique fracture orientations (a, b) on the first exposure visited and then spends the remaining flight looking at fractures in the same orientation rather than searching for new orientations. As a result, a novel N–S fracture orientation is not detected in the user's flight path – the accompanying certainty plot in column B shows that time spent at uninformative exposure (T1 to T2) results in increased certainty that all orientations have been sampled, when in fact they have not (i.e., the threshold of confidence is reached before sampling the N–S orientation). This is reflected in the rose diagrams in column B, which show the orientation of fractures and the relative number of fractures sampled in each orientation; even at time T3 the three fracture sets (as shown in the rose diagram in column A) are not represented.
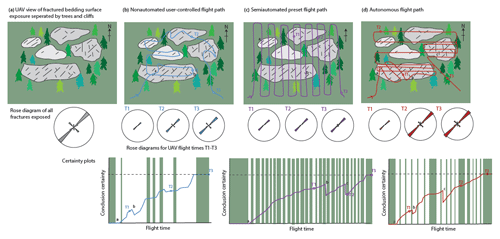
Figure 4Hypothetical UAV scenario in which the goal is to identify fractures on a bedding surface. Panel (a) shows a UAV view of fractured bedding surface (with exposure separated by trees and cliffs) and a rose diagram of all exposed fractures. Panel (b) shows a user-controlled flight path (in blue) over bedding surface, rose diagrams of cumulative fracture orientation data at flight times (T1, T2, T3), and an evolving conclusion certainty plot as the UAV collects data. On the conclusion certainty plot, green bars represent flight time over woodland rather than rock exposure, the letters a, b, and c represent when the UAV collects fracture data in a new orientation, and the dotted horizontal line represents the threshold of conclusion confidence. Panel (c) shows similar plots for a preset flight path (purple), and (d) shows plots for an autonomous flight path (red) on which the UAV attends and reacts to measurement data in real time.
Column C in Fig. 4 shows a UAV flight (purple) that is semiautomated to follow a preset path. For this hypothetical example, a reasonable preset flight path (and the one we assume most experts would take) would be to scan forward and backward across the area of interest, akin to a lawn mower. With this approach, the appearance is that no areas will be missed, the area is equally covered, and there is no risk of resampling, but the flight path will not be optimal to collect the data of interest – time will be wasted scanning areas that have little data value, and the linear N–S-oriented path will preferentially sample E–W-oriented fractures (biasing the dataset). On the certainty plot in column C this is visualized as long delays between the detection of unique fracture orientations (a, b, c), resulting in a step-like pattern – dramatic decreases in certainty when a new orientation is observed during navigation, followed by periods of slowly increasing certainty as information is observed that is consistent with previous features or irrelevant (green bars representing time over woodland). In this instance the user’s threshold of certainty is reached after a longer time period than in the user-driven scenario (column B), but the full range of fracture orientations is determined (see rose diagrams, column C).
The most efficient solution is for the UAV to move autonomously to areas with high data value by attending and reacting to measurement data in real time, e.g., skipping areas that are poorly exposed or homogenous, slowing, and flying multiple angles in areas that have a high frequency of important features (as defined by the user). This is visualized in column D (red flight path), where the UAV detects the first fracture orientation (a) and then recommends the user update the flight path to move orthogonal to the orientation to ensure it is representative and to optimize continued sampling. When a new orientation is detected (b) the UAV recommends updating the flight path again to optimize the collection of both orientations (i.e., horizontal flight path). In the updated horizontal flight path, the UAV moves efficiently over exposure that features already detected orientations (a, b), which leads to quicker detection of new orientations (c). The rose diagrams in column D show that by time T2 all three fracture sets have been identified. In fact, by time T3 fractures oriented NE–SW are being oversampled; the same fracture is crossed more than once by the UAV flight path, which is not an issue here as we are only interested in constraining the orientations of fractures, but it would need to be taken into consideration if the user wanted both orientation and relative intensity. The accompanying certainty plot in column D shows that this autonomous flight path results in a more efficient scientific decision-making process, i.e., a strong positive relationship between conclusion certainty and time, with a quick ascension to the threshold of confidence.
The goal of the reconnaissance UAV mapping was to detect all fracture orientations and determine the optimal location for sampling heterogeneous orientations. A UAV that possesses some representation of this goal can use multiple fracture orientation angles to rapidly calculate a flight path that will optimize continued sampling to confirm a fracture set or allow for more rapid detection of new orientations. This type of calculation is a task in which a computer is likely to excel relative to the human mind, since it will not be anchored to existing fracture models or the dominant fracture set. By offloading the task of navigation geometry to a UAV, the human expert frees up their cognitive resources for more important and difficult tasks, such as the real-time interpretation of surface features from UAV imagery. Were the goal of UAV reconnaissance to collect data on fracture length, orientation, and intensity, the programming of the UAV and the human interaction would be different. In this manner, we view the robot and human as a collaborative team, whereby better decision-making is a property of the coordination of both agents and a mutual understanding of the task goal as well as each other's strengths and weaknesses (Shipley and Tikoff, 2018). For example, critical to the success of our example would be for experts to understand how autonomous flight paths are being calculated and the conditions in which they will optimize data collection; an expert not privy to this information may mistrust well-calibrated path suggestions or over-trust path suggestions that are inconsistent with their goals. Also critical to the success of our example is that experts retain the ability to ignore autonomous path recommendations if their expertise leads them to favor an alternative path. One of the challenges in geosciences, and perhaps all sciences, is that AI systems focus only on the constrained problem and (unlike humans) are not open to the frisson of exploring other questions en route to the answer. Therefore, it is important that AI systems do not restrict users' autonomy to override recommendations, thereby barring the exploration of ideas through data collection that is too narrow or via inbuilt biases to automated data collection.
4.2.2 Case Study 2 – fault interpretations in 3-D seismic image data to minimize availability bias
In this case study, we consider how software interpretations of seismic image data, and the information derived from them, could be used to consider alternative models and minimize availability bias. Understanding of the geometries of subsurface geology is dominated by interpretations of seismic image data, and these interpretations serve a critical role in important tasks like resource exploration and geohazard assessment. 3-D seismic image volumes are analyzed as sequences of 2-D slices. Manual interpretation involves visually analyzing a 2-D image, identifying important patterns (e.g., faulted horizons, salt domes, gas chimneys), and labeling those patterns with distinct marks or colors, then keeping this information in mind while generating expectations about the contents of the next 2-D image. Given the magnitude and complexity of this task, there has been a strong and continued interest in developing semiautonomous and autonomous digital tools to make seismic interpretation more efficient and accurate (Araya-Polo et al., 2017; Di, 2018; Farrokhnia et al., 2018).
Here, we consider how 3-D information could be used with digital nudge technology to inform fault interpretations in a 3-D seismic image volume. Simple normal fault patterns show a bull's-eye pattern of greatest displacement in the center of an isolated fault, decreasing towards the fault tip (see image A, Fig. 5). Consider interpreting 2-D seismic image lines across the fault starting at in-line A (image A) and working towards in-line F: with each subsequent line the displacement of horizons across the fault should increase and then decrease, although this pattern will not be known until the interpretation is completed. Holding this information on displacements for individual faults between in-line interpretations in complicated seismic image data (e.g., with multiple faults per seismic section; image B, Fig. 5) is incredibly challenging even for the well-practiced expert. We imagine a digital nudge that alerts users to discrepancies in fault displacement patterns and prompts the consideration of alternative fault patterns, thereby relieving some of the cognitive burden of 3-D interpretation from the expert and guarding them against availability bias by encouraging the consideration of models beyond what is most readily accessible to the mind.
In our hypothetical example, a geoscientist analyzes a 3-D seismic volume, interpreting in a series of 2-D in-line images faults and horizon offsets. As subsequent in-lines (A–F) are interpreted, fault displacement patterns are co-visualized, so inconsistencies from normal fault displacement can be clearly seen. Fault 1 (image B) conforms to a simple fault displacement pattern (see Fault 1 displacement–distance plot). Fault 2 appears to conform to a similar pattern until in-line D when the interpreted displacement decreases; on interpretation of in-line E, the displacement on Fault 2 increases again, further highlighting the displacement anomaly on in-line D. Reduced displacement in itself does not highlight an issue, but consideration of the displacement–distance plot for Fault 1 suggests that if the interpreted displacement for Fault 2 is correct then the two faults are behaving differently. In our imagined digital tool, this discrepancy in displacement between nearby faults would be flagged for further consideration by the user, and potential alternative models could be highlighted. You can see the hypothetical conclusion certainty plots for the interpreter for the two faults (Fault 1: green line, Fault 2: pale blue line) during the interpretation process. Note the decrease in certainty of the interpreter for Fault 2, as they interpret in-lines D and E, in comparison to the increasing certainty for Fault 1 as consecutive interpreted in-lines conform to a simple normal fault displacement pattern. At in-line E the co-visualized displacement–distance plot nudges the interpreter to consider a new interpretation for Fault 2 at in-line D. Certainty in this new interpretation (displayed as a dark blue dashed line on the certainty plot) now increases as subsequent in-line interpretations conform to expected displacements.
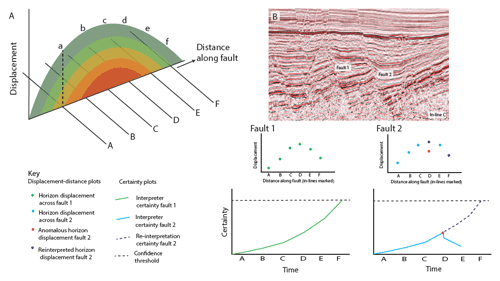
Figure 5Fault displacement–distance patterns used by a hypothetical digital tool to aid in the 3-D interpretation of normal faults. Panel (a) is a graphical representation of a horizon displacement with distance along a fault. In this simple pattern for an isolated normal fault, maximum displacement (red–green) is in the center with minimal displacement (green) at the faults tips. The fault is intersected by a series of hypothetical seismic lines (A–F), which correspond to the points on the displacement–distance plots. Panel (b) is a seismic image through faulted sedimentary rocks from the Inner Moray Firth, UK (note the complex fault pattern, including fault intersections). Two faults are highlighted in (b), Fault 1 and Fault 2, and their respective hypothetical displacement–distance plots are shown for a single horizon. On the Fault 2 plot, the red point at in-line D highlights an anomaly to the simple displacement–distance plot characteristics seen for Fault 1, and the dark blue point at in-line D represents the user manually inserting a new interpretation. Certainty plots corresponding to Fault 1 and Fault 2 are also displayed. Seismic image courtesy of the Virtual Seismic Atlas (http://www.seismicatlas.org, last access: 28 August 2019).
Our imagined digital tool builds on current autocorrelation tools in seismic interpretation software by aiding users in extrapolating information from a 2-D image to a 3-D representation. In this case study we show that by drawing on known fault displacement patterns, it should be possible to design tools that flag to users potential errors in fault displacement patterns along interpreted faults in 3-D seismic data. We describe information for a single horizon displacement, but multiple horizons could be plotted to highlight displacement changes with depth, such as syn-sedimentation. Our case study uses a simplified case, but fault displacement inconsistencies would likely be the result of more complex fault patterns and interactions. For example, it is possible to imagine a scenario in which both Fault 1 and Fault 2 showed significant decreases in displacement at in-line D, which might result in a decrease in user certainty for both faults and reinterpretation as linked faults. As highlighted in a 3-D seismic interpretation of faulted sedimentary rocks by Freeman et al. (2010), fault intersections are common and add challenges to understanding fault growth and displacement partitioning between faults; in their example, a full reinterpretation of the 3-D dataset was required after the evaluation of fault displacements on the original interpretations. Therefore, a digital tool (similar to the one we describe) that highlights possible fault intersections and relays during interpretation could cue researchers that more complex reasoning is needed so that simple dominant models – which as identified by Alcalde et al. (2017b) often show availability effects – are tempered by the consideration of more complex fault patterns and displacements. One concern, of course, is in limiting users’ consideration to known alternative concepts, which could constrain free and flexible thought while introducing software bias – this, and other digital nudging concerns, is discussed in more detail below.
Uncertainty is an inherent challenge in geological reasoning. Over 50 years of cognitive research demonstrates that, when faced with uncertainty, people rely on intuitive heuristics that can arise rapidly and with minimal effort. While efficient and effective in many situations, heuristics do lead to predictable biases in decision-making. We reviewed three biases that have been shown to influence geoscience experts: availability bias, framing bias, and anchoring bias. Bias can be overcome by engaging deliberative cognitive processing resources, which work as an “editor” to modify or override faulty heuristic responses. This occurs either because the decision maker employs a strategy that activates deliberative processes or because the environment is modified in such a way that the decision maker is “nudged” towards deliberative thinking. Because of the many barriers to success when debiasing is self-employed (e.g., not recognizing debiasing is needing, using the incorrect debiasing strategy, etc.), we strongly advocate adoption of the environment modification (i.e., choice architecture) approach. Further, we believe innovations in the use of information technology and AI in the geosciences can be leveraged to improve expert decision-making, i.e., digital nudging. We discussed two case studies illustrating different applications of intelligent systems for the debiasing of geoscientific decision-making. In each case study, debiased decision-making was an emergent property of the coordinated and integrated processing of human–AI collaborative teams.
Our discussion of digital nudging in the geosciences highlighted the positive attributes of this debiasing approach, chiefly that it provides relief from the cognitive constraints that lead to biased choice (and difficulty in effectively self-employing debiasing strategies), leaving the decision maker and their deliberative cognitive processing resources free to tackle other tasks. However, we would be remiss to not also caution against the potential pitfalls of the digital nudge. First, digital nudges can propagate existing biases (or introduce new ones) if they are poorly designed or trained using biased data. In a recent famous case, Amazon ceased testing an AI system used to evaluate job applicants after it was revealed to be gender biased, consistently penalizing applicants who attended women's colleges or whose resume contained the word “women's” in some other capacity (e.g., women's book club). Similar gender and racial biases have been demonstrated in judicial (Skeem and Lowenkamp, 2016) and medical (Challen et al., 2019) AI. To avoid unintended bias, choice architects must have a well-defined goal for the nudge and a clear understanding of the decision process, including the identification of the critical actions involved in following through with the decision, the identification of constraints to the achievement of each critical action, a determination of the amount of attention devoted to each critical action and the decision process as a whole, a determination of the amount of decision-relevant information gathered, and the identification of the main heuristics and biases influencing the decision process (see Ly et al., 2013, Appendix 2 for a suggested list of questions choice architects should ask themselves when evaluating a decision process). Choice architects should also submit nudge designs to careful testing, paying special attention to factors unrelated to the nudge that might influence the results.
A second pitfall is that there is a risk of limited uptake of digital nudges if they are perceived by the user as untrustworthy. To be effective, a nudge must address the particular bias an individual is experiencing – but people can differ in the biases they bring to a choice environment. Nudges that are viewed as inappropriate or misleading by the user may be ignored and mistrusted. Therefore, choice architects should be thoughtful in their selection of environments (i.e., employing nudges when there is consistency in the type of bias observed in a specific environment) and seek to design nudges that are effective against a range of biases. Special attention should also be paid to the relative “politeness” of nudges (Whitworth, 2005); i.e., does the nudge respect and not preempt user choice, and does the nudge avoid pestering or interrupting the user unnecessarily? Nudges that make correct suggestions, but do so in an impolite or obtrusive manner, will still be viewed as untrustworthy – we call this the “Mr. Clippy problem” in reference to the famously derided Microsoft Office assistant that took the form of an animated paper clip. Early users of Office will recall that Mr. Clippy popped up uninvited, preemptively taking control of the cursor and demanding to help (his most famous line, “It looks like you're writing a letter…” appeared every time the user typed “Dear…”). Worse yet, Mr. Clippy ignored continuous rejection: hide him and he would simply reappear, ignore him and he would repeat the unsolicited advice (again and again). To avoid the Mr. Clippy problem, choice architects should consider how best to implement nudges within existing user workflows to minimize distraction and maintain user autonomy.
One way choice architects may increase understanding and trust of digital nudges is through being transparent in the “reasoning” behind why a nudge is prompted, whereby reasoning refers to some interpretable translation of the underlying AI algorithm and decision inputs. This type of “explainable AI” (Miller, 2019) is critical to our vision of collaborative and coordinated decision-making in human–AI teams. Just as successful human teams are aware of the values, needs, intentions, actions, and capabilities of all team members, so should human–AI teams be reciprocally aware – this occurs over time: through interaction, shared experience, and feedback, team members can jointly and iteratively refine their beliefs and expectations about each other's behavior. While there are challenges to achieving mutually aware human–AI teams (for review, see de Graaf and Malle, 2017), they are, in our opinion, far outweighed by the potential value of calibrating user trust in technology.
Finally, there are concerns about the ethics and morality of nudging (digital or otherwise). Some believe that nudging is morally reprehensible because it patronizes the decision maker by assuming they are not capable of making the best choice for themselves (Gigerenzer, 2015). However, as discussed in this paper, there is strong scientific evidence that human decision makers are both (a) susceptible to cognitive bias across a range of choices and (b) struggle to successfully employ debiasing techniques to improve their judgment. We believe that if decision makers are aware of their vulnerabilities and shown the potential value of nudging (through education or experience with polite and explainable nudges), they may be less likely to perceive nudges as condescending or infantilizing. Another oft-cited ethical concern is that nudged individuals will become used to being guided away from negative consequences, resulting in a diminished ability to make good choices and assume responsibility for those choices (Bovens, 2009). Related to this, there is concern that the more used to nudging we become, the less we will be bothered by the introduction of more controlling or coercive techniques (Rizzo and Whitman, 2009). Yet, how decision makers respond to nudges in the long term is an open empirical question. One possibility is that nudges have only short-term effects, and as time goes on, the level of nudging required to retain this effect increases because decision makers habituate to the nudge. If this is the case, then decision makers would retain their original preference structures, meaning they would make different choices without the aid from the nudge, placing them at risk of taking less personal responsibility for their choices because they assume other members of society will nudge them away from anything that is bad. The alternative is that repeated nudges induce actual preference change in the long term; this could occur because the decision maker recognizes the hitherto unknown benefits of the nudged choice, because their sense of identity becomes linked to the nudged choice, or because the nudged choice becomes conditioned (in the Pavlovian style). Ultimately, different people will likely adapt preferences in response to different nudges in different ways, and future research should consider both the short-term and long-term effects.
Returning to the opening quote and question posed by Gilbert, “…whether it is possible by training to improve the guessing faculty, and if so, how it is to be done”, the answer is unequivocally yes, and we believe that digital nudging offers the best opportunity to overcome the cognitive constraints that result in biased decisions. As described at the outset of this paper, we hope our review of the cognitive literature on bias and debiasing will help readers to understand the constraints to human decision-making and better equip them with strategies for improving choice. We also hope this paper will stimulate future research on the important topic of debiasing geologic decision-making, particularly in the context of evolving advancements in information technology and AI.
No data sets were used in this article.
CGW wrote Sects. 1 through 3 and contributed to writing Sect. 4. CEB and TFS contributed to writing Sect. 4 and assisted in editing the entire paper.
The authors declare that they have no conflict of interest.
This article is part of the special issue “Understanding the unknowns: the impact of uncertainty in the geosciences”. It is not associated with a conference.
Special thanks to the reviewers of this paper for their insightful comments.
This research has been supported by the National Science Foundation (Science of Learning Collaborative Network (grant no. 1640800), National Robotics Initiative (grant no. 1734365), and Future of Work at the Human Technology Frontier (grant no. 1839705)) and the Royal Society of Edinburgh (Research sabbatical grant).
This paper was edited by Lucia Perez-Diaz and reviewed by Florian Wellmann, Simon Oldfield, and one anonymous referee.
Alcalde, J., Bond, C. E., and Randle, C. H.: Framing bias: The effect of figure presentation on seismic interpretation, Interpretation, 5, 591–605, https://doi.org/10.1190/INT-2017-0083.1, 2017a. a
Alcalde, J., Bond, C. E., Johnson, G., Butler, R. W. H., Cooper, M. A., and Ellis, J. F.: The importance of structural model availability on seismic interpretation, J. Struct. Geol., 97, 161–171, https://doi.org/10.1016/j.jsg.2017.03.003, 2017b. a, b, c, d
Andrews, B. J., Roberts, J. J., Shipton, Z. K., Bigi, S., Tartarello, M. C., and Johnson, G.: How do we see fractures? Quantifying subjective bias in fracture data collection, Solid Earth, 10, 487–516, https://doi.org/10.5194/se-10-487-2019, 2019. a
Araya-Polo, M., Dahlke, T., Frogner, C., Zhang, C., Poggio, T., and Hohl, D.: Automated fault detection without seismic processing, The Leading Edge, 36, 208–214, https://doi.org/10.1190/tle36030208.1, 2017. a
Argent, R. M.: An overview of model integration for environmental applications – components, frameworks and semantics, Environ. Modell. Softw., 19, 219–234, https://doi.org/10.1016/S1364-8152(03)00150-6, 2004. a, b
Argent, R. M. and Houghton, B.: Land and water resources model integration: Software engineering and beyond, Adv. Environ. Res., 5, 351–359, https://doi.org/10.1016/S1093-0191(01)00086-7, 2001. a, b
Baddeley, M. C., Curtis, A., and Wood, R.: An introduction to prior information derived from probabilistic judgements: Elicitation of knowledge, cognitive bias and herding, in: Geological Prior Information: Informing Science and Engineering, edited by: Curtis, A. and Wood, R., The Geological Society of London, London, UK, 15–27, https://doi.org/10.1144/GSL.SP.2004.239.01.02, 2004. a
Baddeley, M.: Herding, social influences and behavioural bias in scientific research: Simple awareness of the hidden pressures and beliefs that influence our thinking can help to preserve objectivity, EMBO Rep., 16, 902–905, https://doi.org/10.15252/embr.201540637, 2015. a
Barclay, E. J., Renshaw, C. E., Taylor, H. A., and Bilge, A. R.: Improving decision making skill using an online volcanic crisis simulation: Impact of data presentation format, Journal of Geoscience Education, 59, 85–92, https://doi.org/10.5408/1.3543933, 2011. a, b, c, d
Bárdossy, G. and Fodor, J.: Traditional and new ways to handle uncertainty in geology, Natural Resources Research, 10, 179–187, https://doi.org/10.1023/A:1012513107364, 2001. a
Beaulac, G. and Kenyon, T.: Critical thinking education and debiasing, Informal Log., 34, 341–363, https://doi.org/10.22329/il.v34i4.4203, 2014.
Böckenholt, U.: The cognitive-miser response model: Testing for intuitive and deliberate reasoning, Psychometrika, 77, 388–399, https://doi.org/10.1007/s11336-012-9251-y, 2012. a
Bond, C. E.: Uncertainty in structural interpretation: Lessons to be learnt, J. Struct. Geol., 74, 185–200, https://doi.org/10.1016/j.jsg.2015.03.003, 2015. a, b
Bond, C. E., Gibbs, A. D., Shipton, Z. K., and Jones, S.: What do you think this is? “Conceptual uncertainty” in geoscience interpretation, Geological Society of America Today, 17, 4–10, https://doi.org/10.1130/GSAT01711A.1, 2007. a, b, c, d
Bond, C. E., Shipton, Z. K., Gibbs, A. D., and Jones, S.: Structural models: Optimizing risk analysis by understanding conceptual uncertainty, First Break, 26, 65–71, https://doi.org/10.3997/1365-2397.2008006, 2008. a
Bovens, L.: The ethics of nudge, in: Preference change, edited by: Hansson, M. J. and Grüne-Yanoff, T., Springer, Berlin, Germany, 207–219, https://doi.org/10.1007/978-90-481-2593-7_10, 2009. a
Camerer, C. F. and Hogarth, R. M.: The effects of financial incentives in experiments: A review and capital-labor-production framework, J. Risk Uncertainty, 19, 7–42, https://doi.org/10.1023/A:1007850605129, 1999. a
Challen, R., Denny, J., Pitt, M., Gompels, L., Edwards, T., and Tsaneva-Atanasova, K.: Artificial intelligence, bias and clinical safety, BMJ Quality and Safety, 28, 231–237, https://doi.org/10.1136/bmjqs-2018-008370, 2019. a
Chamberlin, T. C.: The method of multiple working hypotheses, Science, 15, 92–96, https://doi.org/10.1126/science.148.3671.754, 1890. a
Chen, C. and Zhao, B.: Review of relationship between indoor and outdoor particles: I∕O ratio, infiltration factor and penetration factor, Atmos. Environ., 45, 275–288, https://doi.org/10.1016/j.atmosenv.2010.09.048, 2011. a
Cooke, N. M. and McDonald, J. E.: A formal methodology for acquiring and representing expert knowledge, P. IEEE, 74, 1422–1430, https://doi.org/10.1109/PROC.1986.13643, 1986. a
Czaika, E. and Selin, N. E.: Model use in sustainability policy making: An experimental study, Environ. Modell. Softw., 98, 54–62, https://doi.org/10.1016/j.envsoft.2017.09.001, 2017. a
Dawes, R. M. and Corrigan, B.: Linear models in decision making, Psychol. Bull., 81, 95–106, https://doi.org/10.1037/h0037613, 1974. a
Dawes, R. M., Faust, D., and Meehl, P. E.: Clinical versus actuarial Judgment, Science, 24, 1668–1674, https://doi.org/10.1126/science.2648573, 1989. a
de Graaf, M. M. and Malle, B. F.: How people explain action (and autonomous intelligent systems should too), in: AAAI Fall Symposium Series, Artificial Intelligence for Human-Robot Interaction, 9–11 November 2017, Arlington, Virgina, 19–26, 2017. a
Di, H.: Developing a seismic pattern interpretation network (SpiNet) for automated seismic interpretation, arXiv preprint arXiv:1810.08517, 2018. a
Downs, J. S., Loewenstein, G., and Wisdom, J.: Eating by the numbers, New York Times, A31, available at: https://www.nytimes.com/2009/11/13/opinion/13lowenstein.html (last access: 28 August 2019), 2009. a
Edwards, D., Morris, J., and Tabbush, P.: Stakeholder participation in the development and use of sustainability impact assessment tools for European land use policies, in: EASY-ECO Conference, Sustainable Development Evaluations in Europe, Brussels, Belgium, 17–19 November, 2010. a
Elliot, L. P. and Brook, B. W.: Revisiting Chamberlin: Multiple working hypotheses for the 21st century, BioScience, 57, 608–614, https://doi.org/10.1641/B570708, 2007. a
Englich, B., Mussweiler, T., and Strack, F.: Playing dice with criminal sentences: The influence of irrelevant anchors on experts judicial decision making, Pers. Soc. Psychol. B., 32, 188–200, https://doi.org/10.1177/0146167205282152, 2006. a
Epley, N. and Gilovich, T.: The anchoring-and-adjustment heuristic: Why the adjustments are insufficient, Psychol. Sci., 17, 311–318, https://doi.org/10.1111/j.1467-9280.2006.01704.x, 2006. a, b
Evans, J. S. B. T. and Stanovich, K. E.: Dual-process theories of higher cognition: Advancing the debate, Perspect. Psychol. Sci., 8, 223–241, https://doi.org/10.1177/1745691612460685, 2013. a, b, c
Fagley, N. S. and Miller, P. M.: Framing effects and arenas of choice: Your money or your life?, Organ. Behav. Hum. Dec., 71, 355–373, https://doi.org/10.1006/obhd.1997.2725, 1997. a
Farrokhnia, F., Kahoo, A. R., and Soleimani, M.: Automatic salt dome detection in seismic data by combination of attribute analysis on CRS images and IGU map delineation, J. Appl. Geophys., 159, 395–407, https://doi.org/10.1016/j.jappgeo.2018.09.018, 2018. a
Fogg, B. J.: Persuasive technology: Using computers to change what we think and do, Morgan Kaufmann Publishers, San Francisco, CA, 2003. a
Fischhoff, B.: Debiasing, in: Judgment Under Uncertainty: Heuristics and Biases, edited by: Kahneman, D., Slovic, P., and Tversky, A., Cambridge University Press, Cambridge, UK, 422–444, 1982. a, b
Fong, G. T., Krantz, D. H., and Nisbett, R. E.: The effects of statistical training on thinking about everyday problems, Cognitive Psychol., 18, 253–292, https://doi.org/10.1016/0010-0285(86)90001-0, 1986.
Frederick, S.: Cognitive reflection and decision making, J. Econ. Perspect., 19, 25–42, https://doi.org/10.1257/089533005775196732, 2005. a
Freeman, B., Boult, P. J., Yielding, G., and Menpes, S.: Using empirical geological rules to reduce structural uncertainty in seismic interpretation of faults, J. Struct. Geol., 32, 1668–1676, https://doi.org/10.1016/j.jsg.2009.11.001, 2010. a
Frodeman, R.: Geological reasoning: Geology as an interpretive and historical science, Geol. Soc. Am. Bull., 107, 960–968, https://doi.org/10.1130/0016-7606(1995)107<0960:GRGAAI>2.3.CO;2, 1995. a
Garcia-Retamero, R. and Dhami, M. K.: On avoiding framing effects in experienced decision makers, Q. J. Exp. Psychol., 66, 829–842, https://doi.org/10.1080/17470218.2012.727836, 2013. a
Gigerenzer, G.: On the supposed evidence for libertarian paternalism, Review of Philosophy and Psychology, 6, 361–383, https://doi.org/10.1007/s13164-015-0248-1, 2015. a
Gil, Y., David, C. H., Demir, I., Essawy, B. T., Fulweiler, R. W., Goodall, J. L., Karlstrom, L., Lee, H., Mills, H. J., Oh, J., Pierce, S. A., Pope, A., Tzeng, M. W., Villamizar, S. R., and Yu, Z.: Toward the geoscience paper of the future: best practices for documenting and sharing research from data to software to provenance, Earth Space Sci., 3, 388–415, https://doi.org/10.1002/2015EA000136, 2016. a
Gil, Y., Pierce, S. A., Babaie, H., Banerjee, A., Borne, K., Bust, G., Cheatham, M., Ebert-Uphoff, I., Gomes, C., Hill, M., Horel, J., Hsu, L., Kinter, J., Knoblock, C., Krum, D., Kumar, V., Lermusiaux, P., Liu, C., North, C., Pankratius, V., Peters, S., Plale, B., Pope, A., Ravela, S., Restrepo, J., Ridley, A., Samet, H., and Shekhar, S.: Intelligent systems for geosciences: an essential research agenda, Commun ACM, 62, 76–84, https://doi.org/10.1145/3192335, 2018. a
Gilbert, G. K.: The inculcation of scientific method by example, with an illustration drawn from the Quaternary geology of Utah, Am. J. Sci., 31, 284–299, https://doi.org/10.2475/ajs.s3-31.184.284, 1886. a
Gilovich, T., Griffin, D. W., and Kahneman, D. (Eds.): Heuristics and Biases: The Psychology of Intuitive Judgment, Cambridge University Press, Cambridge, UK, 2002. a
Hemming, V., Walshe, T. V., Hanea, A. M., Fidler, F., and Burgman, M. A.: Eliciting improved quantitative judgements using the IDEA protocol: A case study in natural resource management, PloS One, 13, e0198468, https://doi.org/10.1371/journal.pone.0198468, 2018. a
Henderson, C., Mestre, J. P., and Slakey, L. L.: Cognitive Science Research Can Improve Undergraduate STEM Instruction: What Are the Barriers?, Policy Insights from the Behavioral and Brain Sciences, 2, 51–60, https://doi.org/10.1177/2372732215601115, 2015.
Holden, M. H. and Ellner, S. P.: Human judgment vs. quantitative models for the management of ecological resources, Ecol. Appl., 26, 1553–1565, https://doi.org/10.1890/15-1295, 2016. a
Johnson, E. J. and Goldstein, D.: Do defaults save lives?, Science, 302, 1338–1339, https://doi.org/10.1126/science.1091721, 2003. a
Kahneman, D.: Thinking, Fast and Slow, Farrar, Straus, and Giroux, New York, 2011. a
Kahneman, D. and Frederick, S.: A model of heuristic judgment, in: The Cambridge Handbook of Thinking and Reasoning, edited by: Holyoak, K. J. and Morrison, R. G., Cambridge University Press, Cambridge, UK, 267–293, 2002. a
Kahneman, D. and Frederick, S.: Frames and brains: Elicitation and control of response tendencies, Trends Cogn. Sci., 11, 45–46, https://doi.org/10.1016/j.tics.2006.11.007, 2007. a
Karpatne, A., Ebert-Uphoff, I., Ravela, S., Babaie, H. A., and Kumar, V.: Machine learning for the geosciences: Challenges and opportunities, IEEE T. Knowl Data En., 31, 1544–1554, https://doi.org/10.1109/TKDE.2018.2861006, 2018. a
Kempler, L. and Gross, S.: Teaching Quantitative Thinking in Geoscience with MATLAB, Geophys. Res. Abstr., EGU2018-19379, EGU General Assembly 2018, Vienna, Austria, 2018.
Koparan, C., Koc, A., Privette, C., and Sawyer, C.: In situ water quality measurements using an unmanned aerial vehicle (UAV) system, Water, 10, 264–278, https://doi.org/10.3390/w10030264, 2018. a
Koriat, A., Lichtenstein, S., and Fischhoff, B.: Reasons for confidence, J. Exp. Psychol.-Hum. L., 6, 107, https://doi.org/10.1037/0278-7393.6.2.107, 1980. a
Larrick, R. P.: Debiasing, in: Blackwell Handbook of Judgment and Decision Making, edited by: Koehler, D. J. and Harvey, N., Blackwell Handbook of Judgment and Decision Making, Blackwell Publishing Ltd, Hoboken, NJ, 316–337, 2004. a, b, c
Larrick, R. P., Morgan, J., and Nisbett, R. E.: Teaching the use of cost-benefit reasoning in everyday life, Psychol. Sci., 1, 362–370, https://doi.org/10.1111/j.1467-9280.1990.tb00243.x, 1990.
Larrick, R. P., Nisbett, R. E., and Morgan, J.: Who uses the normative rules of choice, in: Rules for Reasoning, edited by: Nisbett, R. E., Lawrence Erlbaum Associates, Inc., Hillsdale, NJ, 277–294, 1993.
Lerner, J. S. and Tetlock, P.: Accounting for the effects of accountability, Psychol. Bull., 125, 255–275, https://doi.org/10.1037/0033-2909.125.2.255, 1999. a
Levin, I. P., Schneider, S. L., and Gaeth, G. J.: All frames are not created equal: A typology and critical analysis of framing effects, Organ. Behav. Hum. Dec., 76, 149–188, https://doi.org/10.1006/obhd.1998.2804, 1998. a
Lindberg, D. C.: Galileo, the church, and the cosmos, in: When Science and Christianity Meet, edited by: Lindberg, D. C. and Numbers, R. L., University of Chicago Press, Chicago, IL, 33–60, 2003. a
Lorenz, J., Rauhut, H., Schweitzer, F., and Helbing, D.: How social influence can undermine the wisdom of crowd effect, P. Natl. Acad. Sci. USA, 108, 9020–9025, https://doi.org/10.1073/pnas.1008636108, 2011. a
Ly, K., Mažar, N., Zhao, M., and Soman, D.: A practitioner's guide to nudging, Rotman School of Management Working Paper, 2609347, https://doi.org/10.2139/ssrn.2609347, 2013. a
Madrian, B. C. and Shea, D. F.: The power of suggestion: Inertia in 401(k) participation and savings behavior, Q. J. Econ., 116, 1149–1187, https://doi.org/10.3386/w7682, 2001. a
Manduca, C. A., Baer, E., Hancock, G., Macdonald, R. H., Patterson, S., Savina, M., and Wenner, J.: Making undergraduate geoscience quantitative, EOS T. Am. Geophys. Un., 89, 149–150, https://doi.org/10.1029/2008EO160001, 2008.
Macrae, E. J., Bond, C. E., Shipton, Z. K., and Lunn, R. J.: Increasing the quality of seismic interpretation, Interpretation, 4, T395–T402, https://doi.org/10.1190/INT-2015-0218.1, 2016. a, b, c, d, e, f, g
McIntosh, B., Voinov, A., and Giupponi, C.: Bridging the gaps between design and use: developing appropriate tools for environmental management and policy, in: Environmental Modelling, Software and Decision Support, edited by: Jakeman, A. J., Voinov, A. A., Rizzoli, A. E., and Chen, S. H., Elsevier, Amsterdam, the Netherlands, 33–48, 2008. a
McIntosh, B. S., Jeffrey, P., Lemon, M., and Winder, N.: On the design of computer-based models for integrated environmental science, Environ. Manage., 35, 741–752, https://doi.org/10.1007/s00267-004-0172-0, 2005. a
McNeil, B. J., Pauker, S. G., Sox, H. C., and Tversky, A.: On the elicitation of preferences for alternative therapies, New Engl. J. Med., 306, 1259–1262, https://doi.org/10.1056/NEJM198205273062103, 1982. a
Milkman, K. L., Chugh, D., and Bazerman, M. H.: How can decision making be improved?, Perspect. Psychol. Sci., 4, 379–383, https://doi.org/10.1111/j.1745-6924.2009.01142.x, 2009. a, b
Miller, T.: Explanation in artificial intelligence: Insights from the social sciences, Artif. Intell., 267, 1–38, https://doi.org/10.1016/j.artint.2018.07.007, 2019. a
Mirsch, T., Lehrer, C., and Jung, R.: Digital nudging: altering user behavior in digital environments, in: Proceedings der 13. Internationalen Tagung Wirtschaftsinformatik, St. Gallen, Switzerland, 12–15 February 2017, 634–648, 2017. a
Mussweiler, T., Strack, F., and Pfeiffer, T.: Overcoming the inevitable anchoring effect: Considering the opposite compensates for selective accessibility, Pers. Soc. Psychol. B, 26, 1142–1150, https://doi.org/10.1177/01461672002611010, 2000. a
Ore, J. P., Elbaum, S., Burgin, A., and Detweiler, C.: Autonomous aerial water sampling, J. Field Robot., 32, 1095–1113, https://doi.org/10.1002/rob.21591, 2015. a
Oxley, T., McIntosh, B. S., Winder, N., Mulligan, M., and Engelen, G.: Integrated modelling and decision-support tools: a Mediterranean example, Environ. Modell. Softw., 19, 999–1010, https://doi.org/10.1016/j.envsoft.2003.11.003, 2004. a
Phillips, L. D.: Group elicitation of probability distributions: Are many heads better than one?, in: Decision Science and Technology, edited by: Shanteau, J., Mellers, B. A., and Schum, D. A., Springer, Boston, Massachusetts, 313–330, 1999. a, b
Polson, D. and Curtis, A.: Dynamics of uncertainty in geological interpretation, J. Geol. Soc. London, 167, 5–10, https://doi.org/10.1144/0016-76492009-055, 2010. a, b, c, d, e, f, g, h
Qian, F., Jerolmack, D., Lancaster, N., Nikolich, G., Reverdy, P., Roberts, S., Shipley, T. F., Van Pelt, R. S., Zobeck, T. M., and Koditschek, D. E.: Ground Robotic Measurement of Aeolian Processes, Aeolian Res., 27, 1–11, https://doi.org/10.1016/j.aeolia.2017.04.004, 2017. a
Randle, C. H., Bond, C. E., Lark, R. M., and Monaghan, A. A.: Uncertainty in geological interpretations: Effectiveness of expert elicitations, Geosphere, 15, 108–118, https://doi.org/10.1130/GES01586.1, 2019. a, b
Rankey, E. C. and Mitchell, J. C.: That's why it's called interpretation: Impact of horizon uncertainty on seismic attribute analysis, The Leading Edge, 22, 820–828, https://doi.org/10.1190/1.1614152, 2003. a, b
Rizzo, M. J. and Whitman, D. G.: Little brother is watching you: New paternalism on the slippery slopes, Arizona Law Review, 51, 685–739, https://doi.org/10.2139/ssrn.1119325, 2009. a
Rizzoli, A. E. and Young, W. J.: Delivering environmental decision support systems: software tools and techniques, Environ. Modell. Softw., 12, 237–249, https://doi.org/10.1016/S1364-8152(97)00016-9, 1997. a, b
Rowbotham, P., Kane, P., and Bentley, M.: Bias in geophysical interpretation: The case for multiple deterministic scenarios, The Leading Edge, 29, 590–595, https://doi.org/10.1190/1.3422459, 2010. a, b
Sanna, L. J. and Schwarz, N.: Metacognitive experiences and human judgment: The case of hindsight bias and its debiasing, Curr. Dir. Psychol. Sci., 15, 172–176, https://doi.org/10.1111/j.1467-8721.2006.00430.x, 2006. a
Shanteau, J., Weiss, D. J., Thomas, R. P., and Pounds, J. C.: Performance-based assessment of expertise: How to decide if someone is an expert or not, Eur. J. Oper. Res., 136, 253–263, https://doi.org/10.1016/S0377-2217(01)00113-8, 2002. a
Shipley, T. F. and Tikoff, B.: Collaboration, cyberinfrastructure, and cognitive science: The role of databases and dataguides in 21st century Structural Geology, J. Struct. Geol., 125, 48–54, https://doi.org/10.1016/j.jsg.2018.05.007, 2018. a, b
Skeem, J. L. and Lowenkamp, C. T.: Risk, race, and recidivism: Predictive bias and disparate impact, Criminology, 54, 680–712, https://doi.org/10.2139/ssrn.2687339, 2016. a
Soll, J. B., Milkman, K. L., and Payne, J. W.: A user's guide to debiasing, in: Wiley Blackwell Handbook of Judgment and Decision Making, 2nd Edn., edited by: Keren, G. and Wu, G., Blackwell Publishing Ltd, Hoboken, NJ, 506–951, 2016. a, b
Spino, R.: Big data and artificial intelligence analytics in geosciences: Promises and potential, Geological Society of America Today, 29, 42–43, https://doi.org/10.1130/GSATG372GW.1, 2019. a
Stanovich, K. E.: The thinking that IQ tests miss, Scientific American Mind, 20, 34–39, 2009. a
Stanovich, K. E. and West, R. F.: Individual differences in reasoning: Implications for the rationality debate?, Behav. Brain Sci., 23, 645–726, https://doi.org/10.1017/CBO9780511808098.026, 2000. a
Taylor, H. A., Renshaw, C. E., and Jensen, M. D.: Effects of computer-based role-playing on decision making skills, J. Educ. Comput. Res., 17, 147–164, https://doi.org/10.2190/Y1CX-XU4G-KDFK-JBFJ, 1997. a, b, c, d, e
Thaler, R. H. and Sunstein, C. R.: Nudge: Improving decisions about health, wealth, and happiness, Yale University Press, New Haven, CT, 2008. a, b
Thiele, S. T., Grose, L., Samsu, A., Micklethwaite, S., Vollgger, S. A., and Cruden, A. R.: Rapid, semi-automatic fracture and contact mapping for point clouds, images and geophysical data, Solid Earth, 8, 1241–1253, https://doi.org/10.5194/se-8-1241-2017, 2017. a
Tversky, A. and Kahneman, D.: The framing of decisions and the psychology of choice, Science, 211, 453–458, https://doi.org/10.1126/science.7455683, 1981. a, b
Vasuki, Y., Holden, E. J., Kovesi, P., and Micklethwaite, S.: Semi-automatic mapping of geological Structures using UAV-based photogrammetric data: An image analysis approach, Comput. Geosci., 69, 22–32, https://doi.org/10.1016/j.cageo.2014.04.012, 2014. a
Vasuki, Y., Holden, E. J., Kovesi, P., and Micklethwaite, S.: An interactive image segmentation method for lithological boundary detection: A rapid mapping tool for geologists, Comput. Geosci., 100, 27–40, https://doi.org/10.1016/j.cageo.2016.12.001, 2017. a
Vine, F. J.: The continental drift debate, Nature, 266, 19–22, https://doi.org/10.1038/266019a0, 1977. a
Watkins, H., Bond, C. E., Healy, D., and Butler, R. W.: Appraisal of fracture sampling methods and a new workflow to characterise heterogeneous fracture networks at outcrop, J. Struct. Geol., 72, 67–82, https://doi.org/10.1016/j.jsg.2015.02.001, 2015. a
Weinmann, M., Schneider, C., and vom Brocke, J.: Digital nudging, Bus. Inf. Syst. Eng., 58, 433–436, https://doi.org/10.1007/s12599-016-0453-1, 2016. a
Whitworth, B.: Polite computing, Behav. Inform. Technol., 24, 353–363, https://doi.org/10.1080/01449290512331333700, 2005. a
Wood, R. and Curtis, A.: Geological prior information and its applications to geoscientific problems, Geol. Soc. Spec Publ., 239, 1–14, https://doi.org/10.1144/GSL.SP.2004.239.01.01, 2004. a, b
Zhang, Y., Wang, G., Li, M., and Han, S.: Automated Classification Analysis of Geological Structures Based on Images Data and Deep Learning Model, Appl. Sci., 8, 2493, https://doi.org/10.3390/app8122493, 2018. a
The reporter–editor analogy, to our knowledge, was first introduced by Daniel Kahneman in 2013 during an interview with Morgan Housel for The Motley Fool, a multimedia financial advisement company.
This example represents only one manifestation of framing bias, referred to as “risky choice framing”. For a complete typology of framing effects see Levin et al., 1998.